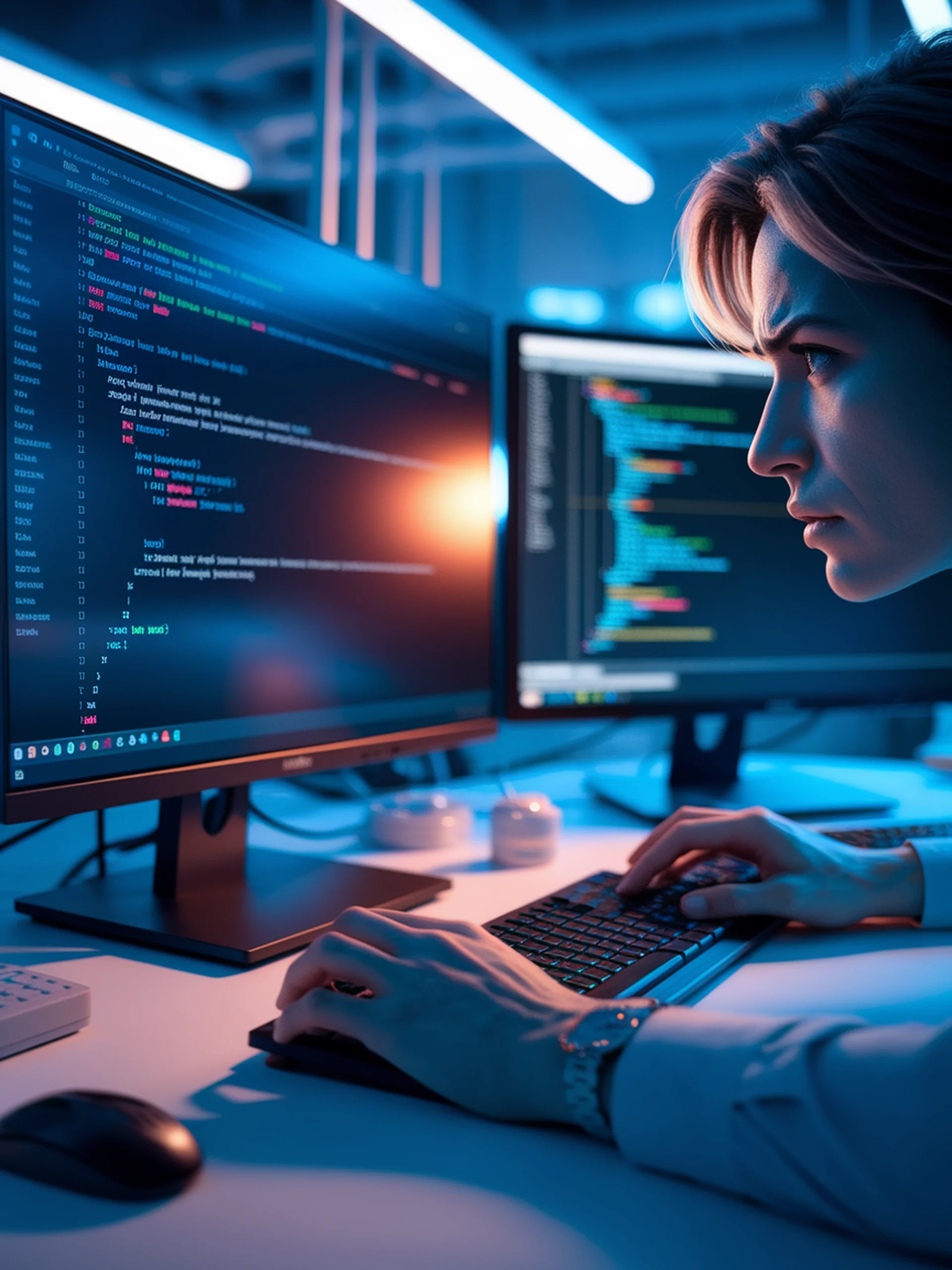
Testing LLMs' Code Error Prediction
A novel benchmark for evaluating code understanding beyond synthesis
ThrowBench evaluates LLMs' ability to predict runtime exceptions, offering a new dimension for assessing code comprehension capabilities.
- Tests LLMs on predicting when code will fail at runtime
- Provides an alternative to standard code synthesis benchmarks
- Addresses concerns about benchmark contamination and leakage
- Enables evaluation of deeper code understanding for security applications
This research advances security by improving our ability to assess if AI models can detect potential runtime errors that could lead to vulnerabilities in software systems.
ThrowBench: Benchmarking LLMs by Predicting Runtime Exceptions