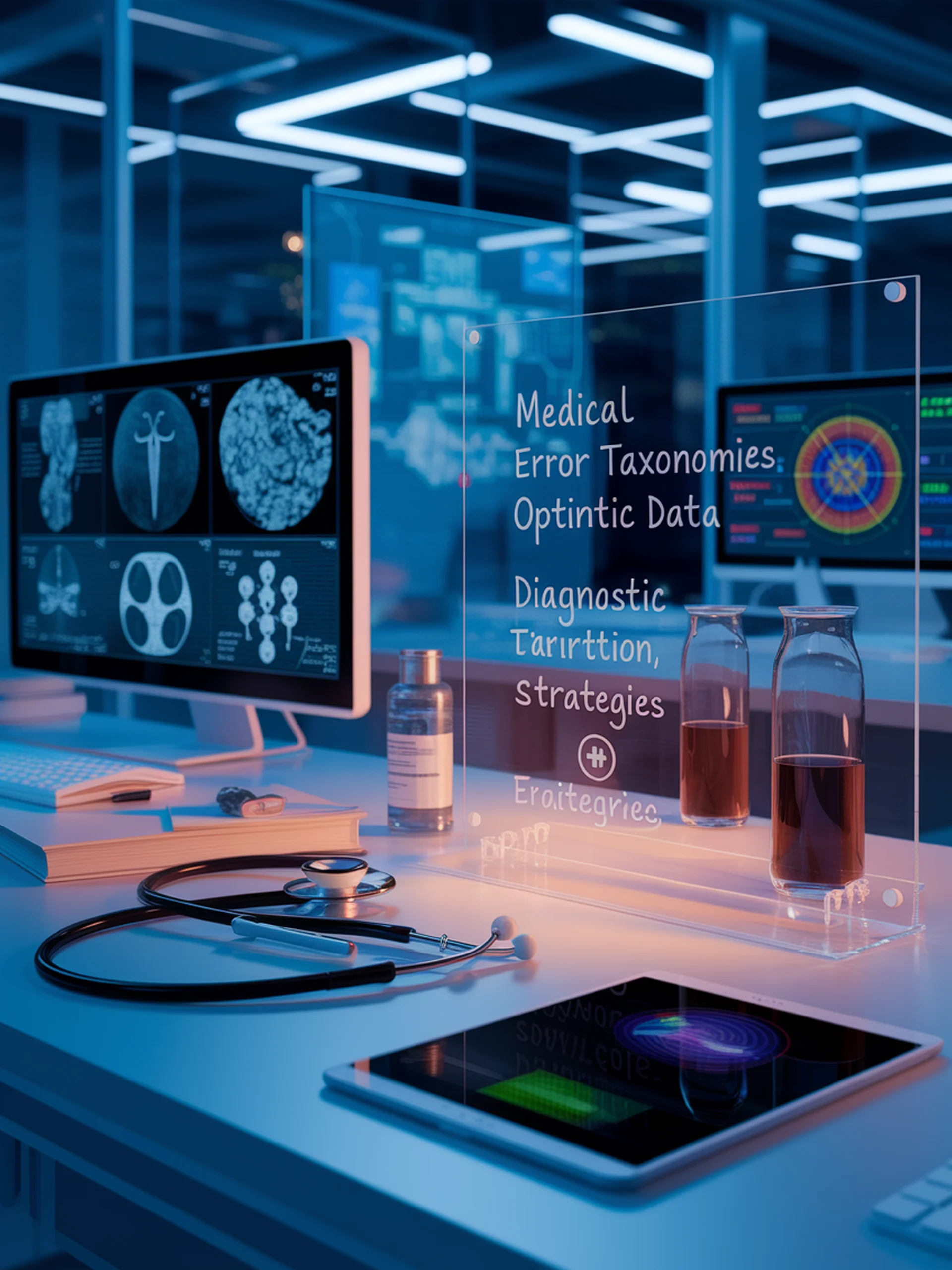
Improving Medical AI Accuracy
A Framework for Understanding and Fixing LLM Errors in Healthcare
This research introduces a comprehensive error taxonomy for medical large language models, providing a systematic approach to identifying and addressing performance gaps.
- Analyzes top 10 models on MedBench, categorizing errors into 8 distinct types including omissions and hallucinations
- Proposes hierarchical optimization strategies to systematically improve model performance
- Reveals specific patterns of failure in medical knowledge recall and clinical reasoning
- Enables more targeted improvements for safer deployment in healthcare settings
This framework matters for healthcare AI because it moves beyond simple accuracy metrics to address the specific types of errors that could impact patient care, enabling more trustworthy medical AI systems.