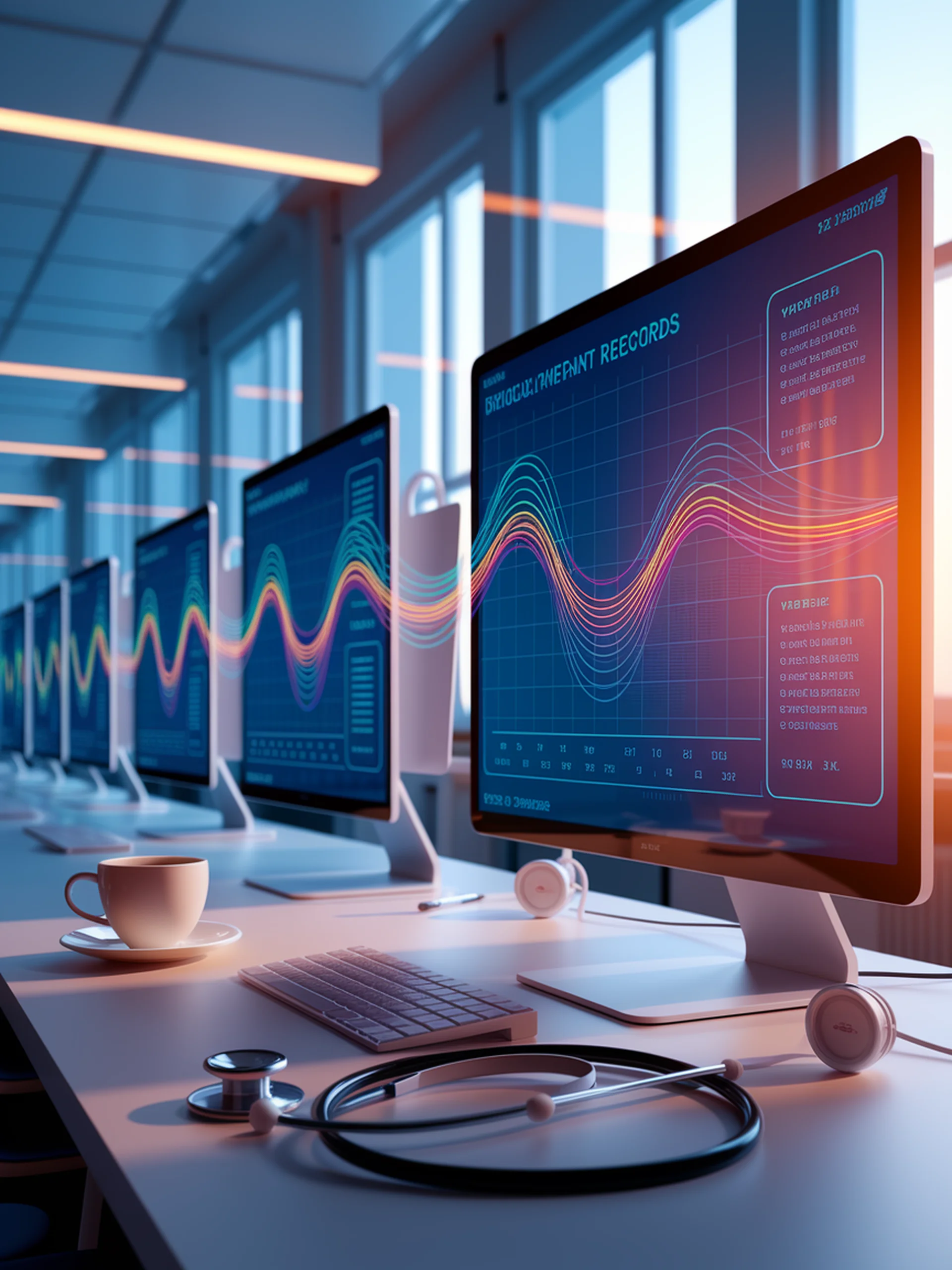
Temporal Intelligence for Medical Records
Enhancing LLMs' Ability to Reason Across Patient Visit Timelines
TIMER introduces a framework for evaluating and improving large language models' temporal reasoning capabilities when processing longitudinal clinical records.
- Addresses the unique challenge of reasoning across multiple patient visits and time frames in Electronic Health Records (EHRs)
- Creates specialized benchmarks and instruction tuning techniques for temporal medical data
- Evaluates LLM capabilities to understand complex patient histories and make time-dependent medical assessments
- Establishes new methods for enhancing medical AI systems' ability to process longitudinal health data
This research is crucial for advancing clinical decision support systems that can accurately interpret patient histories over time, potentially improving diagnostic accuracy and treatment planning in healthcare settings.
TIMER: Temporal Instruction Modeling and Evaluation for Longitudinal Clinical Records