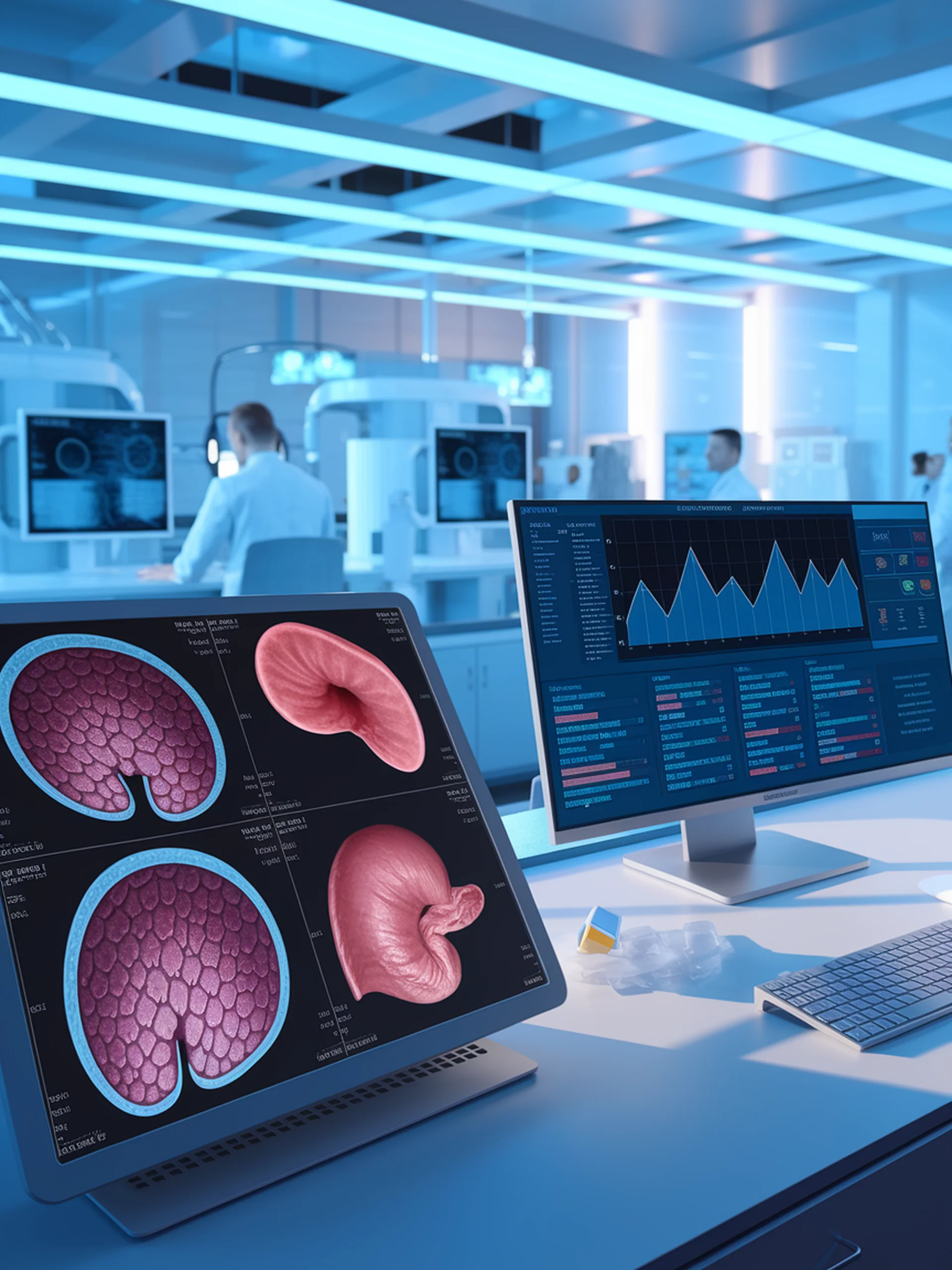
Enhancing Cancer Staging with AI
How Retrieval-Augmented Generation Improves LLM Accuracy in Medical Diagnosis
This study demonstrates that Retrieval-Augmented Generation (RAG) significantly improves Large Language Models' performance in pancreatic cancer staging by providing access to reliable external knowledge.
- RAG-enhanced models (NotebookLM) achieved superior accuracy in staging pancreatic cancer compared to standard LLMs
- Models with RAG showed 32.4% higher accuracy in T-staging and 27.0% higher accuracy in overall staging
- RAG capabilities reduced hallucinations and improved clinical reliability in complex medical assessments
- Findings suggest RAG is essential for medical applications where precision and factual accuracy are critical
This research matters for healthcare by offering a pathway to more reliable AI-assisted diagnosis tools that maintain accuracy while providing explainable results, potentially improving clinical decision-making in oncology.