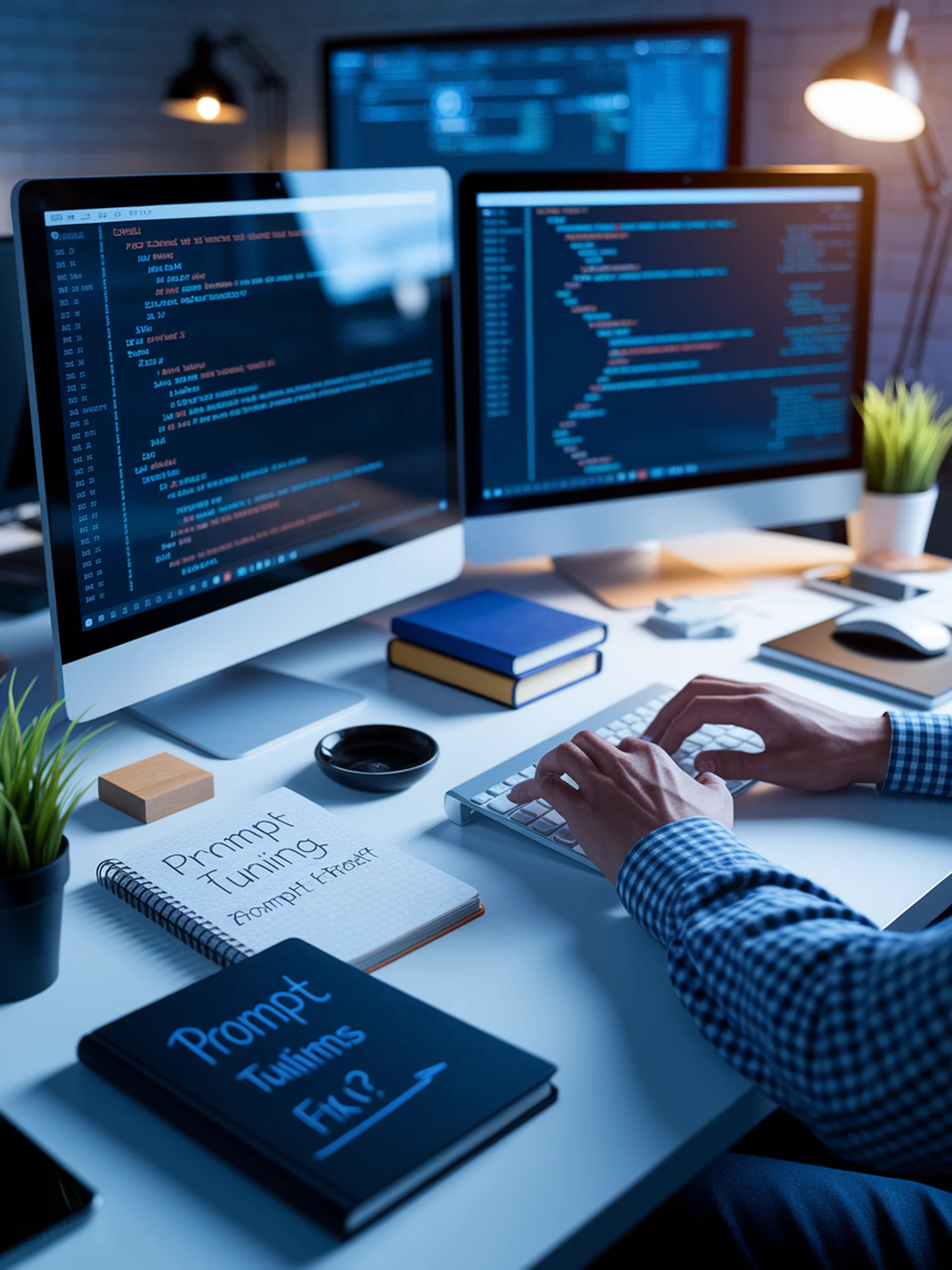
Boosting Automated Program Repair with LLMs
Knowledge Prompt Tuning for Enhanced Bug Fixes with Limited Data
This research introduces an adapted prompt tuning approach that improves automated program repair capabilities when training data is scarce, addressing a common limitation in practical applications.
- Combines knowledge prompt tuning with large language models to enhance bug-fixing accuracy
- Outperforms standard fine-tuning methods in data-limited scenarios
- Demonstrates better generalization capabilities across different programming contexts
- Improves software reliability through more effective automatic error detection and correction
This advancement matters for Engineering teams by offering more efficient software maintenance workflows, reducing debugging time, and improving code quality with fewer resources—enabling developers to focus on creating new features rather than fixing bugs.
Adapting Knowledge Prompt Tuning for Enhanced Automated Program Repair