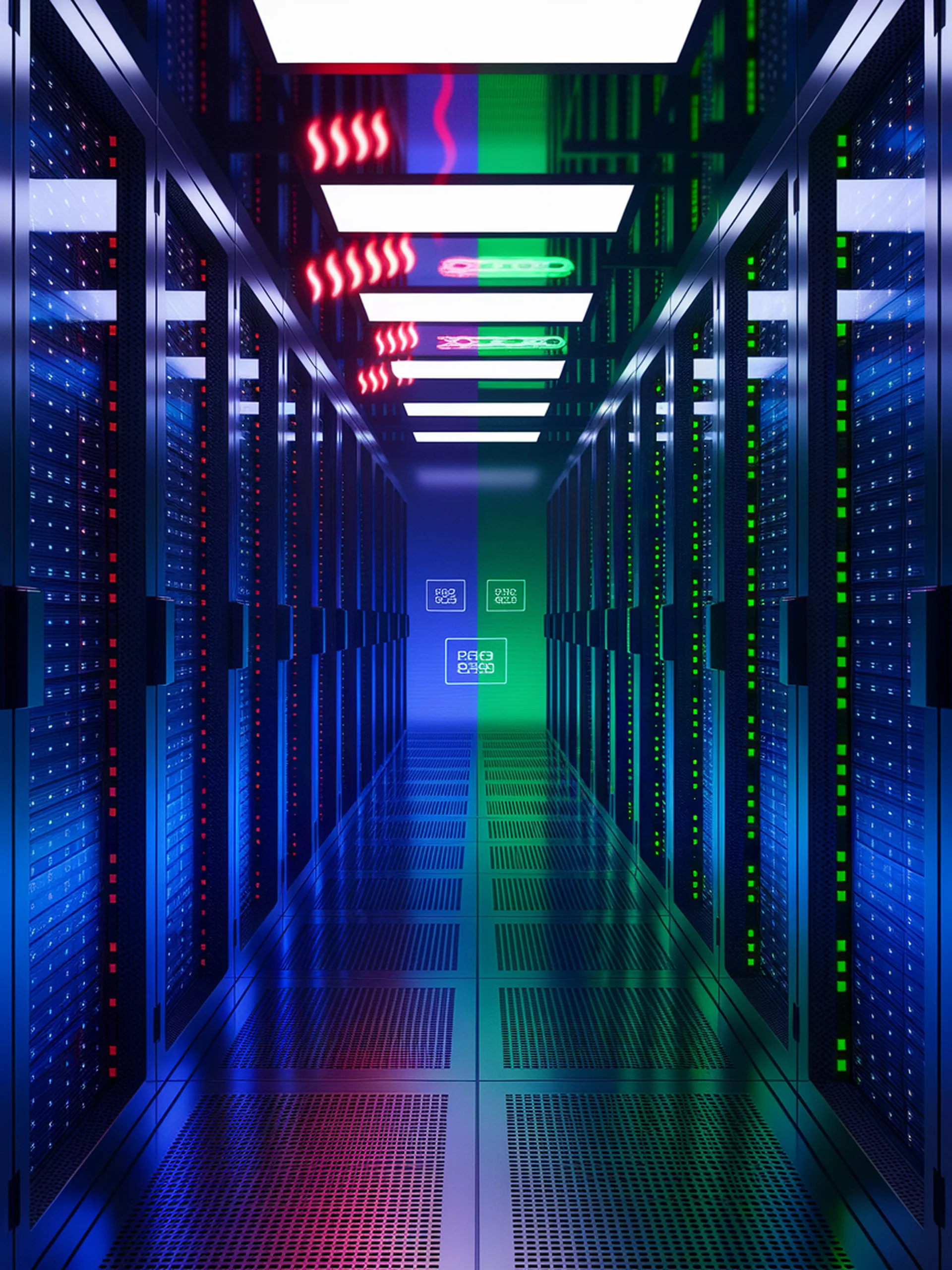
Sustainable AI: Greening Large Language Models
Balancing energy efficiency with performance for real-world applications
This research introduces an end-to-end pipeline for optimizing LLMs to reduce energy consumption while maintaining performance for practical applications.
- Implements quantization and pruning techniques to create energy-efficient LLM variants
- Demonstrates less than 5% performance drop while achieving significant energy savings
- Provides a practical framework for sustainable AI deployment in network support systems
- Evaluates models on real-world fault ticket analysis in communication networks
For engineering teams, this research offers immediate pathways to deploy powerful language models in resource-constrained environments, substantially reducing operational costs and environmental impact without sacrificing essential capabilities.
Energy-Aware LLMs: A step towards sustainable AI for downstream applications