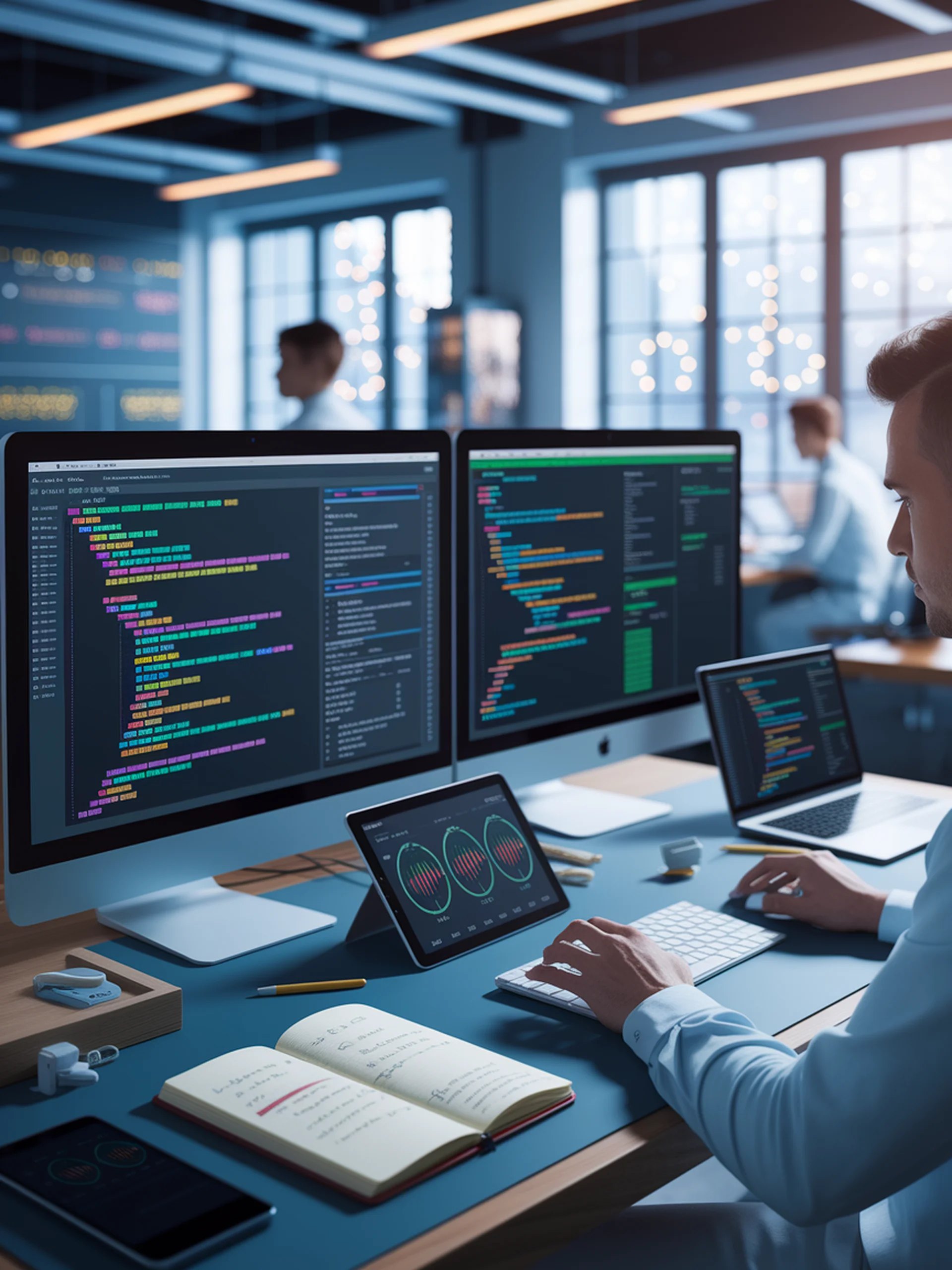
Evaluating Code Assistants: Beyond the Hype
A robust methodology for measuring AI coding tool quality
This research introduces a structured evaluation pipeline for measuring the accuracy and reliability of LLM-based coding assistants using real code snippets.
- Focuses on TabbyML, an open-source code assistant, evaluating its performance on standard algorithms and data structures
- Employs software engineering metrics including cyclomatic complexity and Halstead's metrics
- Addresses the challenge of objectively measuring AI-generated code quality
- Provides a reproducible framework for evaluating other coding assistants
For engineering teams, this research offers a systematic way to assess whether AI coding tools actually improve developer productivity and code quality before adoption.
Quality evaluation of Tabby coding assistant using real source code snippets