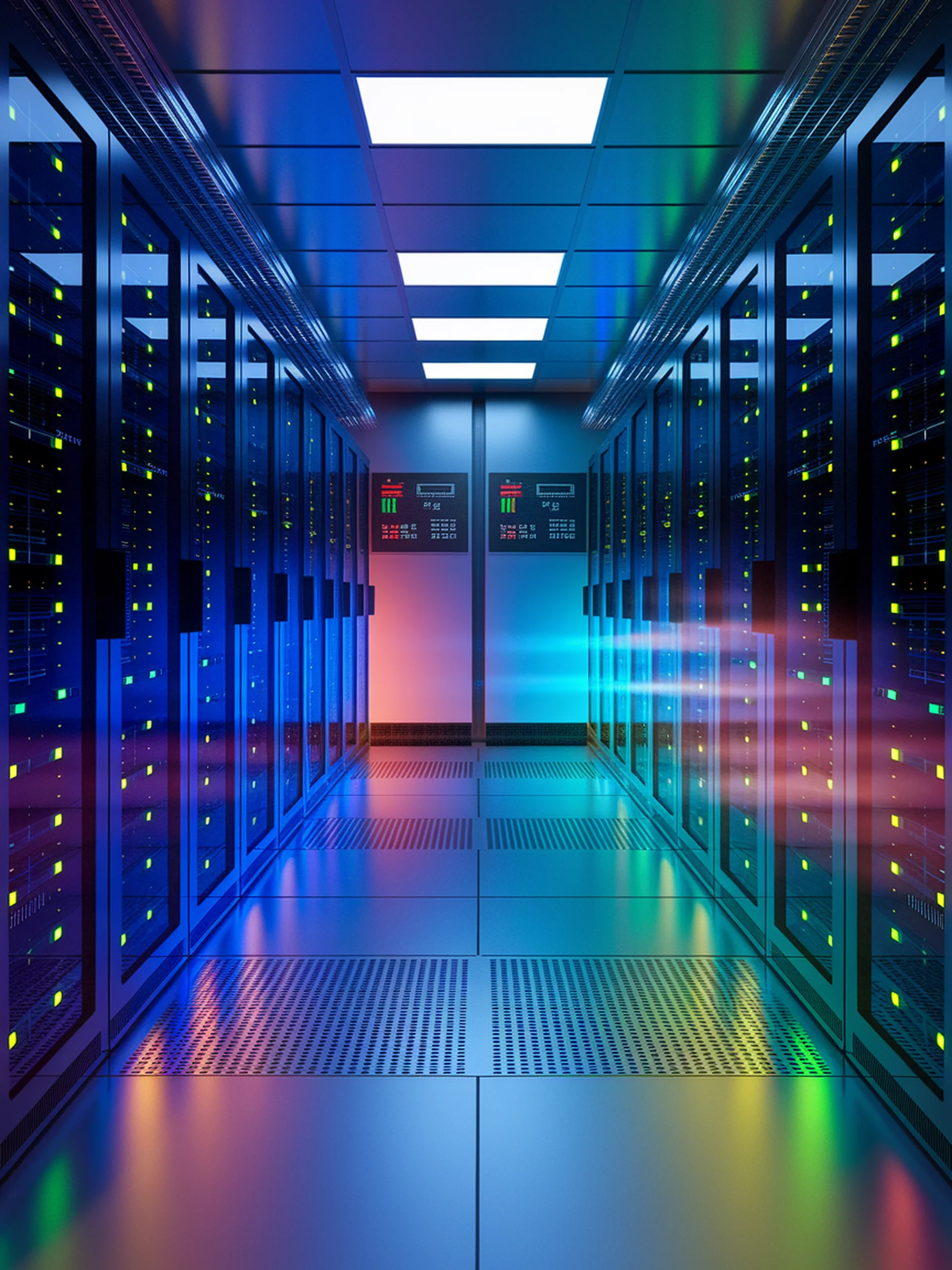
Energy Efficiency in AI Operations
Comparing energy consumption patterns in discriminative and generative AI systems
This research provides critical insights into the energy consumption profiles of AI models across both discriminative and generative paradigms, enabling more sustainable AI deployment strategies.
- Traditional ML models show varied energy needs based on architecture and hyperparameter choices
- For Large Language Models, energy consumption scales dramatically with model size
- Service request patterns significantly impact overall energy footprint of generative AI systems
- Researchers identified specific energy-efficient practices for both training and inference phases
This work matters for Engineering teams by offering empirical guidance to reduce the environmental impact of AI operations while maintaining performance, essential as AI deployment continues to scale globally.