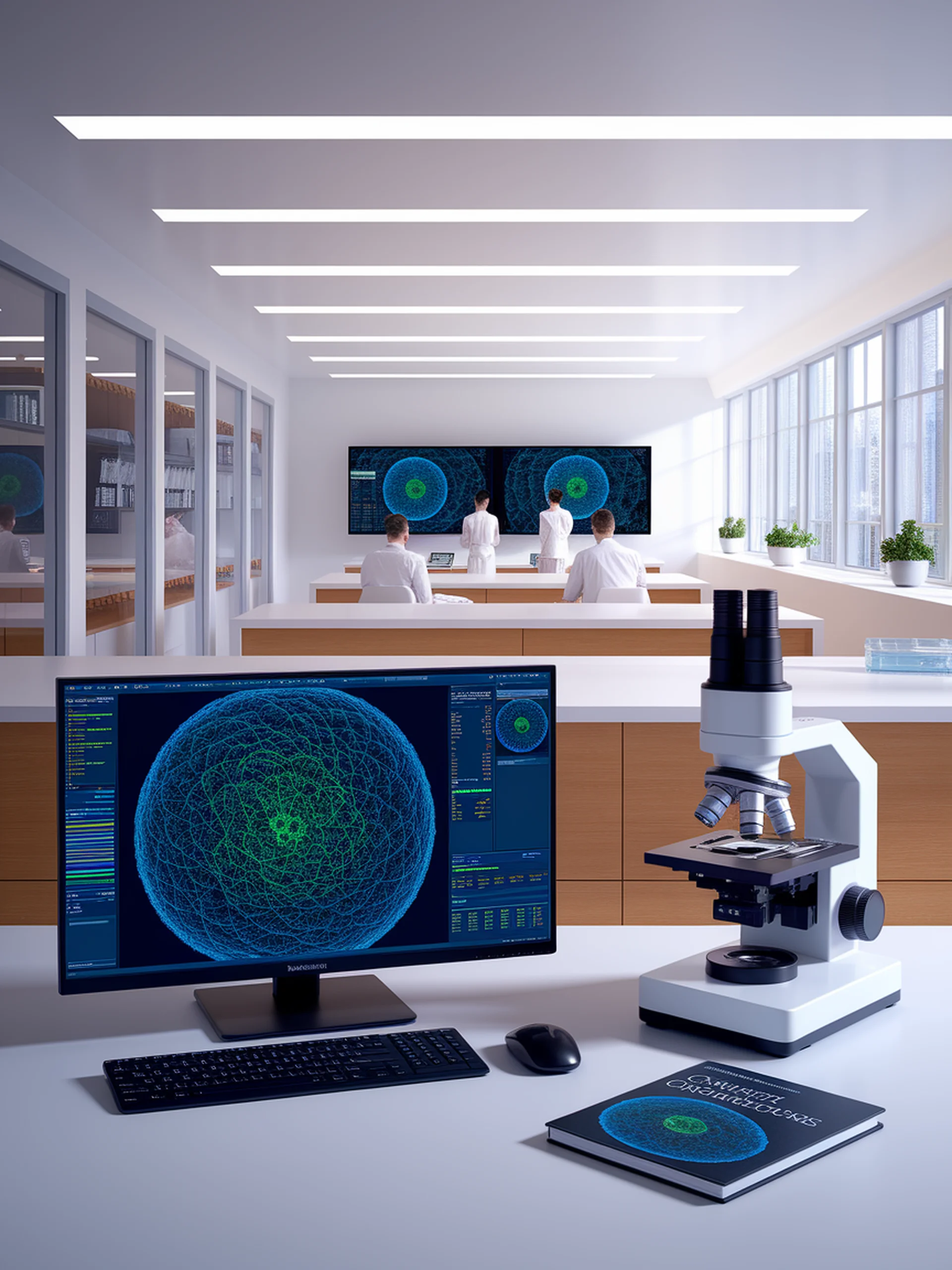
LLMs as Medical Rule Testers
Using AI to validate cancer registry data systems
This research showcases how Large Language Models can effectively perform differential testing on medical rule engines used in cancer registries.
- Demonstrates LLMs' ability to generate test cases for complex medical rule validation systems
- Achieves 89% accuracy in identifying inconsistencies in the GURI rule engine used by Norway's Cancer Registry
- Reveals both false positives and legitimate inconsistencies between medical rules and their implementations
- Establishes a new testing methodology that complements traditional testing approaches in healthcare IT
This approach matters because it can help ensure medical data validation systems operate correctly, potentially improving cancer registry data quality while reducing the need for manual testing by medical experts.
LLMs in the Heart of Differential Testing: A Case Study on a Medical Rule Engine