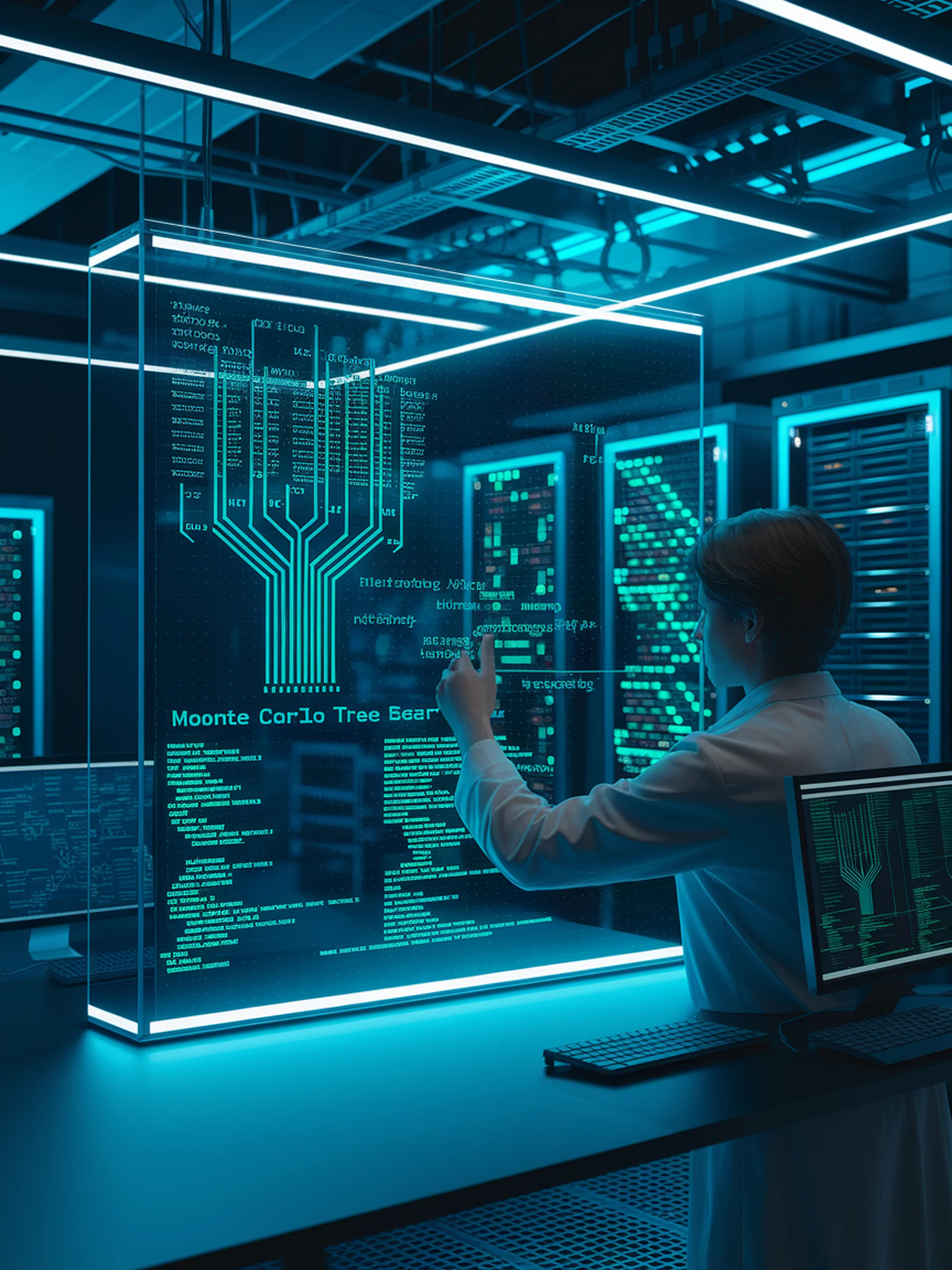
Enhancing AI Code Evaluation
Using Monte Carlo Tree Search to improve LLM-based code assessment
MCTS-Judge is a novel framework that significantly improves how large language models evaluate code correctness by applying strategic problem decomposition and multi-perspective reasoning.
- Combines LLMs with Monte Carlo Tree Search to break complex programming evaluations into simpler sub-problems
- Demonstrates superior performance compared to standard LLM-as-Judge approaches
- Offers a resource-efficient alternative to scaling model size for reasoning tasks
- Provides more reliable code assessments in education and software development contexts
This research matters for engineering teams by offering more accurate automated code evaluation tools that could enhance code quality, accelerate review processes, and improve educational programming assessment.
MCTS-Judge: Test-Time Scaling in LLM-as-a-Judge for Code Correctness Evaluation