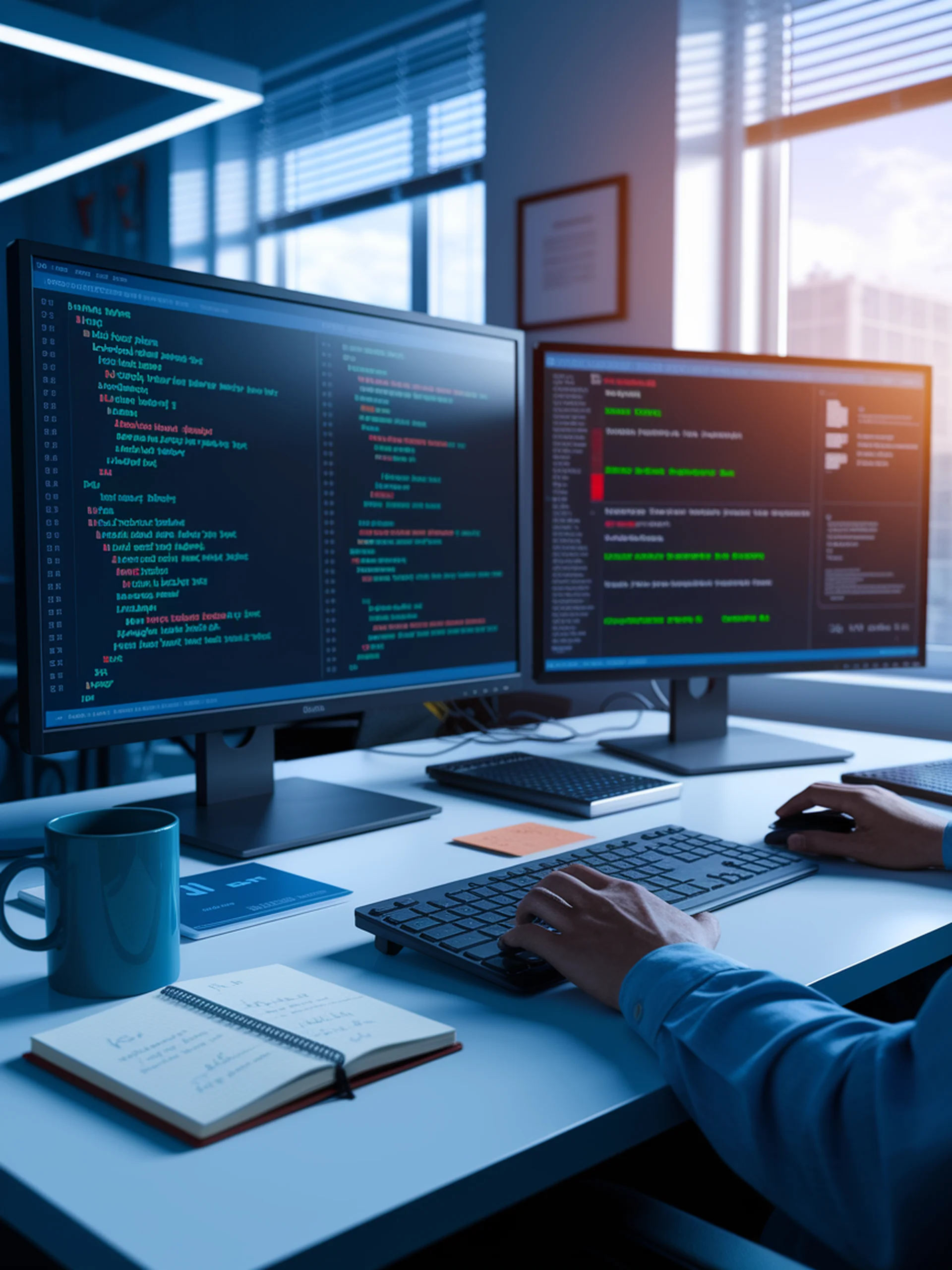
Enhancing LLMs for Code Repair
New benchmark evaluates how well AI models use feedback to fix bugs
FeedbackEval introduces a systematic benchmark for measuring how well large language models understand and utilize different types of feedback when repairing code.
- Evaluates LLMs' ability to comprehend error messages, test cases, and natural language explanations
- Measures performance across diverse programming tasks and feedback types
- Provides insights into how different models handle feedback-driven code repair
- Establishes a standardized framework for consistent evaluation
This research is critical for software engineering teams seeking to integrate AI-powered code assistance tools that can effectively respond to developer feedback, potentially reducing debugging time and improving code quality.
FeedbackEval: A Benchmark for Evaluating Large Language Models in Feedback-Driven Code Repair Tasks