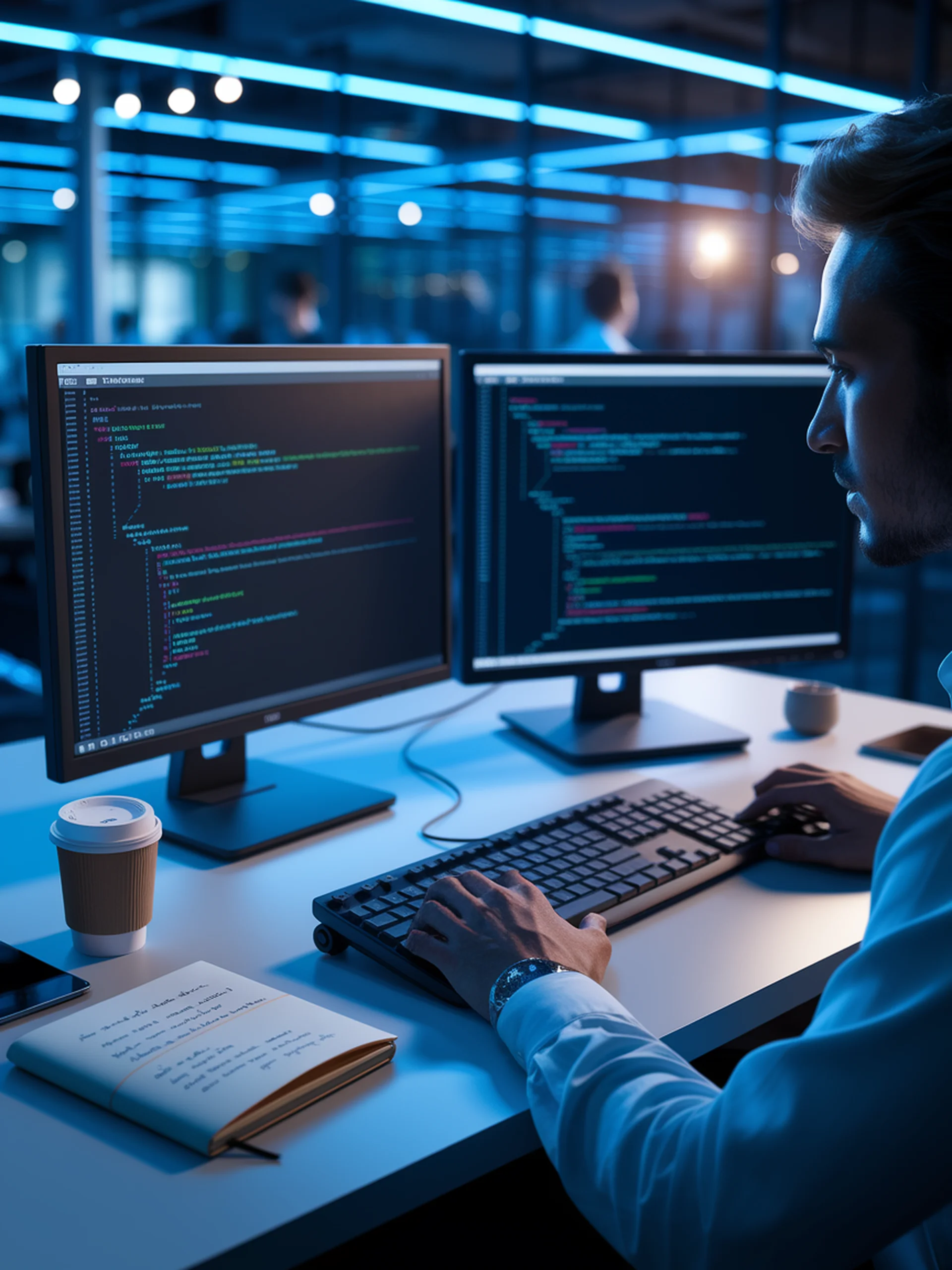
Self-Improving Code Generation
How LLMs Can Refine Their Own Code Through Adaptive Critique
RefineCoder introduces a novel approach where code-generating language models iteratively improve their outputs through self-critique rather than relying solely on teacher models.
- Implements Adaptive Critique Refinement (ACR) enabling models to evaluate and enhance their own generated code
- Demonstrates superior performance compared to traditional supervised fine-tuning methods
- Reduces dependency on expert-annotated training data by leveraging self-improvement cycles
- Creates more robust, accurate code solutions through iterative refinement
This research significantly advances engineering capabilities by creating more reliable automated coding assistants that can identify and correct their own mistakes, potentially accelerating software development workflows.