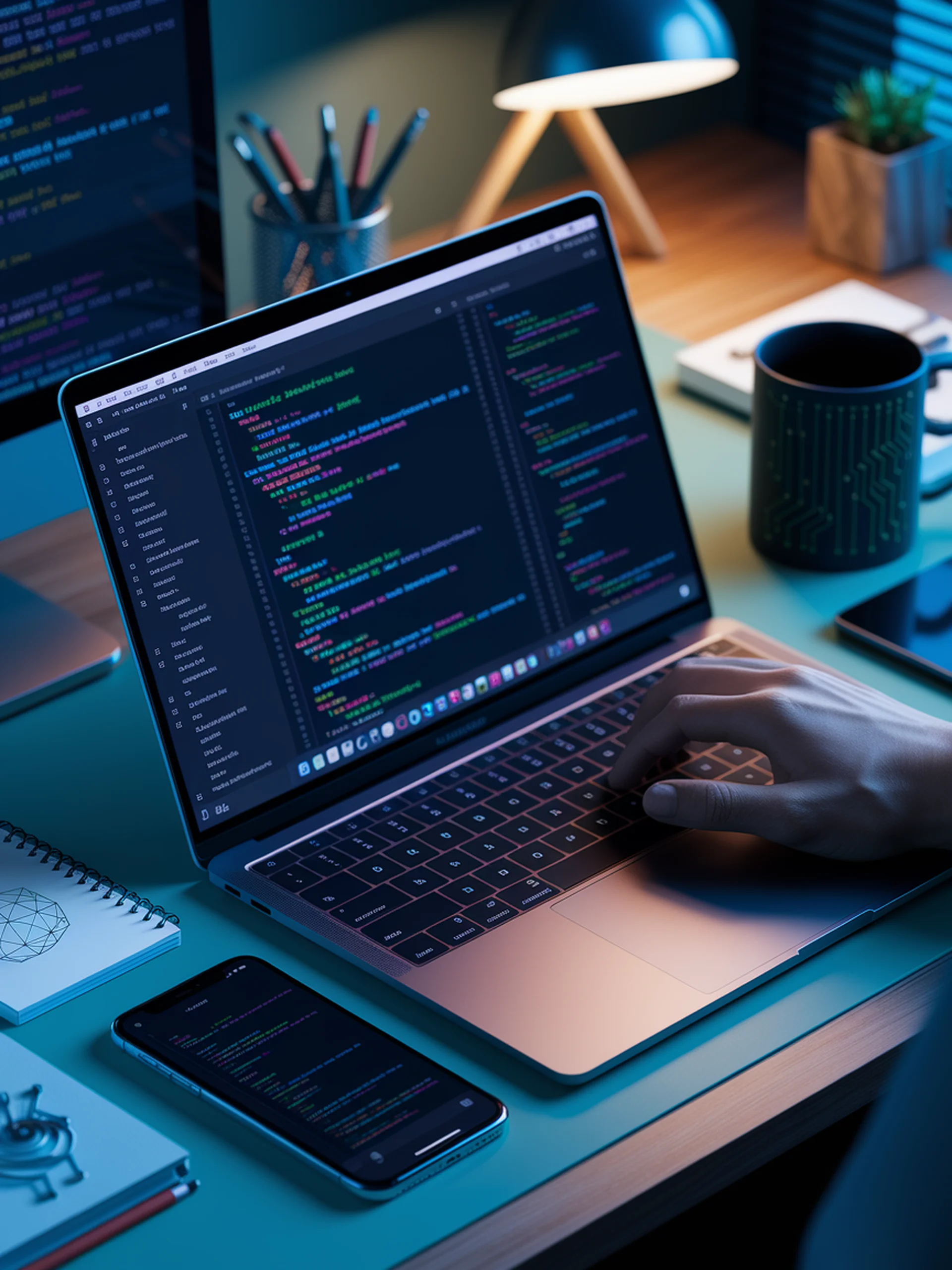
Smarter Code Completion with Long-Context LLMs
Training models to effectively utilize repository-wide context
aiXcoder-7B-v2 addresses a critical limitation in LLMs for code completion: their inability to effectively utilize information from long repository contexts.
- Identified that LLMs often ignore useful information in long contexts even when relevant APIs or similar code are present
- Developed a novel training approach to enhance LLMs' ability to utilize repository-level context
- Created a model that achieves improved performance in repository-level code completion tasks
- Demonstrated practical engineering benefits through better context utilization in real-world coding scenarios
This research matters for software engineering by increasing developer productivity with more contextually relevant code suggestions that understand entire codebases rather than just immediate surroundings.
aiXcoder-7B-v2: Training LLMs to Fully Utilize the Long Context in Repository-level Code Completion