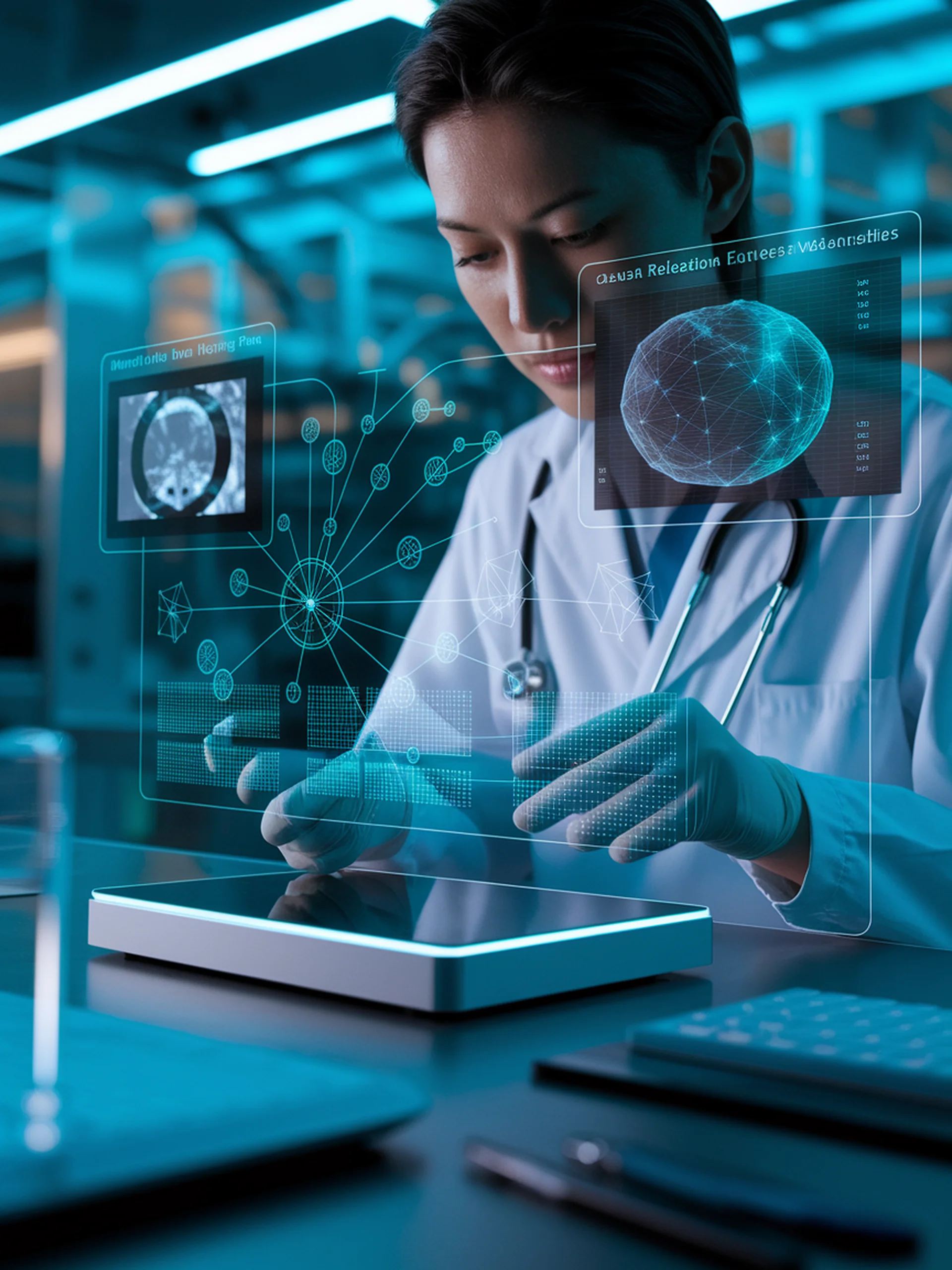
Leveraging LLMs for Causal Validation
Comparing Prompting vs. Fine-tuning Approaches for Medical Causality Assessment
This research explores how Large Language Models can replace human experts in validating causality in medical and biological contexts.
- LLMs evaluate whether causal connections between variables can be inferred from text
- Compares two approaches: prompt engineering vs. fine-tuning for causality assessment
- Focuses on biomedical datasets to validate causal relationships
- Potentially reduces dependence on manual expert validation in causal discovery
Why it matters: This advancement could accelerate medical research by automating the validation of causal relationships, reducing the bottleneck created by the need for human expert assessment in causal discovery methods.
Prompting or Fine-tuning? Exploring Large Language Models for Causal Graph Validation