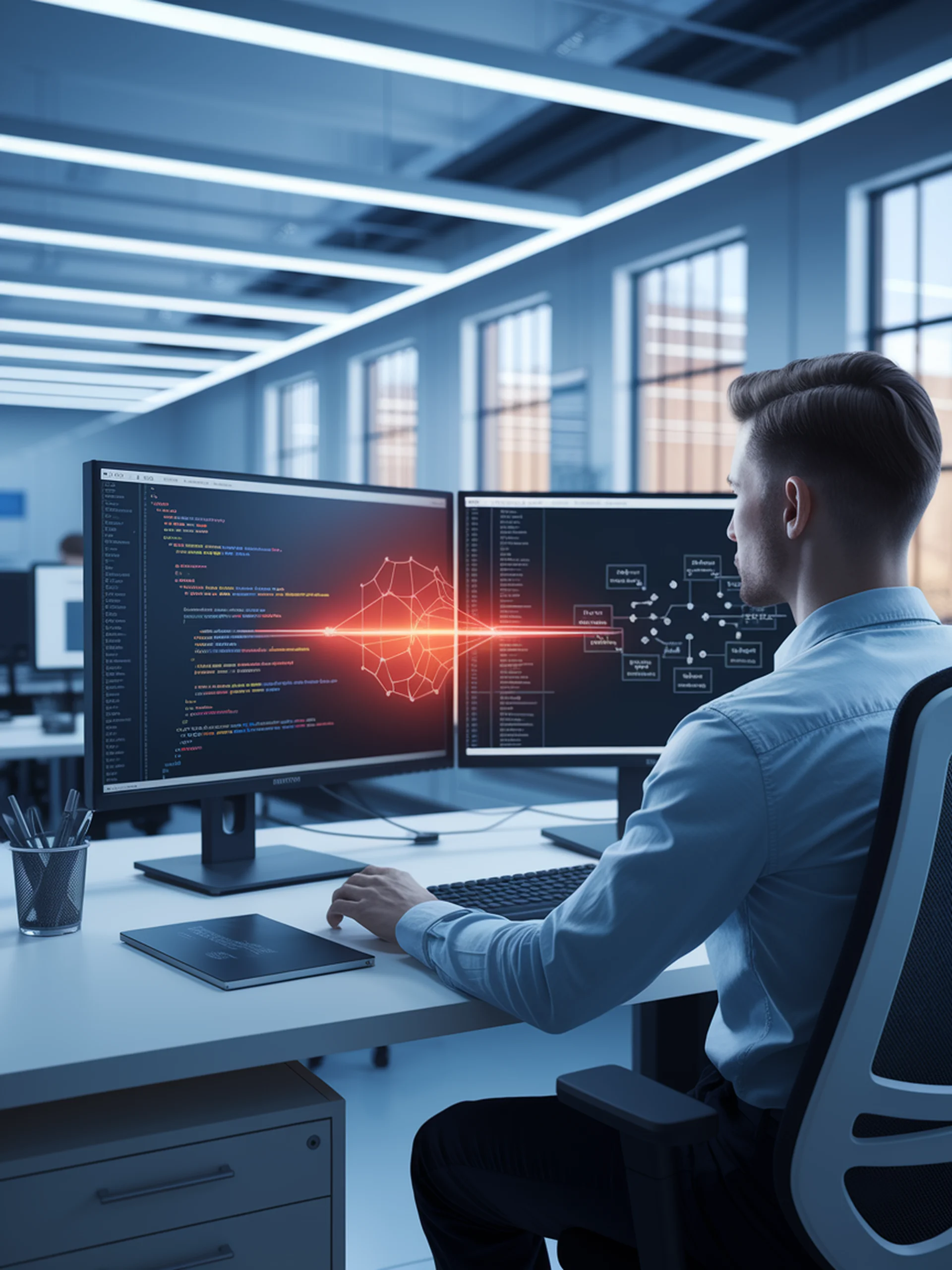
Smart Switching: Code vs. Text Reasoning in LLMs
Optimizing when LLMs should code rather than reason through text
This research develops a framework to intelligently determine when large language models should execute code versus use textual reasoning to solve problems.
- Code execution achieves 100% success on certain tasks while avoiding the computational overhead of textual reasoning
- Textual reasoning struggles with complex math, logic, and search problems that code handles efficiently
- The authors propose methods to effectively steer LLM behavior between these two approaches
- This engineering breakthrough improves efficiency and effectiveness in AI problem-solving systems
For engineering teams, this research offers practical techniques to optimize LLM implementations by selecting the most efficient problem-solving method based on the task type.
Steering Large Language Models between Code Execution and Textual Reasoning