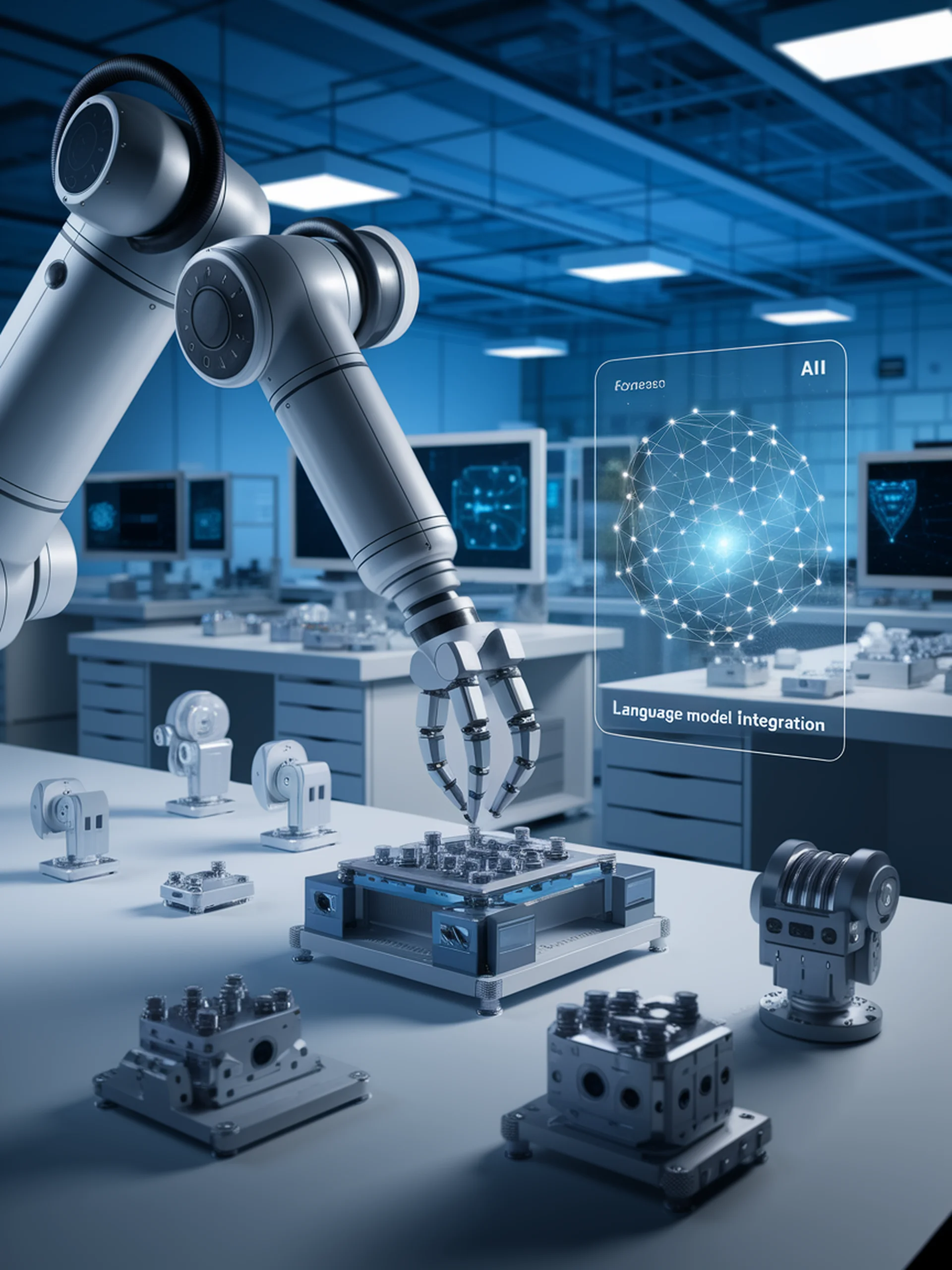
Enhancing Robotic Intelligence with LLMs
A hierarchical reinforcement learning approach for complex tasks
This research introduces LDSC, a framework that combines Large Language Models with hierarchical reinforcement learning to improve robots' ability to learn complex tasks.
- Leverages LLMs for intelligent subgoal selection and option reuse
- Significantly enhances sample efficiency for reinforcement learning
- Improves generalization and multi-task adaptability
- Demonstrates promising results for complex robotic applications
This breakthrough matters for engineering as it addresses fundamental challenges in robotic learning: exploration inefficiency and high computational demands. The approach enables robots to learn complex behaviors more efficiently by using language models to guide the learning process.
Option Discovery Using LLM-guided Semantic Hierarchical Reinforcement Learning