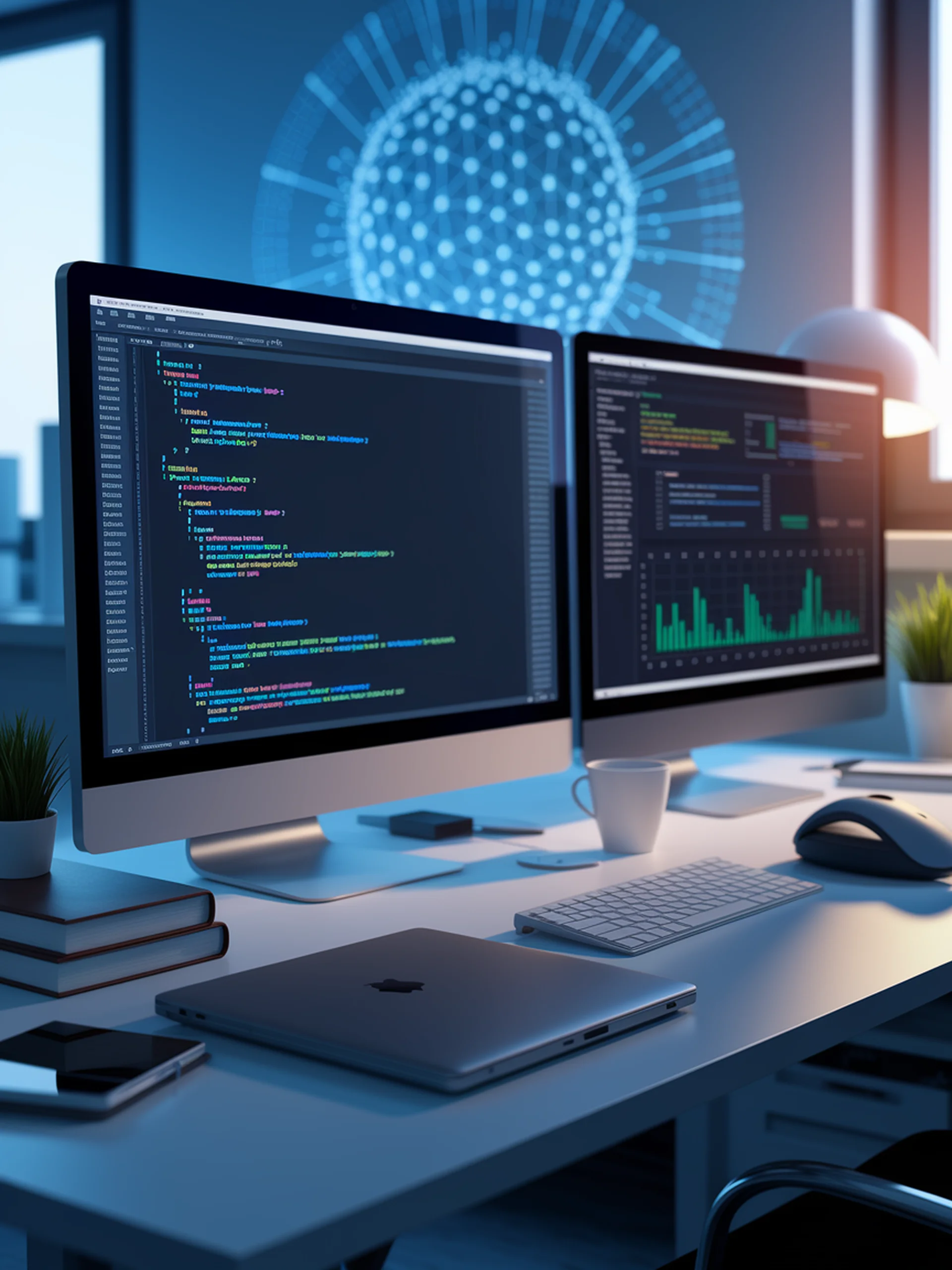
Prompt Engineering vs. Fine-Tuning for Code
Comparing LLM approaches for code-related tasks
This research compares the effectiveness of prompt engineering versus fine-tuning approaches for adapting large language models to perform code-related tasks.
- Evaluates different strategies for querying LLMs like ChatGPT
- Assesses the performance benefits of fine-tuning pre-trained models like CodeBERT
- Provides empirical evidence to guide engineering decisions between these two approaches
- Offers practical insights for implementing LLMs in software development workflows
For engineering teams, this research offers critical guidance on selecting the most effective and resource-efficient approach when implementing AI solutions for code generation, summarization, and translation tasks.
Prompt Engineering or Fine-Tuning: An Empirical Assessment of LLMs for Code