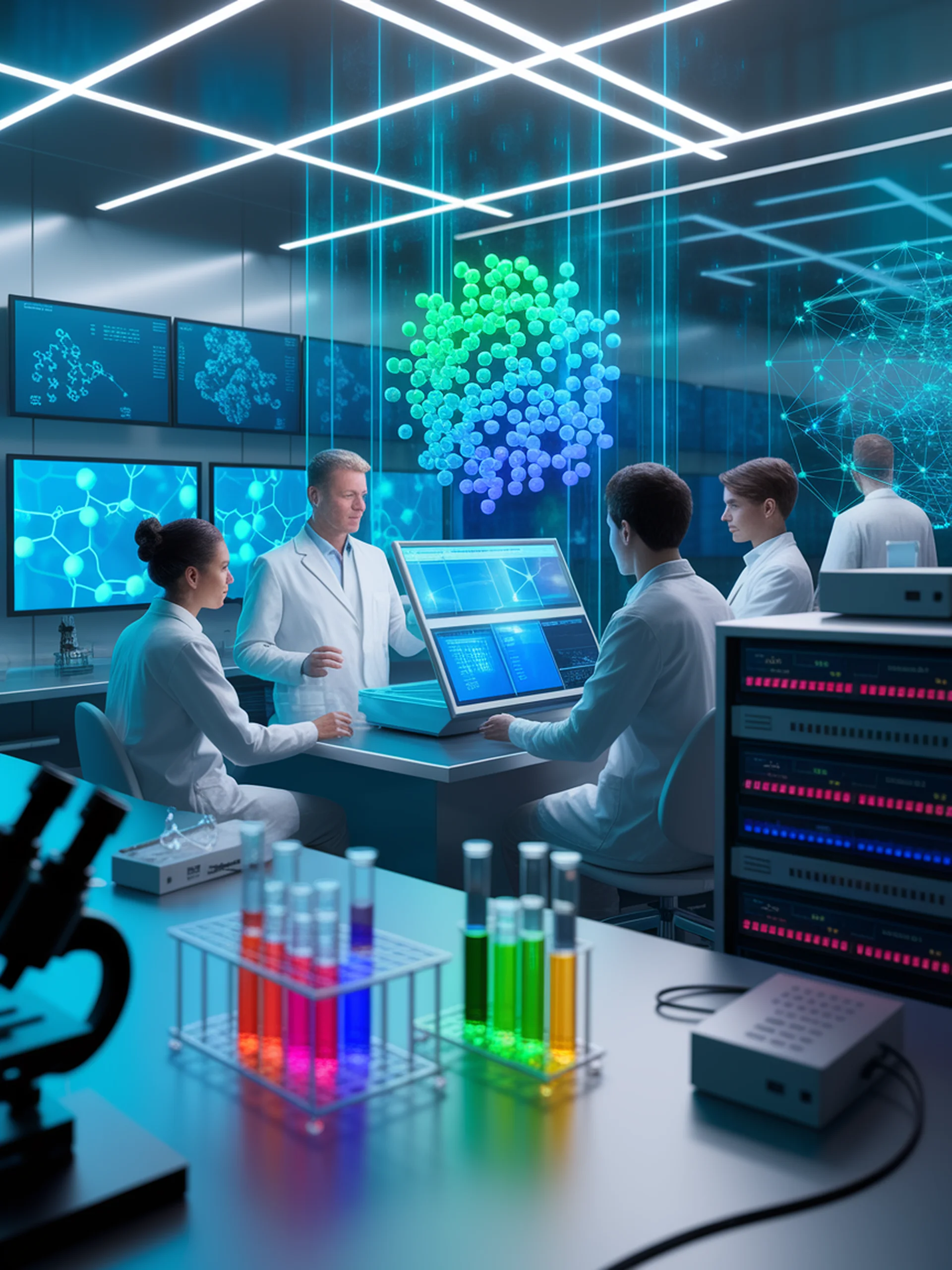
Optimizing AI for Drug Discovery
Refining Reinforcement Learning Approaches for Chemical Language Models
This research enhances reinforcement learning (RL) techniques for chemical language models to accelerate drug design and discovery processes.
- Examines REINFORCE algorithm components including experience replay, hill-climbing, and variance-reducing baselines
- Evaluates different RL approaches for efficient exploration of vast chemical spaces
- Provides practical best practices for implementing RL in pharmaceutical research
- Demonstrates improved molecular optimization techniques for drug candidates
This work matters because it could significantly reduce time and costs in pharmaceutical development by efficiently identifying promising drug compounds while navigating complex chemical spaces.