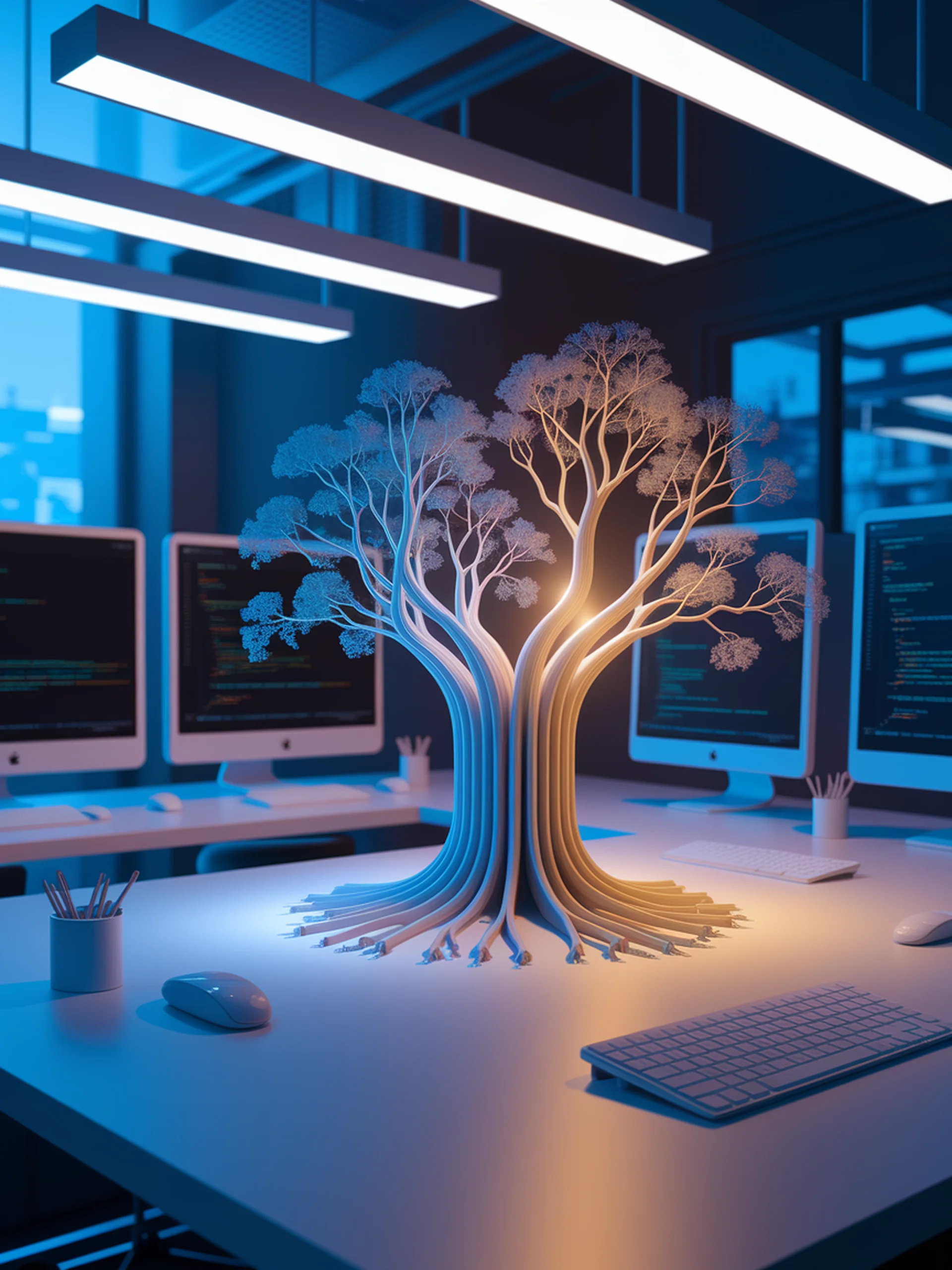
Enhancing AI Coding Assistants
A Dynamic Approach to Improve LLM Decision-Making in Coding Tasks
DARS introduces an adaptive tree traversal method that significantly improves how AI coding agents make decisions without requiring extensive manual intervention.
- Implements Dynamic Action Re-Sampling to help LLMs reconsider sub-optimal coding decisions
- Enables coding agents to explore alternative solutions more effectively
- Achieves higher success rates in software development tasks through strategic backtracking
- Requires minimal additional computation compared to existing approaches
This research matters because it addresses a critical limitation in current AI coding assistants, potentially making them more reliable partners for software engineers in real-world development environments.
DARS: Dynamic Action Re-Sampling to Enhance Coding Agent Performance by Adaptive Tree Traversal