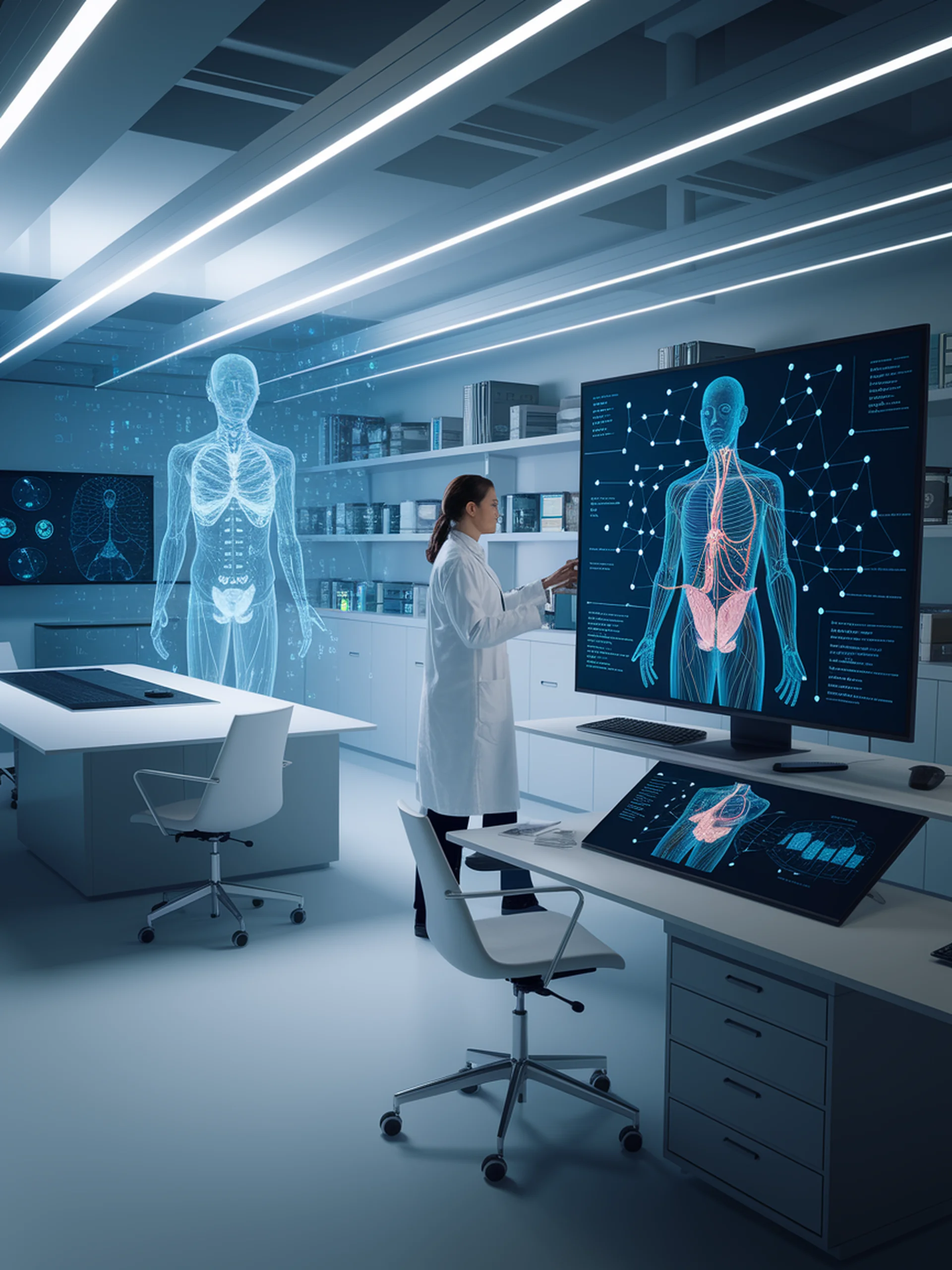
Leveraging LLMs for Causal Knowledge Extraction
Reimagining Expert Elicitation in Healthcare Decision Systems
This research explores how Large Language Models can replace or supplement human experts in building probabilistic causal models for healthcare applications.
- LLM-generated Bayesian networks were benchmarked against traditional statistical methods using healthcare datasets
- Results indicate that LLMs can effectively extract structured causal knowledge with proper prompting
- The approach offers a promising alternative to traditional expert elicitation, which is often time-consuming and expensive
- Particularly valuable for medical domains where expert knowledge is critical but difficult to obtain
This research matters for healthcare by potentially democratizing access to causal modeling tools, accelerating development of decision support systems, and lowering barriers to implementing evidence-based practices in clinical settings.
Can LLMs Assist Expert Elicitation for Probabilistic Causal Modeling?