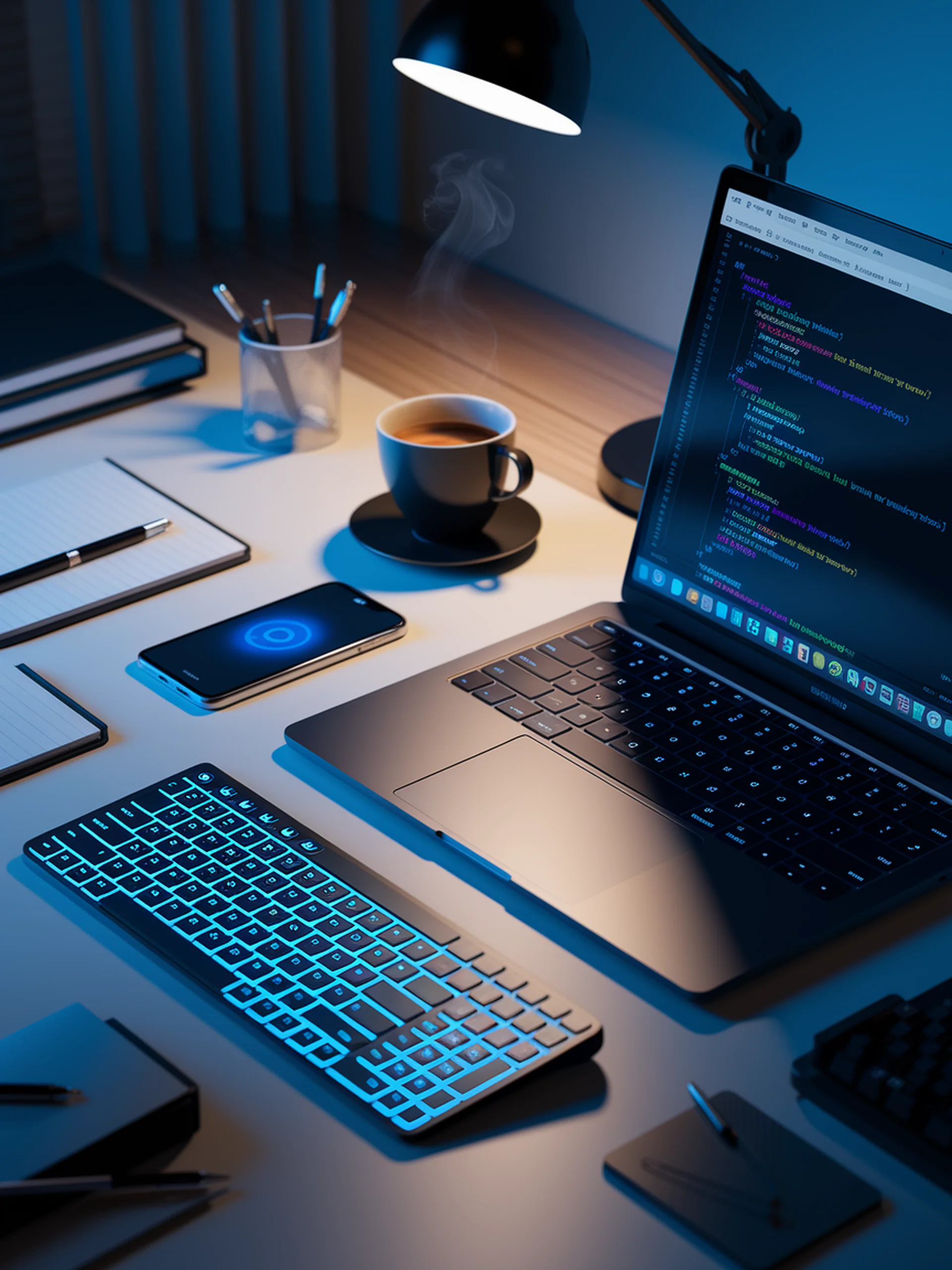
Evaluating LLMs for Interactive Code Generation
New benchmark reveals how well AI follows instructions when writing code
CodeIF-Bench introduces a comprehensive framework for evaluating how effectively large language models follow instructions during interactive code generation sessions.
- Addresses a critical gap in existing benchmarks that primarily assess single-turn functional correctness
- Evaluates LLMs across multiple dimensions: accuracy, instruction adherence, and multi-turn interaction capabilities
- Provides insights into how well AI coding assistants understand and implement specific developer requirements
- Covers diverse programming scenarios across engineering, support, education, and creative tasks
This research matters for Engineering teams as it helps identify which AI coding assistants can most effectively understand and implement specific requirements, potentially improving developer productivity and code quality.