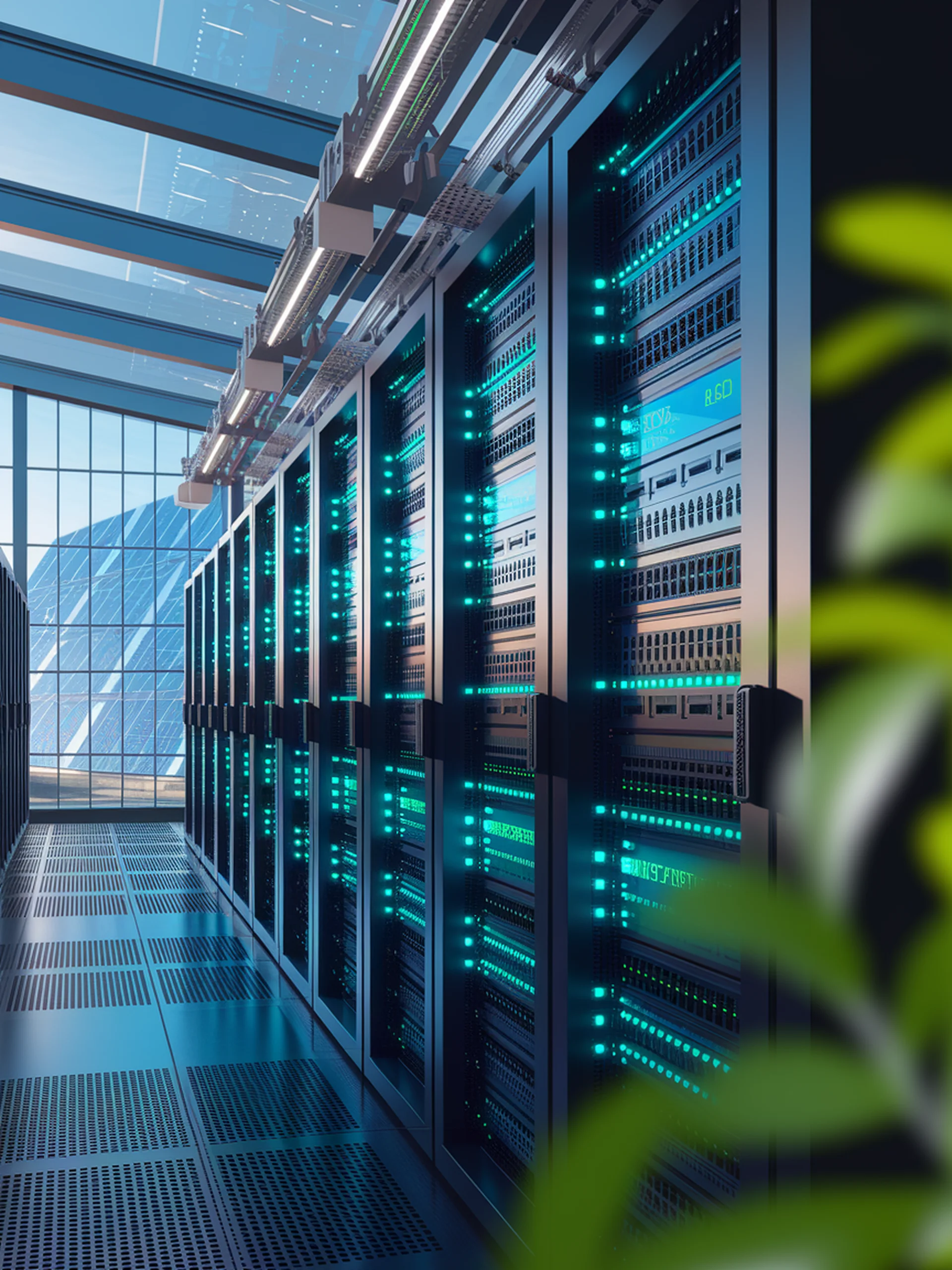
Greening LLMs: The Efficiency Imperative
Reducing the carbon footprint of large language models through optimization
Research demonstrating how strategic optimization techniques can substantially reduce the environmental impact of LLM deployments while maintaining performance.
- Quantization methods significantly lower energy consumption during inference
- Local inference approaches reduce cloud computing dependencies and associated emissions
- Framework provides measurable metrics for evaluating LLM sustainability
- Case study demonstrates real-world application of these optimization techniques
This engineering research offers practical solutions for organizations seeking to balance AI capabilities with environmental responsibility and cost efficiency.
Optimizing Large Language Models: Metrics, Energy Efficiency, and Case Study Insights