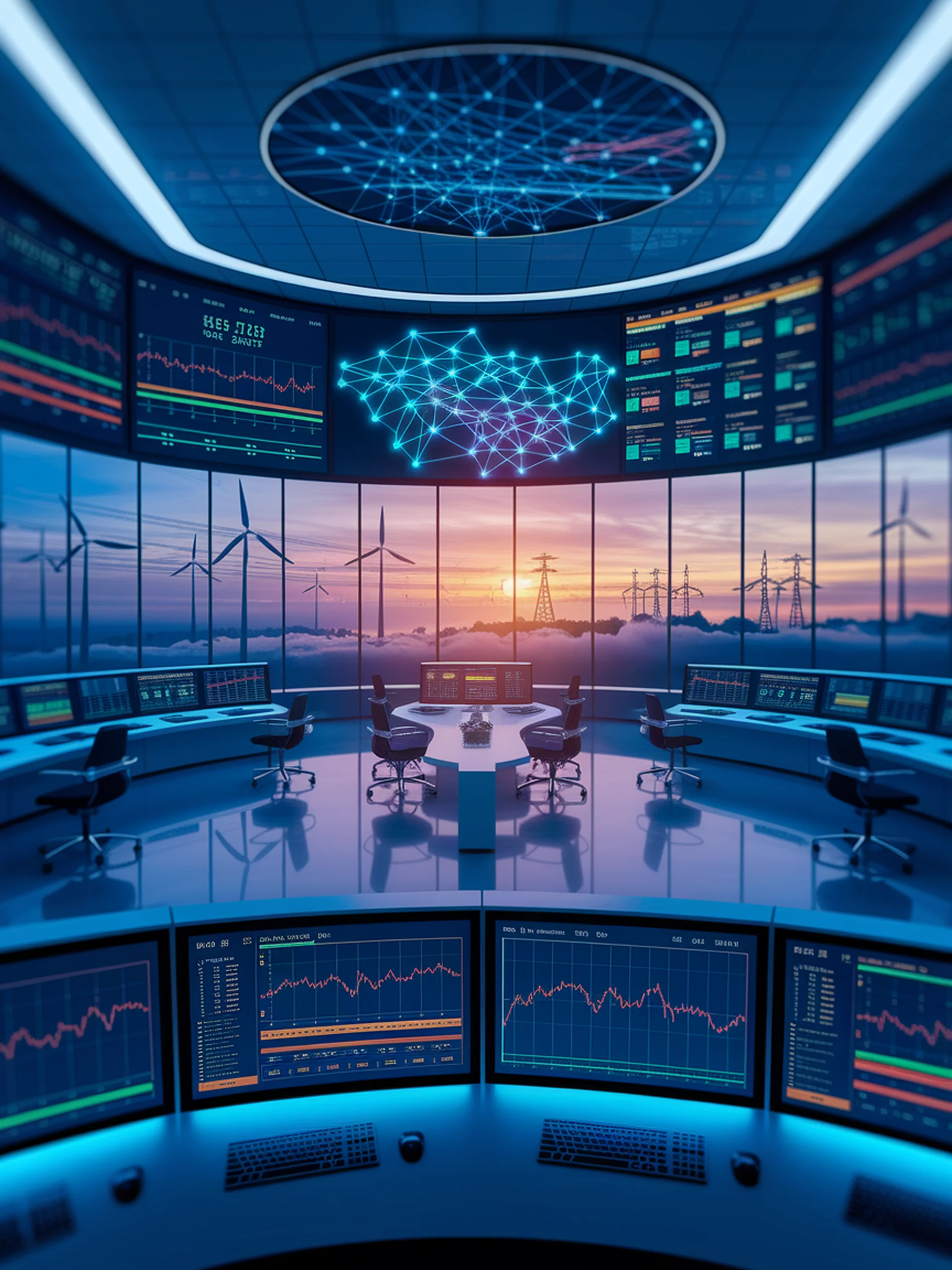
Zero-Shot Load Forecasting with LLMs
Transforming energy prediction without historical data
This research introduces a novel framework leveraging large language models to perform accurate load forecasting in integrated energy systems without requiring historical data.
- Combines multi-task learning with LLMs to enable zero-shot forecasting across different energy carriers
- Demonstrates superior transferability compared to traditional methods in diverse energy scenarios
- Achieves industry-competitive accuracy while eliminating the need for extensive historical datasets
This innovation addresses critical engineering challenges in renewable energy integration, enabling more reliable grid management and energy security as power systems become increasingly complex with multiple energy carriers.