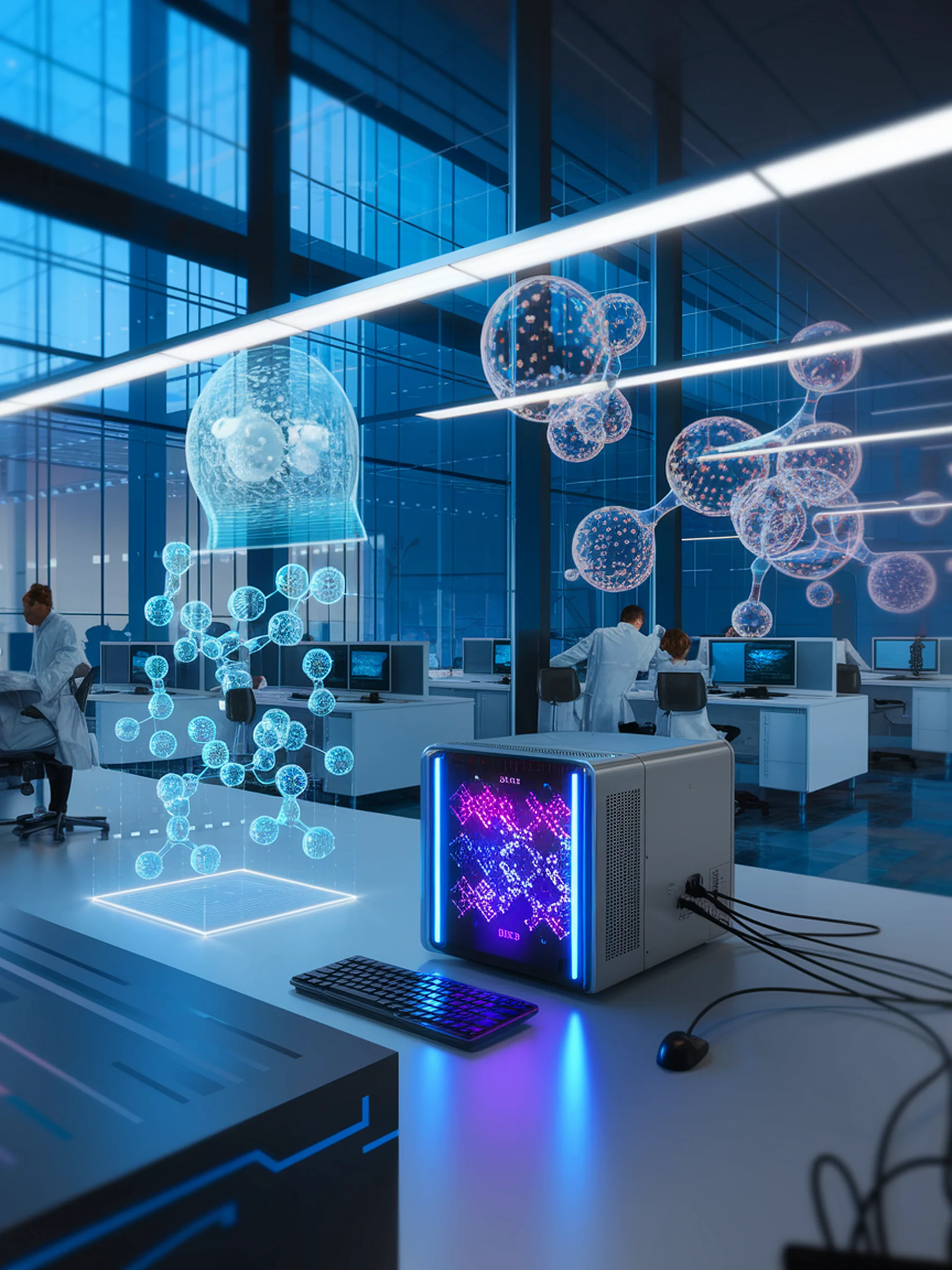
Quantum-Enhanced Transformer Models
Optimizing molecular generation through quantized self-attention
This research introduces a hybrid transformer architecture that uses quantum computing principles to reduce computational overhead while maintaining effectiveness for molecular design.
- Integrates quantized self-attention mechanisms to capture complex molecular relationships
- Successfully generates molecules with target physicochemical properties using the QM9 dataset
- Demonstrates improved computational efficiency compared to classical transformer models
- Creates a pathway for more resource-efficient AI models in computational chemistry
This innovation significantly advances the field of computational biology by enabling more efficient design of novel molecules for drug discovery and materials science, potentially accelerating pharmaceutical development pipelines.