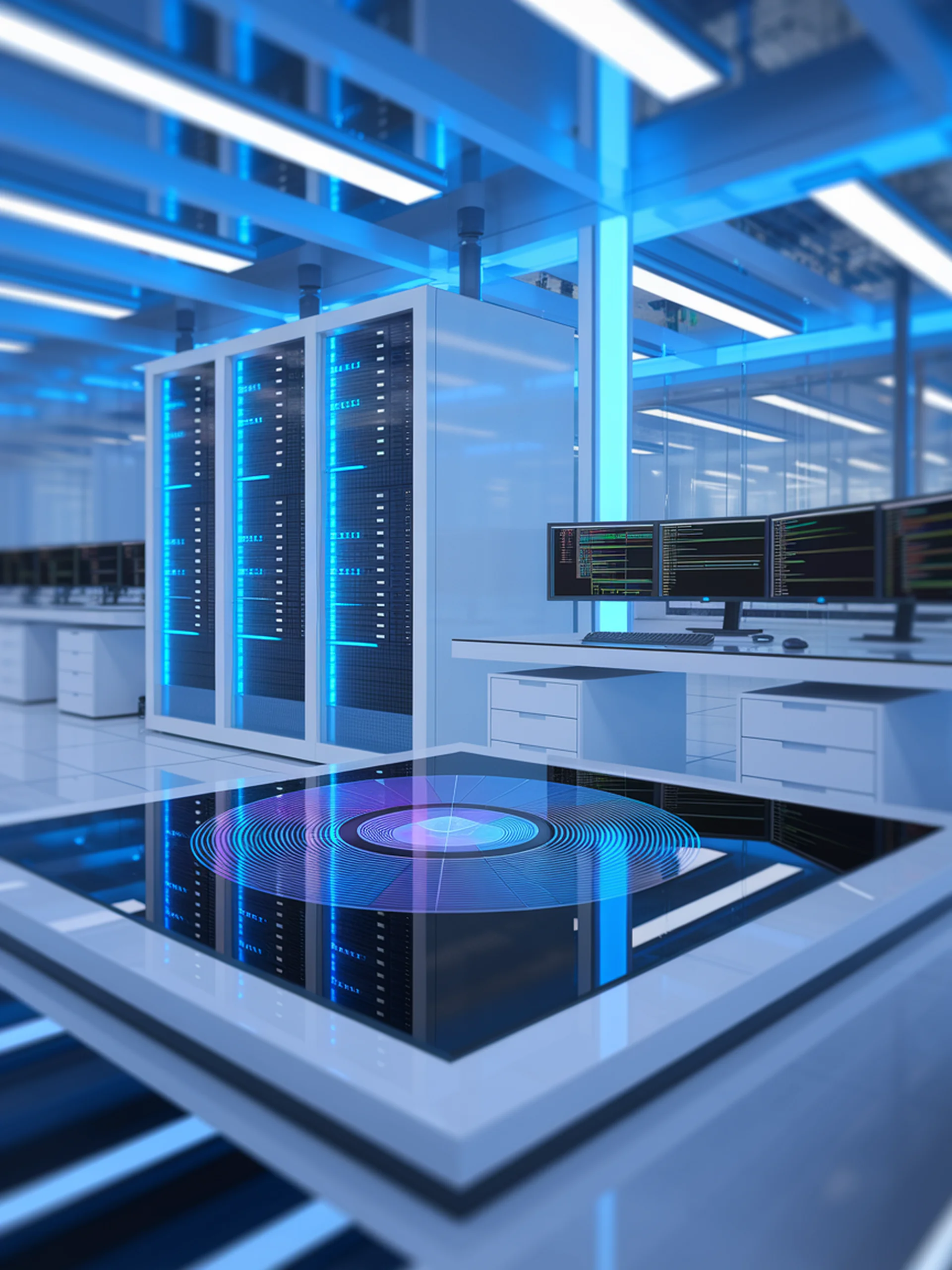
Probabilistic Analysis for LLM-Enabled Software
A framework for reliability and verification in AI systems
This research introduces a probabilistic framework to systematically analyze and improve LLM-enabled software systems by modeling distributions of semantically equivalent outputs.
- Focuses on Transference Models that utilize LLMs to transform inputs into outputs
- Enables systematic evaluation and iteration of LLM components in software
- Addresses core reliability and verifiability challenges in AI-enabled systems
- Provides engineers with a structured approach to improve output consistency
For engineering teams, this framework offers a practical methodology to assess, verify, and enhance LLM components within larger software systems, reducing risks associated with unpredictable AI outputs.
Towards a Probabilistic Framework for Analyzing and Improving LLM-Enabled Software