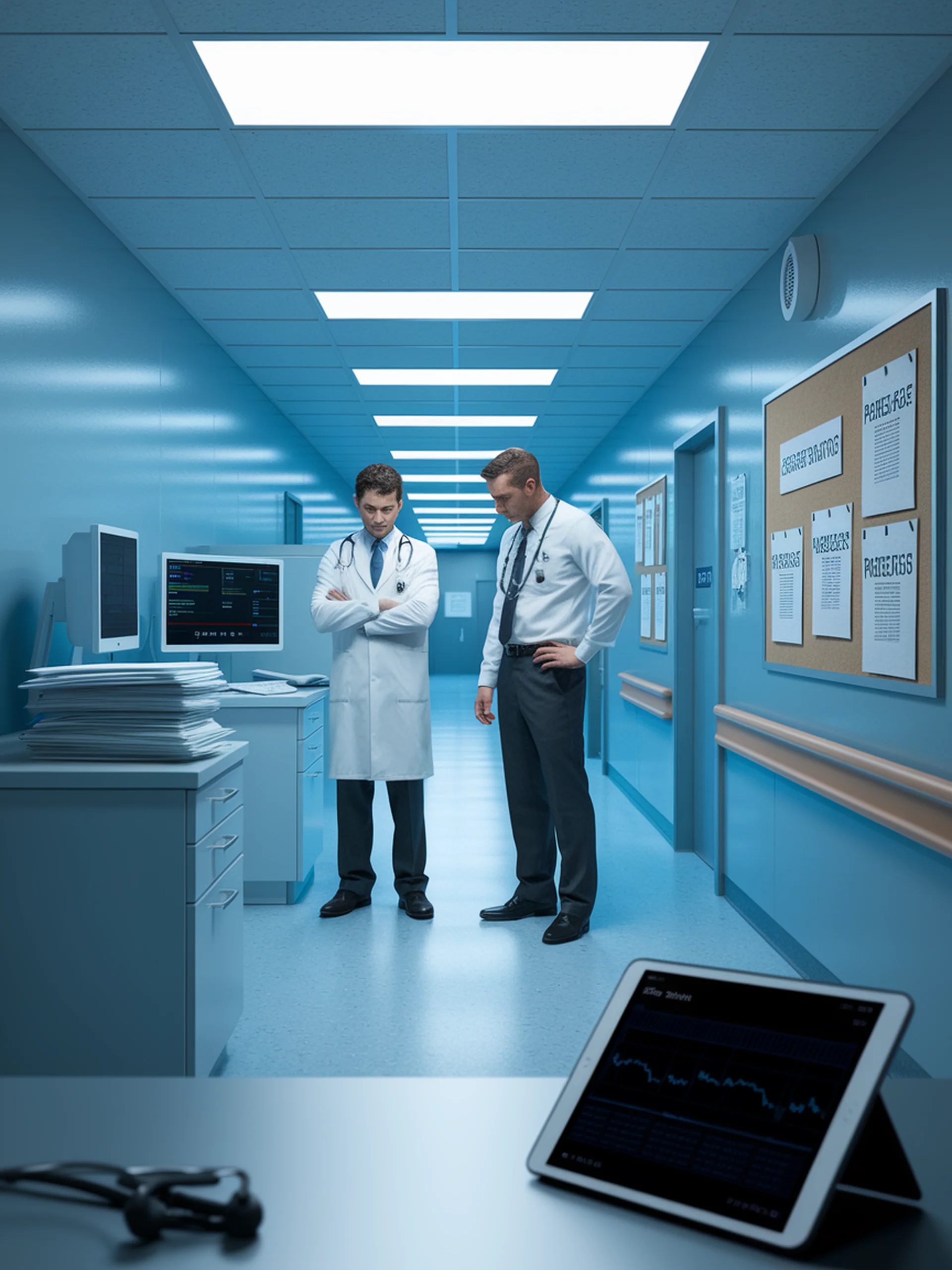
Current Challenges to Adoption
Key barriers slowing AI implementation in hospitals
Data Silos and Digitalization Gaps
- Much of German healthcare was historically paper-based or has fragmented IT systems
- Accelerating healthcare digitalization is urgent – an area where Germany lags
- If EHRs and data interoperability aren't in place, AI algorithms cannot access needed data
- Data silos within hospitals (lab, radiology, notes in separate systems) hinder AI development
Resource and Expertise Limitations
- Survey of German hospitals identified lack of resources as the primary barrier:
- Shortage of skilled AI staff and data scientists
- Limited technical know-how for implementation
- Insufficient financial support for AI projects
- Few hospitals have in-house AI experts to develop or manage AI initiatives
- Many facilities have no clear AI strategy or roadmap
- Smaller hospitals especially may see AI as a luxury amid other pressing needs
Integration and Workflow Challenges
- Seamlessly fitting AI into clinical workflow is non-trivial
- If using AI is cumbersome or disruptive, clinicians will resist adoption
- User interface and interoperability are critical success factors
- AI must slot into existing software (EHRs, imaging systems) to be useful
- Staff training requirements add complexity to implementation
Trust, Acceptance and Cultural Factors
- Medicine is conservative regarding patient safety—clinicians are cautious about new tools
- Building trust in AI requires evidence, transparency, and time for culture change
- Some staff fear AI might replace them, leading to resistance
- Patient acceptance varies, with some discomfort about algorithms in their care
- Hospitals must engage in education for both staff and patients about AI benefits