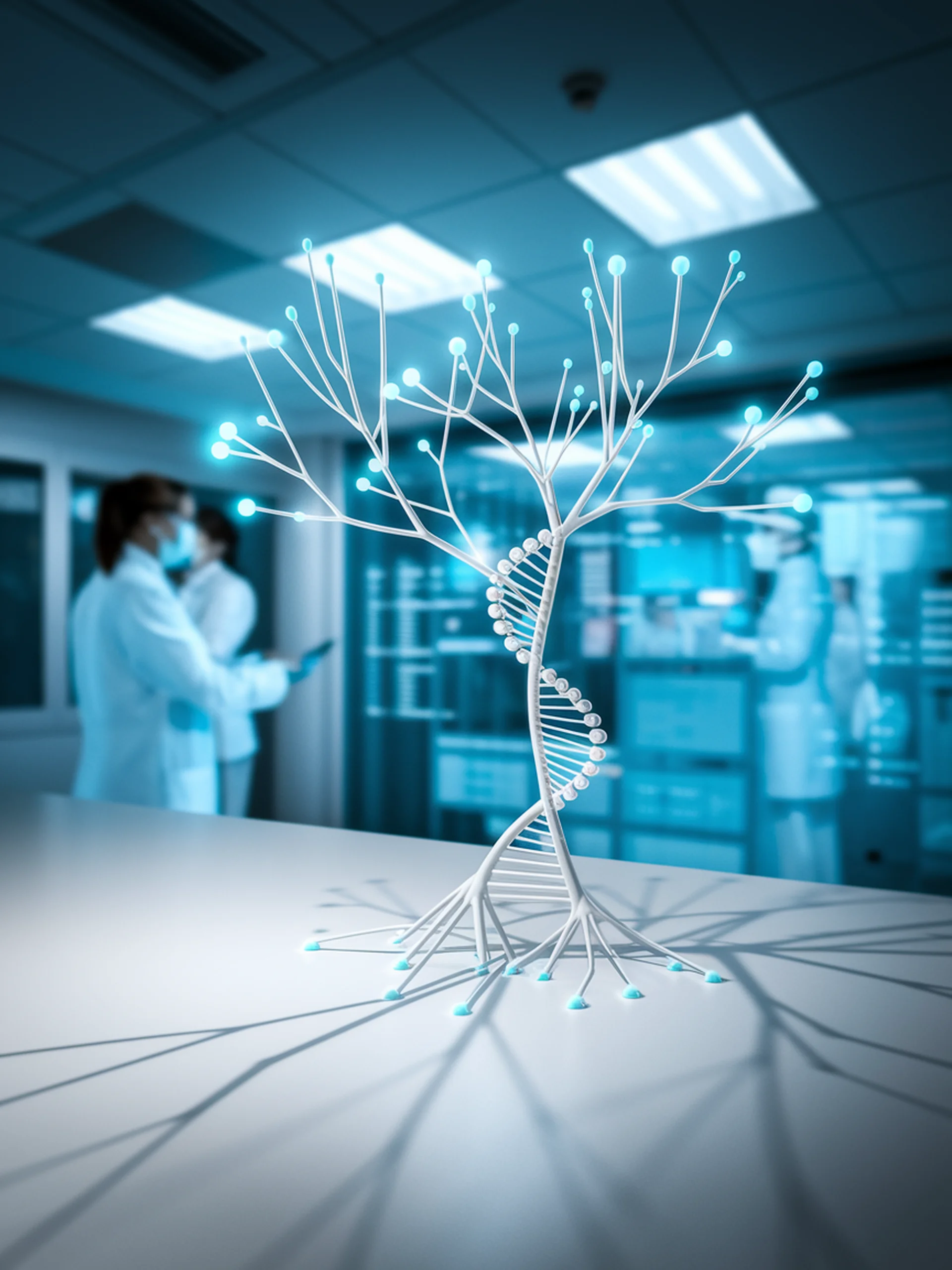
Evolving Better Decision Trees with LLMs
A semantic approach to optimizing interpretable models
This research introduces a novel evolutionary optimization method that uses large language models to create more effective decision trees while maintaining interpretability.
- Addresses limitations of traditional tree induction methods including greedy algorithms and computationally expensive optimization approaches
- Leverages LLMs' semantic understanding to guide the evolutionary process
- Creates decision trees that balance predictive performance with interpretability
- Particularly valuable for domains requiring transparent decision-making like healthcare
For medical applications, this approach could significantly improve diagnostic and prognostic models where clinicians need to understand and trust the decision path, potentially leading to better patient outcomes through more accurate and explainable AI-assisted medical decisions.
Decision Tree Induction Through LLMs via Semantically-Aware Evolution