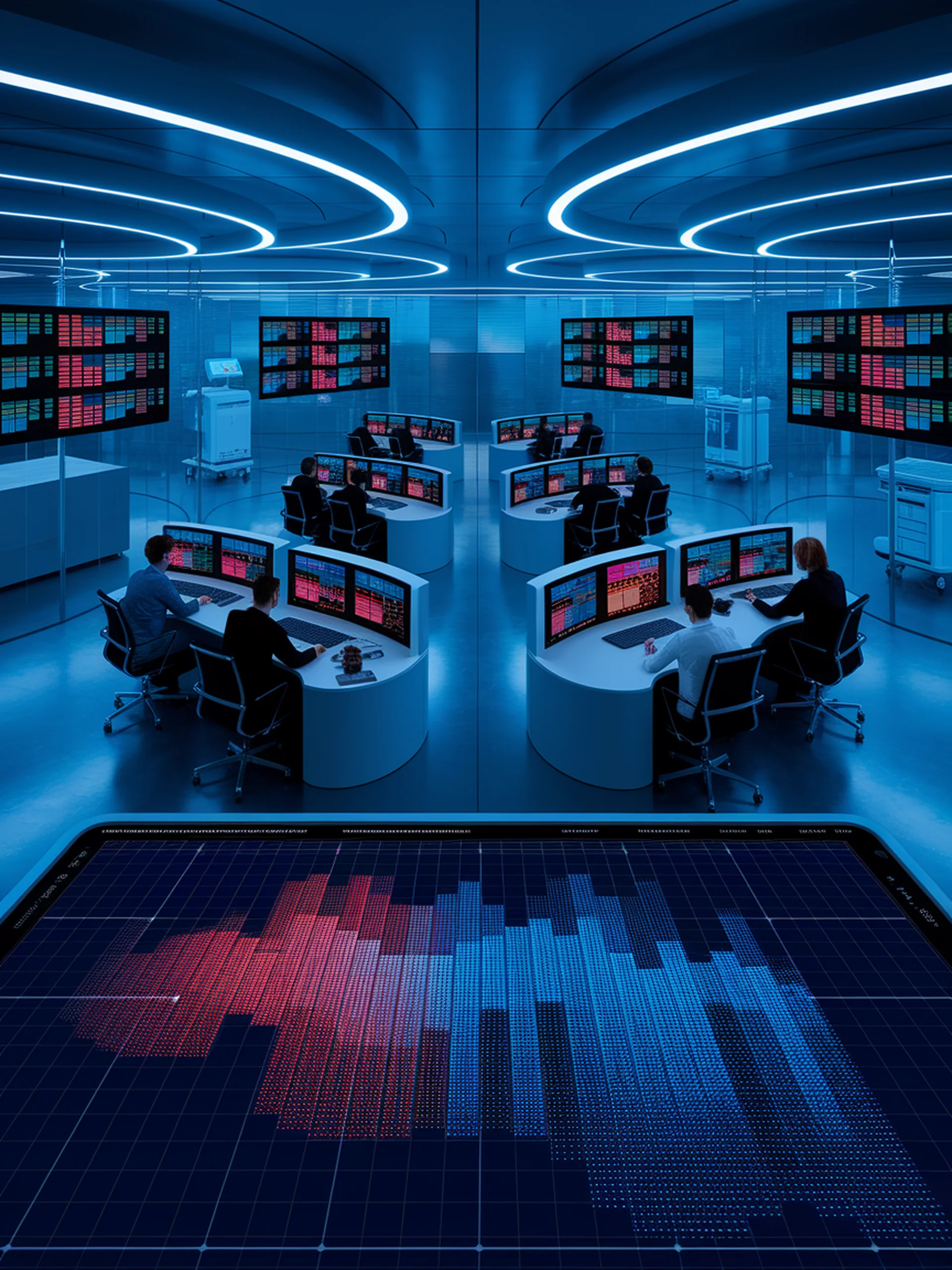
Smart OOD Detection Selection
Automating the choice of distribution shift detection models
MetaOOD introduces a novel framework for automatically selecting optimal out-of-distribution (OOD) detection models, critical for maintaining reliability in security-sensitive applications.
- Eliminates manual trial-and-error by using meta-features to match detection methods to specific tasks
- Achieves 90%+ accuracy in selecting optimal OOD detection models across diverse datasets
- Provides significant performance improvements over random selection approaches
- Creates a powerful meta-learning framework applicable across security, medical, and engineering domains
Security impact: By automatically selecting the best methods to detect distribution shifts and anomalous inputs, MetaOOD significantly enhances protection against potential security threats in online transactions, autonomous systems, and other critical applications.