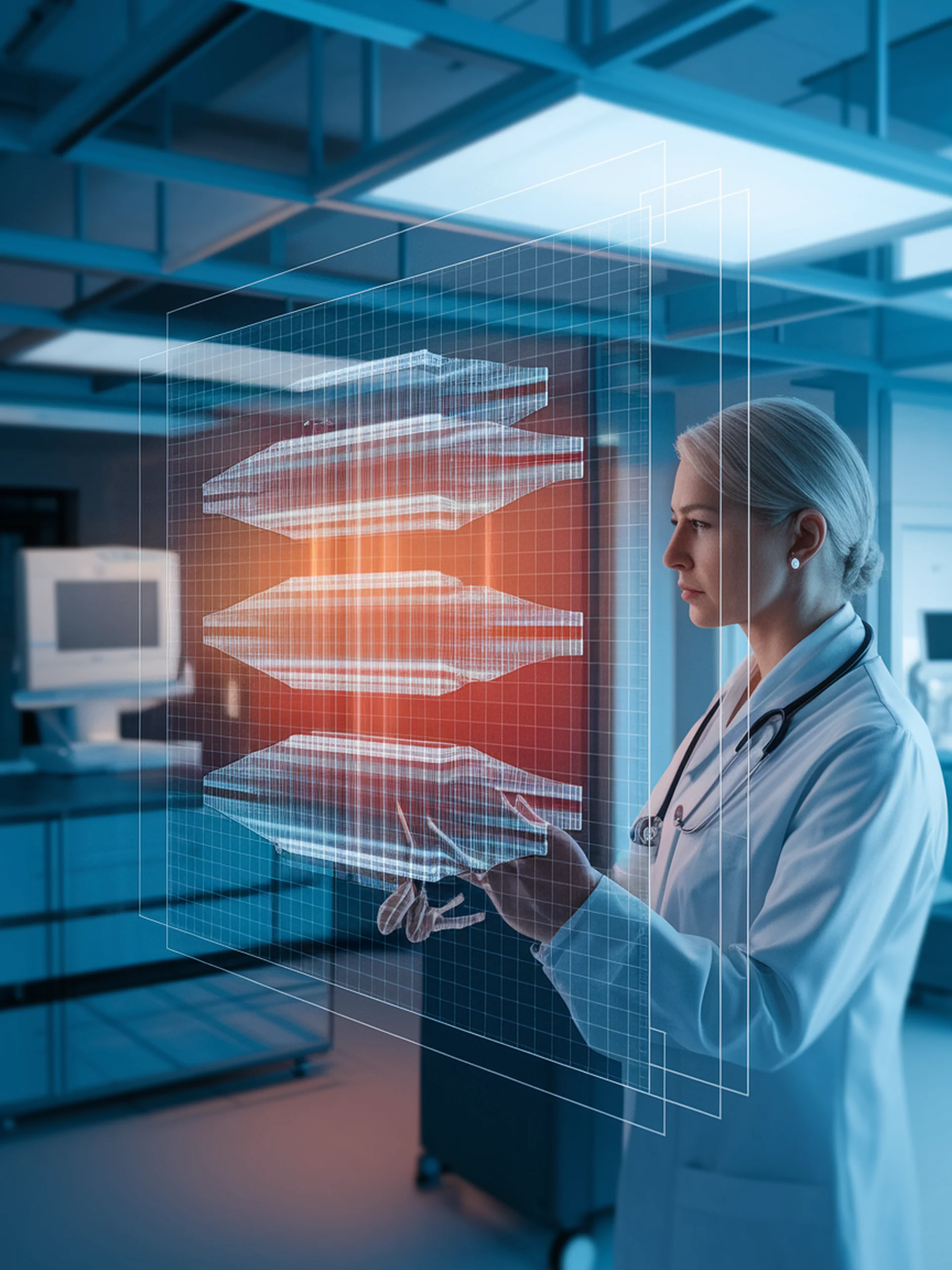
Smart LLM Cascades That Know When to Step Back
Reducing costs while managing risk through strategic abstention
This research introduces a cost-effective approach for deploying LLMs in risk-sensitive domains by using smaller models for most queries and allowing abstention when confidence is low.
Key findings:
- Early abstention in LLM cascades reduces costs by up to 31% while maintaining performance
- System intelligently routes queries between smaller and larger models based on confidence
- Particularly valuable in medical contexts where the cost of errors is high
- Achieves strong results on medical benchmarks including MedMCQA
Why it matters for healthcare: This approach allows medical AI systems to acknowledge uncertainty rather than making potentially harmful errors, balancing cost savings with patient safety in clinical decision support.