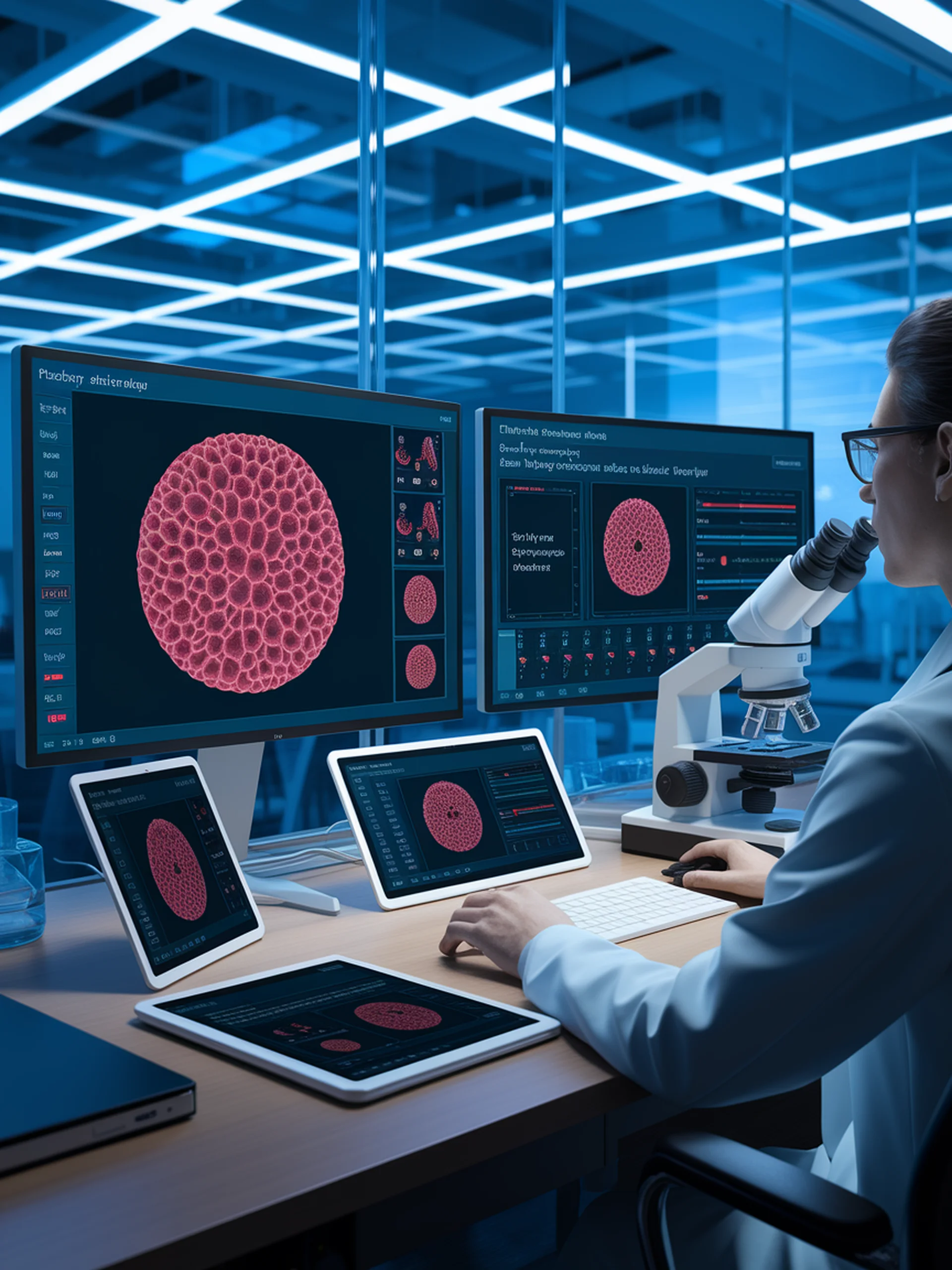
LLMs Revolutionizing Cancer Diagnostics
Automated extraction of critical cancer insights from pathology reports
This research evaluates how Large Language Models can effectively analyze unstructured pathology reports to extract vital cancer information with clinical accuracy.
- Benchmarks multiple LLM families (GPT, Mistral, Llama) on cancer type identification, staging, and prognosis assessment
- Demonstrates the potential for automating critical medical data extraction from complex pathology reports
- Quantifies effectiveness of various prompt engineering techniques for medical applications
- Shows promise for reducing manual review burden while maintaining diagnostic accuracy
This advancement matters because it could significantly accelerate cancer diagnosis workflows, reduce human error, and enable more consistent analysis across healthcare systems while addressing the challenge of extracting structured insights from unstructured medical text.
Original Paper: Cancer Type, Stage and Prognosis Assessment from Pathology Reports using LLMs