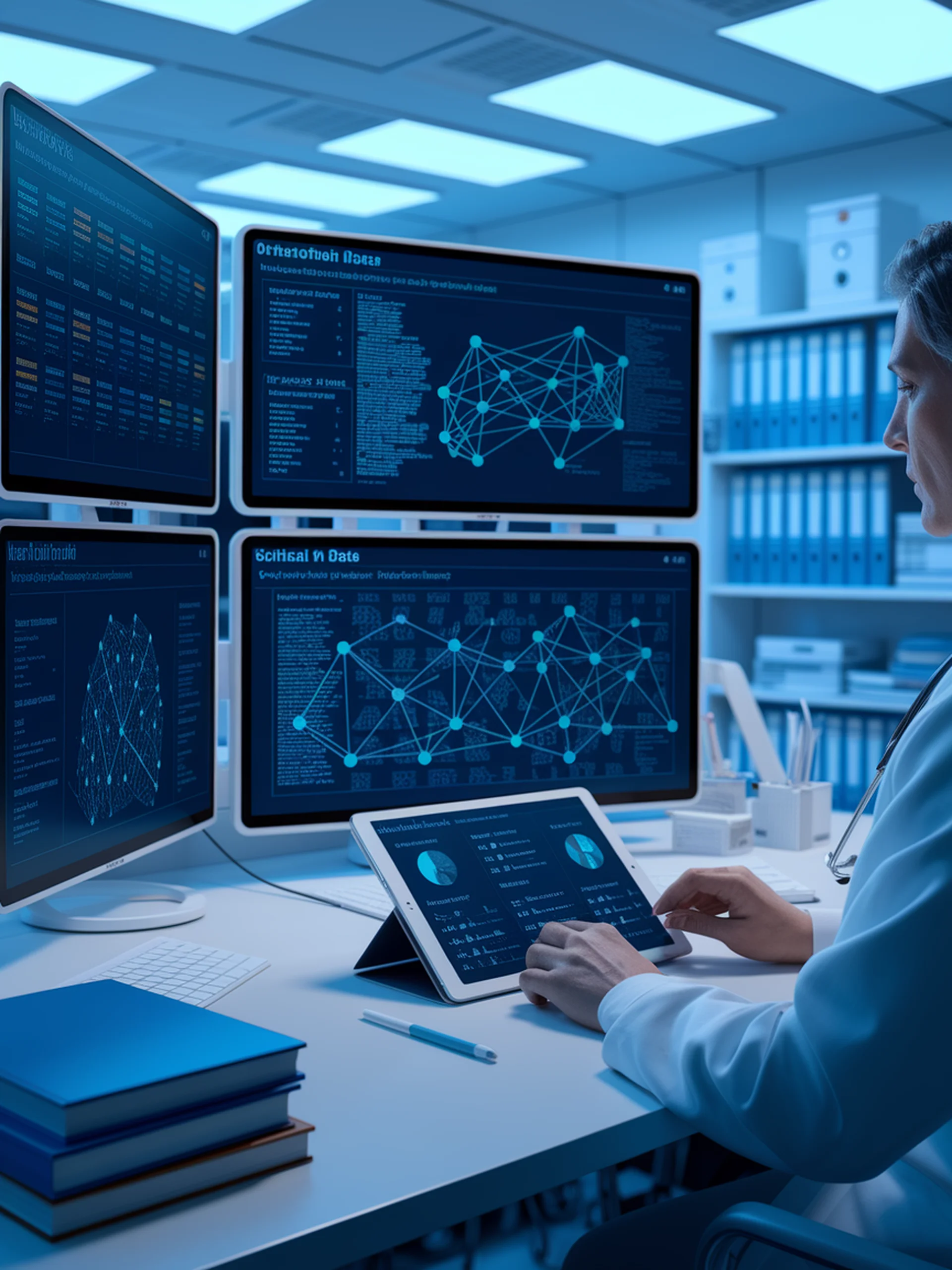
Unlocking Insights from Unstructured Text Data
Using AI to extract causal outcomes from clinical notes & case records
This research introduces a batch-adaptive annotation framework that helps extract and measure causal effects from unstructured text documentation in healthcare and social services.
- Converts complex text data (clinical notes, case records) into measurable outcomes for causal inference
- Employs a novel batch-adaptive approach that optimizes human annotation resources
- Addresses the critical challenge of measuring intervention effectiveness from narrative documentation
- Demonstrates practical applications in healthcare and social services settings
For medical professionals, this technology represents a breakthrough in utilizing existing clinical documentation to measure treatment effectiveness without additional data collection. The framework transforms qualitative notes into quantifiable outcomes that can inform evidence-based practice.
Batch-Adaptive Annotations for Causal Inference with Complex-Embedded Outcomes