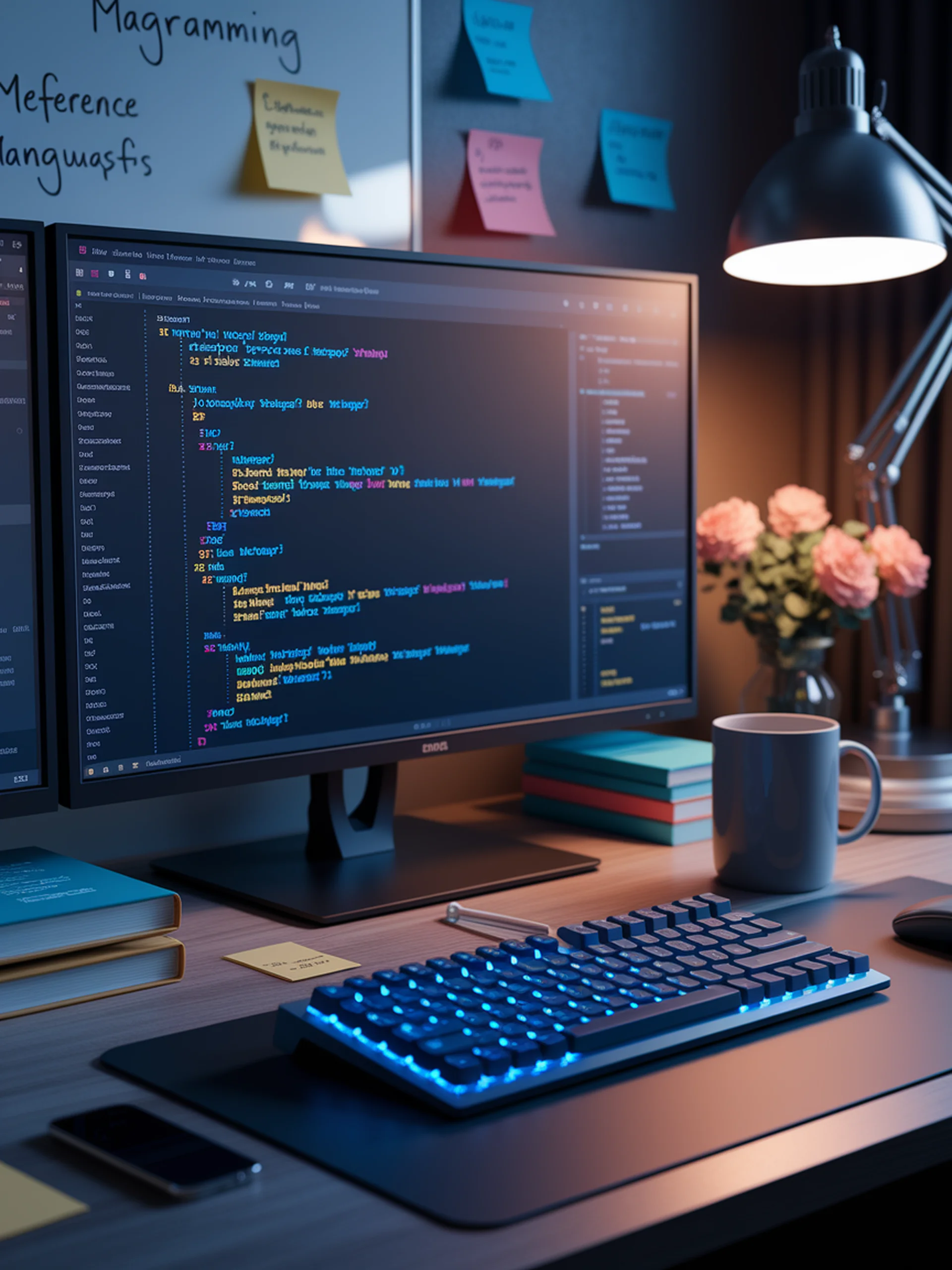
Real-World Code Translation to Rust
First major study evaluating LLM performance on practical language migration
This study benchmarks five leading LLMs (GPT-4, Claude 3, Claude 2.1, Gemini Pro, and Mixtral) on their ability to translate real-world code to Rust, revealing significant performance gaps.
- GPT-4 and Claude 3 achieved highest accuracy, but all models struggle with Rust's ownership concepts
- Models perform best on simple, self-contained functions but struggle with complex dependencies
- Success rates vary dramatically by source language (Python: 56%, Java: 51%, C++: 46%, C: 41%)
- Current models still require significant human intervention for production use
This research is critical for engineering teams considering automated migration tools for legacy codebases, highlighting both the potential and limitations of using LLMs for code translation to memory-safe languages like Rust.
Towards Translating Real-World Code with LLMs: A Study of Translating to Rust