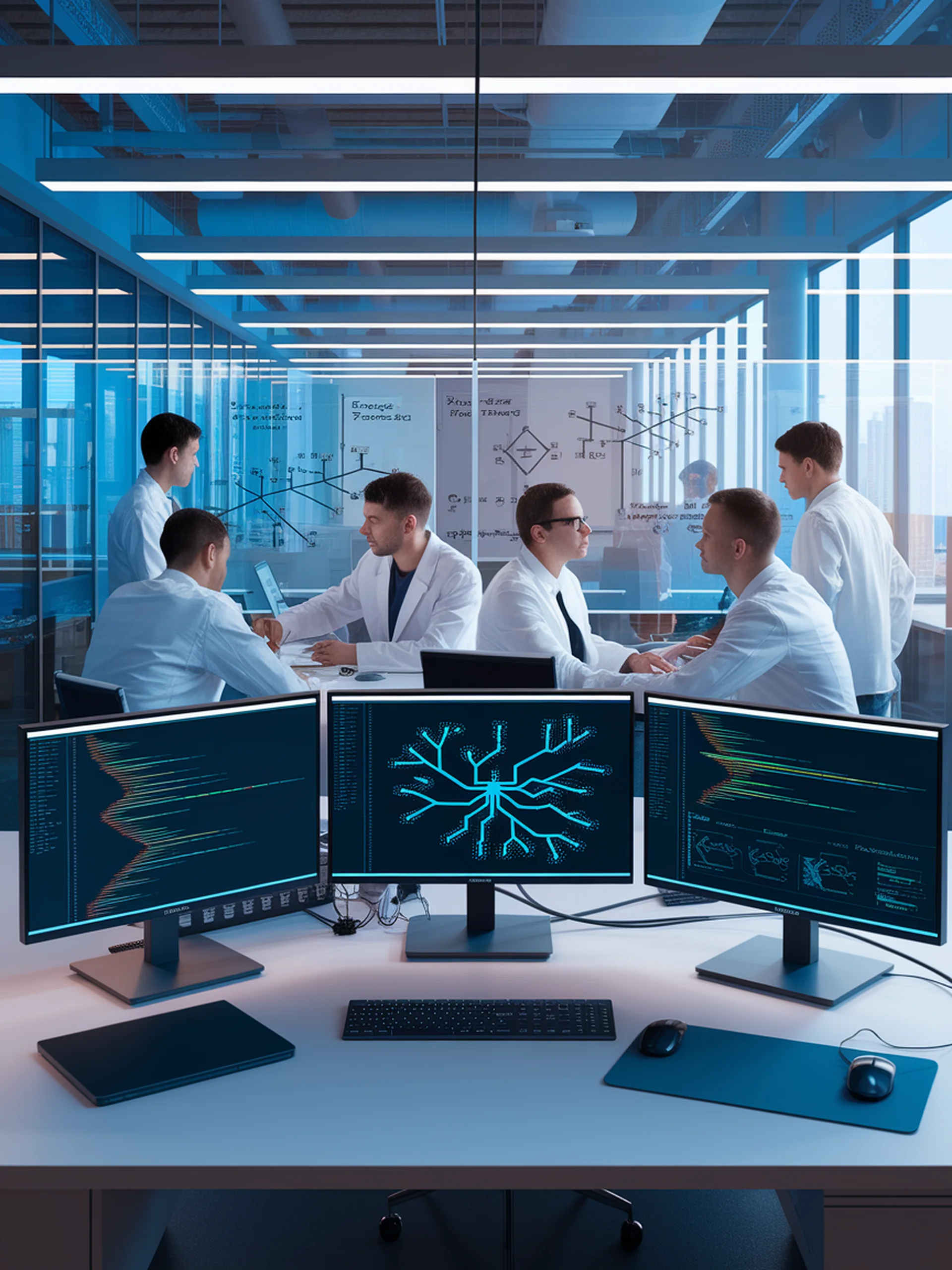
Dynamic LLM Code Evaluation Reimagined
Beyond Static Benchmarks with Monte Carlo Tree Search
Prism introduces a flexible, dynamic framework for benchmarking LLMs' code generation capabilities that evolves with advancing AI technologies.
- Uses Monte Carlo Tree Search to explore possible solution paths dynamically
- Creates comprehensive evaluation scenarios that adapt to model strengths and weaknesses
- Overcomes limitations of static benchmarks that quickly become obsolete
- Provides more nuanced assessment of LLM capabilities in code generation tasks
This research advances engineering practice by enabling more accurate and adaptable evaluation of AI coding assistants, essential for establishing trust in AI systems for software development.