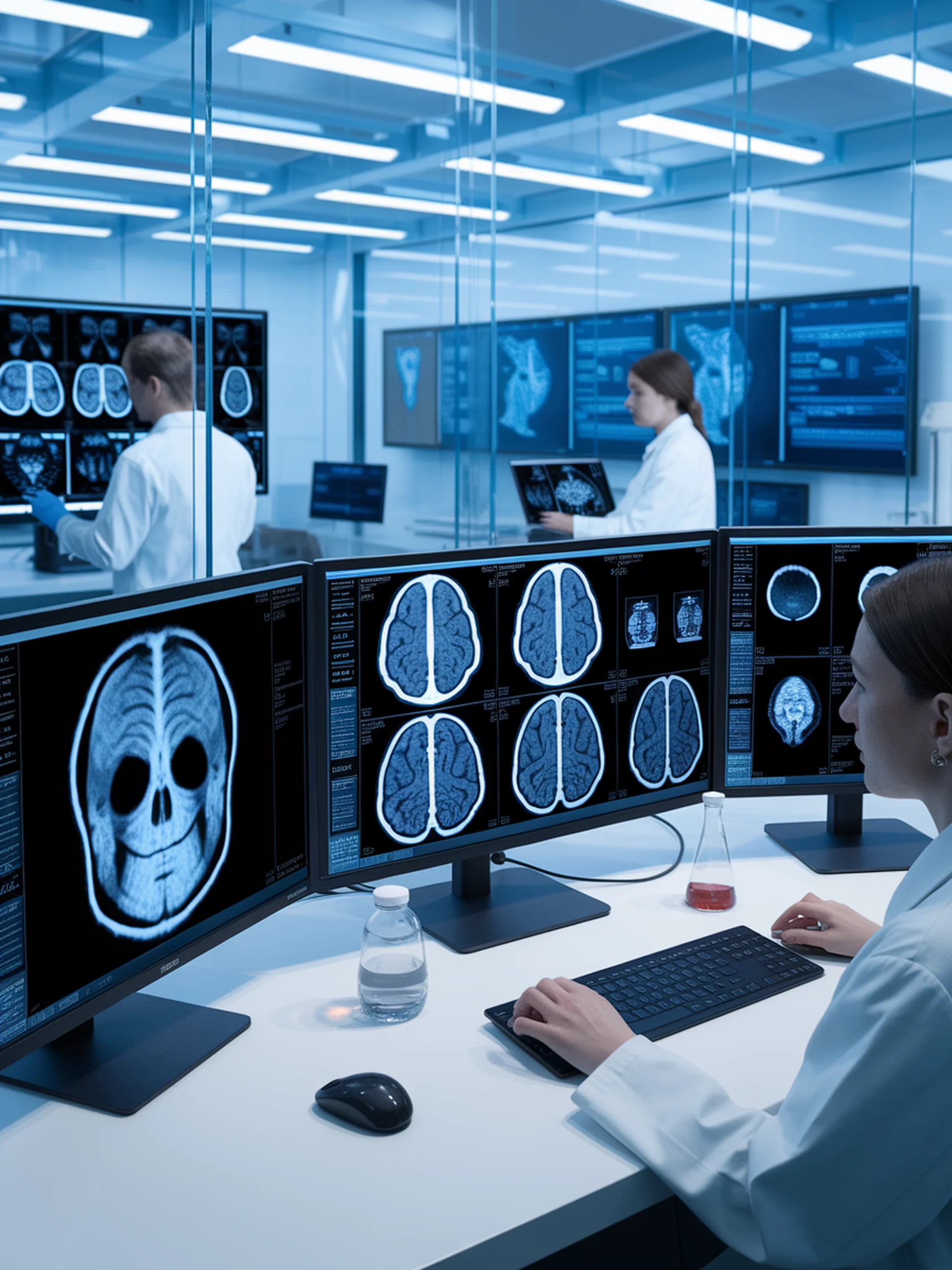
Combating Hallucinations in Medical AI
A systematic benchmark for evaluating and mitigating medical LVLM hallucinations
MedHEval introduces the first comprehensive benchmark for evaluating and mitigating hallucinations in Medical Large Vision-Language Models (Med-LVLMs).
- Systematically categorizes hallucination root causes in medical vision-language tasks
- Evaluates 6 mitigation strategies across 800 instances from 8 diverse medical datasets
- Reveals that retrieval augmentation and rejection sampling provide the most effective mitigation
- Demonstrates that model confidence scores correlate poorly with hallucination likelihood
This research is crucial for healthcare applications where AI hallucinations could lead to patient harm, establishing essential guardrails for safer clinical AI deployment.