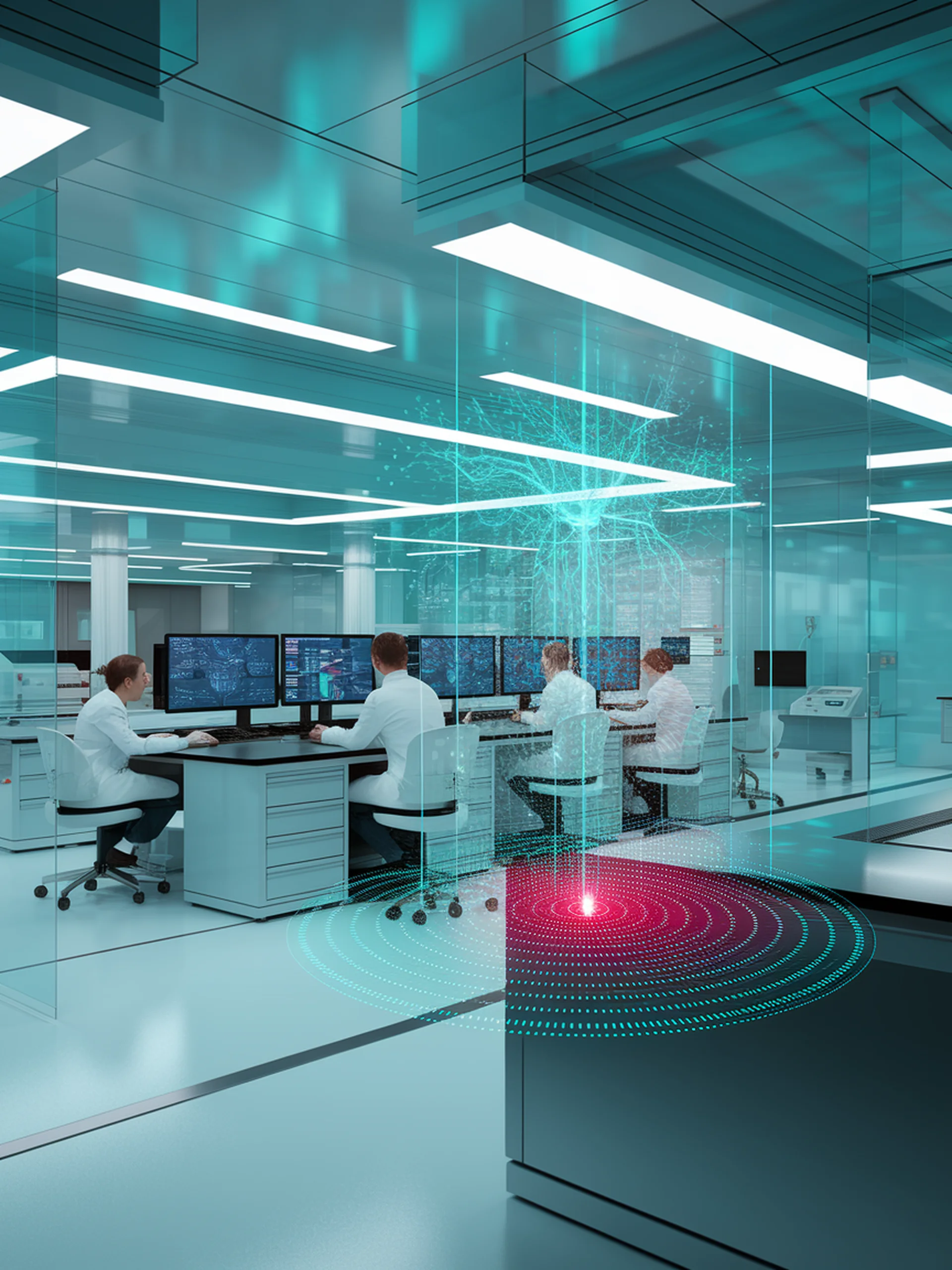
Controlling What LLMs Learn
A framework for removing unwanted features from classification models
This research introduces a novel self-regularization technique that enables controllable classification by identifying and removing unwanted features from LLM embeddings.
- Creates interpretable latent spaces that expose which features influence classification decisions
- Allows selective removal of sensitive or irrelevant features while maintaining performance
- Demonstrates improved results in toxic content detection and other security applications
- Provides a path toward more compliant and generalizable AI classification systems
For security professionals, this approach offers a practical solution to regulatory compliance challenges by enabling precise control over which features influence classification decisions in sensitive applications.
Self-Regularization with Latent Space Explanations for Controllable LLM-based Classification