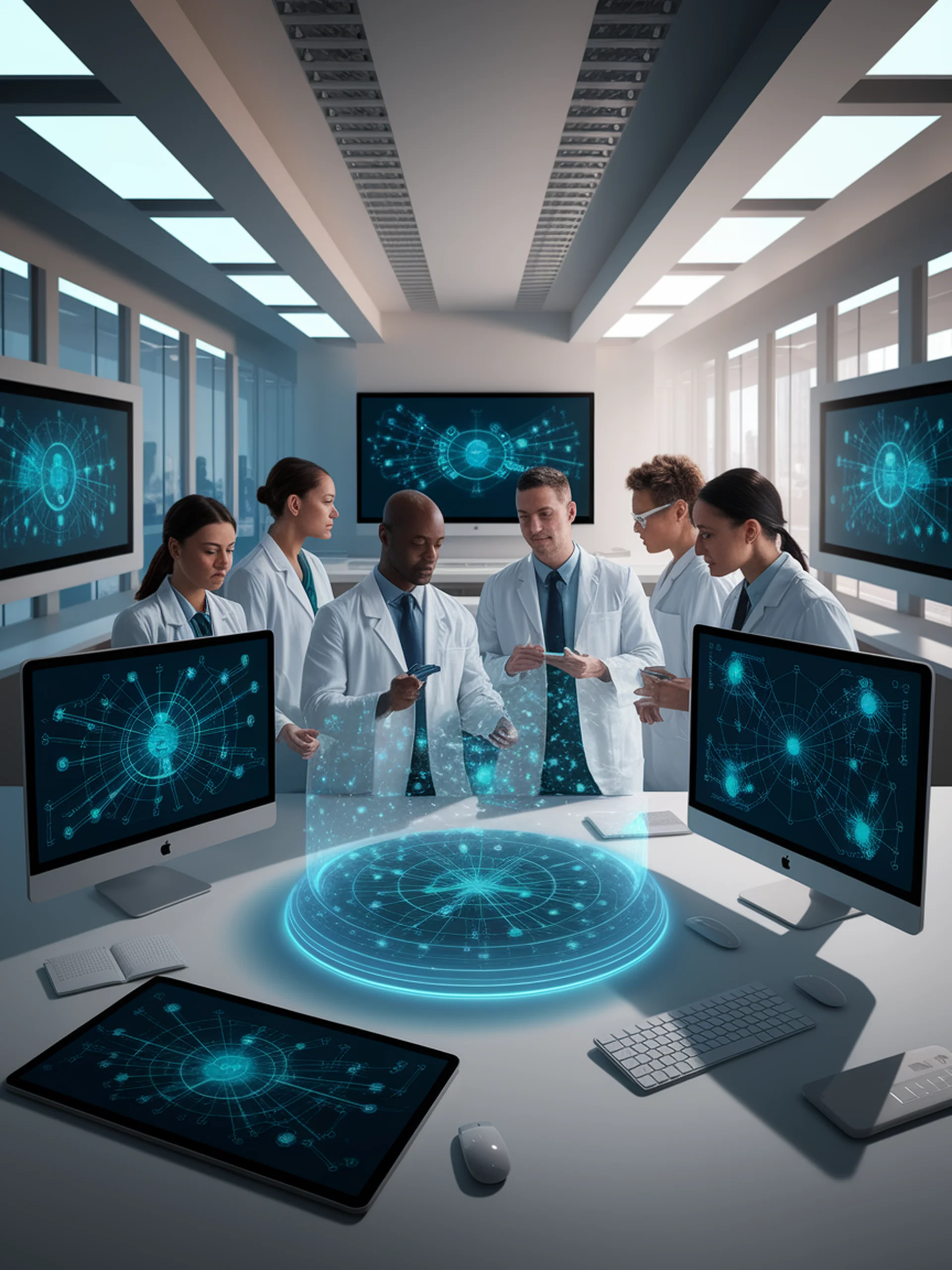
Fairness in AI: LLM-Powered Causal Discovery
Advancing fairness through active learning and dynamic scoring
This research introduces a novel framework using Large Language Models (LLMs) for causal discovery that prioritizes fairness considerations while managing computational complexity.
- Integrates active learning techniques to efficiently identify causal relationships
- Employs dynamic scoring mechanisms to reduce bias in causal inference
- Leverages metadata-based approaches for improved performance
- Addresses high computational demands of traditional causal discovery algorithms
Medical Impact: This framework enables healthcare researchers to identify fair and unbiased causal relationships in patient data, leading to more equitable treatment protocols and reducing disparities in medical outcomes across diverse populations.
Fairness-Driven LLM-based Causal Discovery with Active Learning and Dynamic Scoring