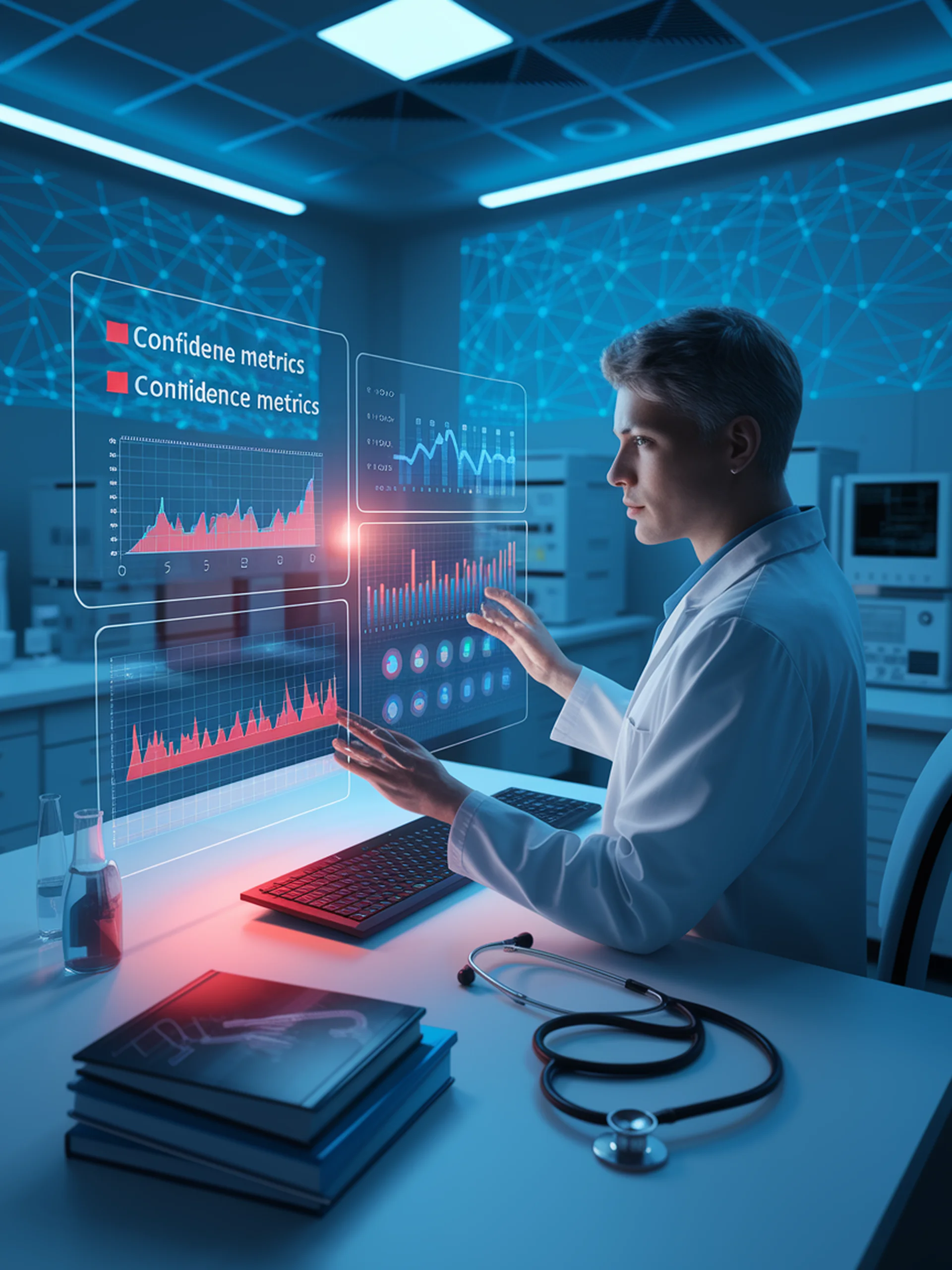
Improving LLM Confidence Evaluation
A new benchmark for accurately assessing when AI systems should trust their own outputs
This research introduces MCQA-Eval, a novel framework for evaluating confidence estimation methods in Large Language Models using gold-standard correctness labels.
- Creates a benchmark of 3,000 multiple-choice questions across various domains
- Eliminates noise and bias in traditional evaluation methods
- Enables more accurate comparison of confidence estimation techniques
- Reveals significant gaps between current confidence methods and ideal performance
Why it matters for healthcare: In medical applications, LLMs must accurately assess when their outputs are reliable to prevent potentially harmful decisions. MCQA-Eval provides a rigorous way to evaluate these confidence systems before deployment in critical healthcare settings.
MCQA-Eval: Efficient Confidence Evaluation in NLG with Gold-Standard Correctness Labels