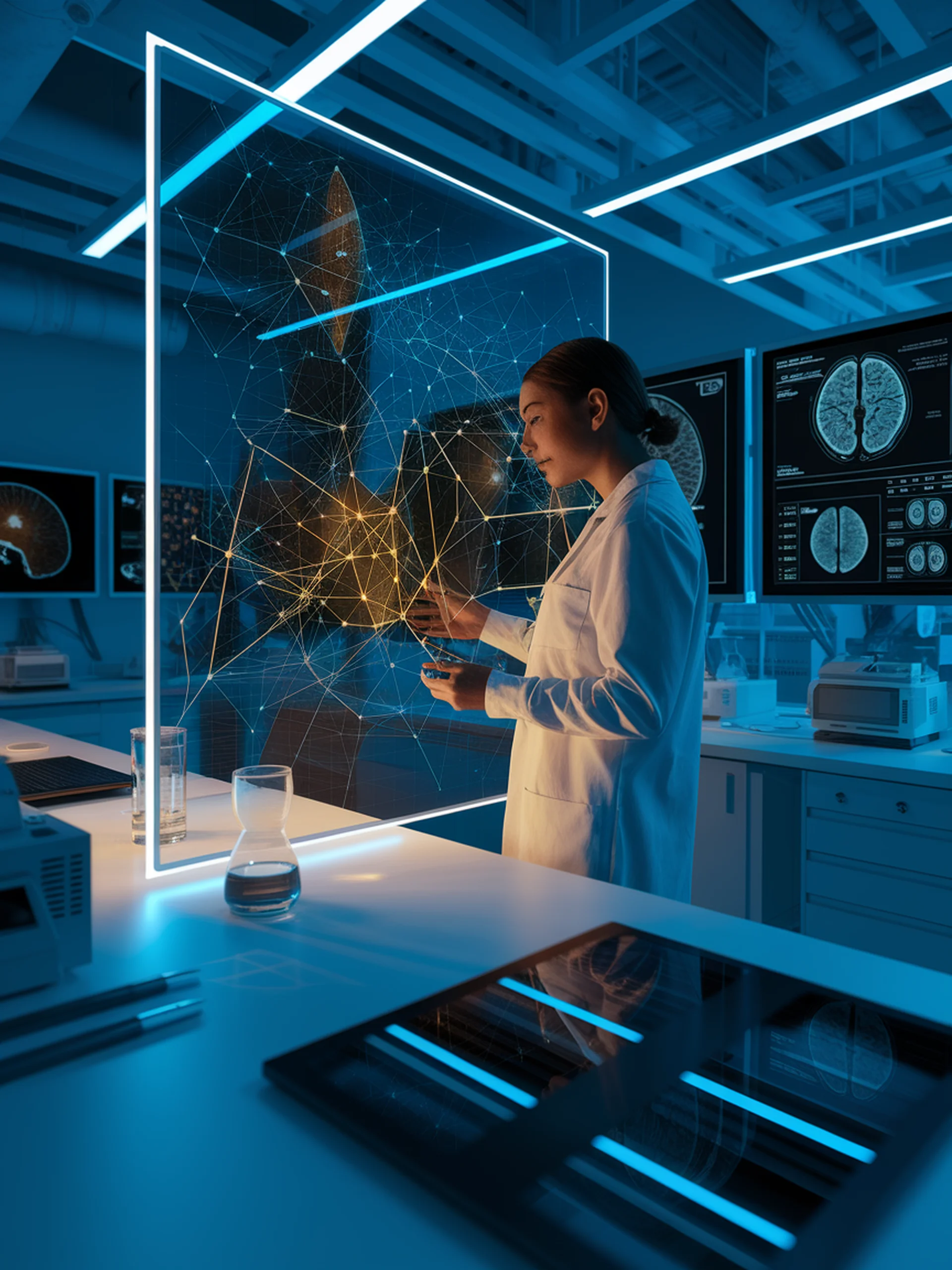
Enhancing Causal Discovery with LLMs
Exploring how language models can process observational data to identify causal relationships
This research investigates whether Large Language Models can effectively leverage observational data for causal discovery, potentially transforming how we identify cause-effect relationships in complex systems.
- Examines LLMs' capabilities beyond traditional statistical methods for causal discovery
- Proposes novel approaches for incorporating domain knowledge with data-driven insights
- Explores how LLMs can process and interpret observational data patterns
- Bridges the gap between AI language capabilities and traditional causal inference techniques
Security Implications: This research could enhance threat detection systems by identifying causal chains in security events, enabling more proactive vulnerability assessment and improving attribution of attack patterns through causal reasoning rather than mere correlation.
Can LLMs Leverage Observational Data? Towards Data-Driven Causal Discovery with LLMs