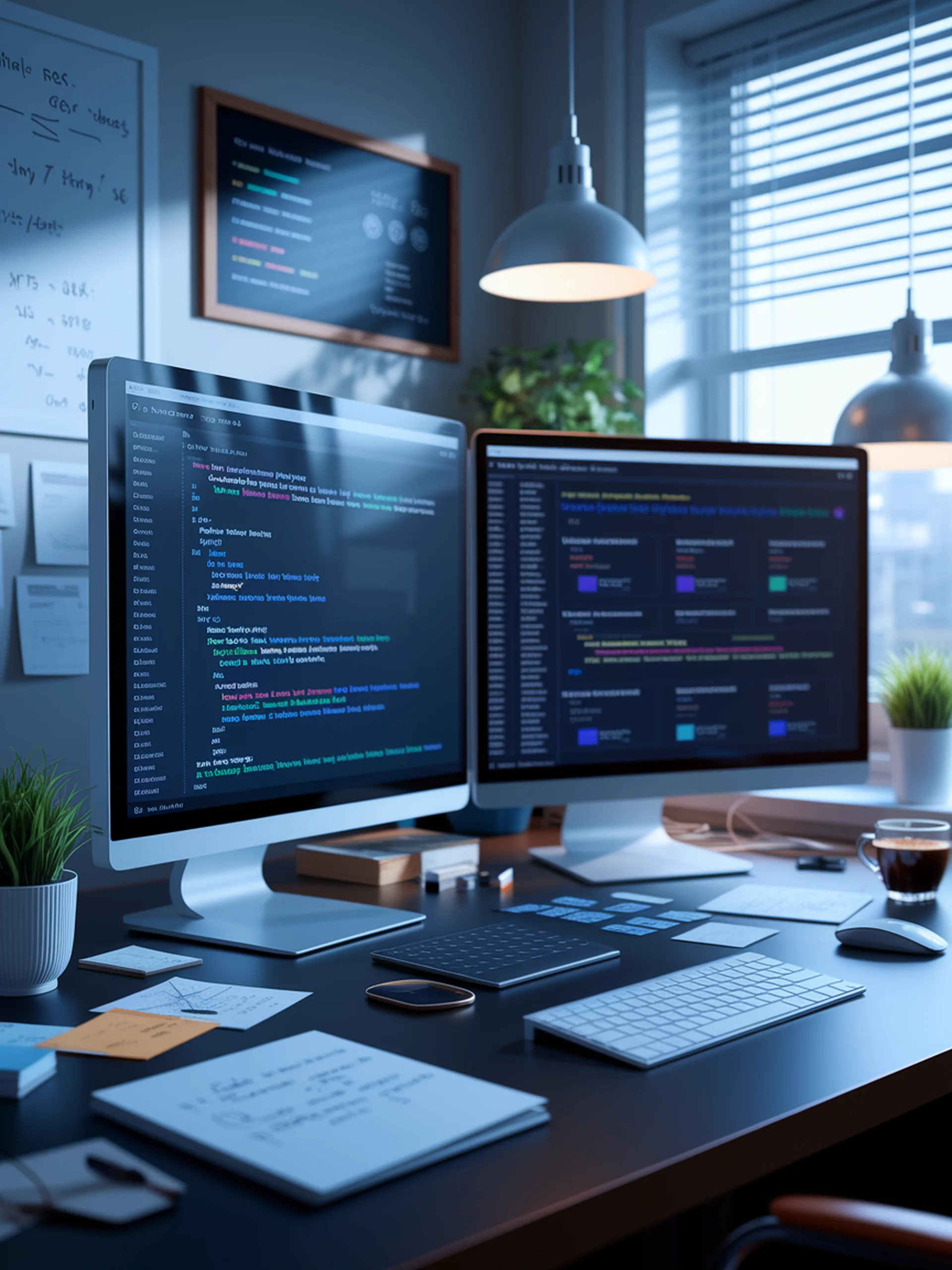
Optimizing RAG Systems for Code Generation
Balancing Effectiveness and Efficiency in Retrieval for Coding Tasks
This research evaluates how different demonstration retrievers impact both quality and performance in Retrieval-Augmented Generation (RAG) systems for coding tasks.
- Dense retrievers (like CodeBERT) deliver higher quality code generation but with slower retrieval times
- Sparse retrievers (like BM25) offer significantly faster retrieval with only modest quality reduction
- Hybrid approaches can achieve optimal efficiency-effectiveness balance for real-world applications
- Key finding: Sparse retrievers can be 2-8x faster while maintaining 95% of the effectiveness
For engineering teams, this research provides practical guidance on implementing RAG systems that deliver high-quality code assistance without prohibitive computational overhead, enabling more responsive developer tools.
Evaluating the Effectiveness and Efficiency of Demonstration Retrievers in RAG for Coding Tasks