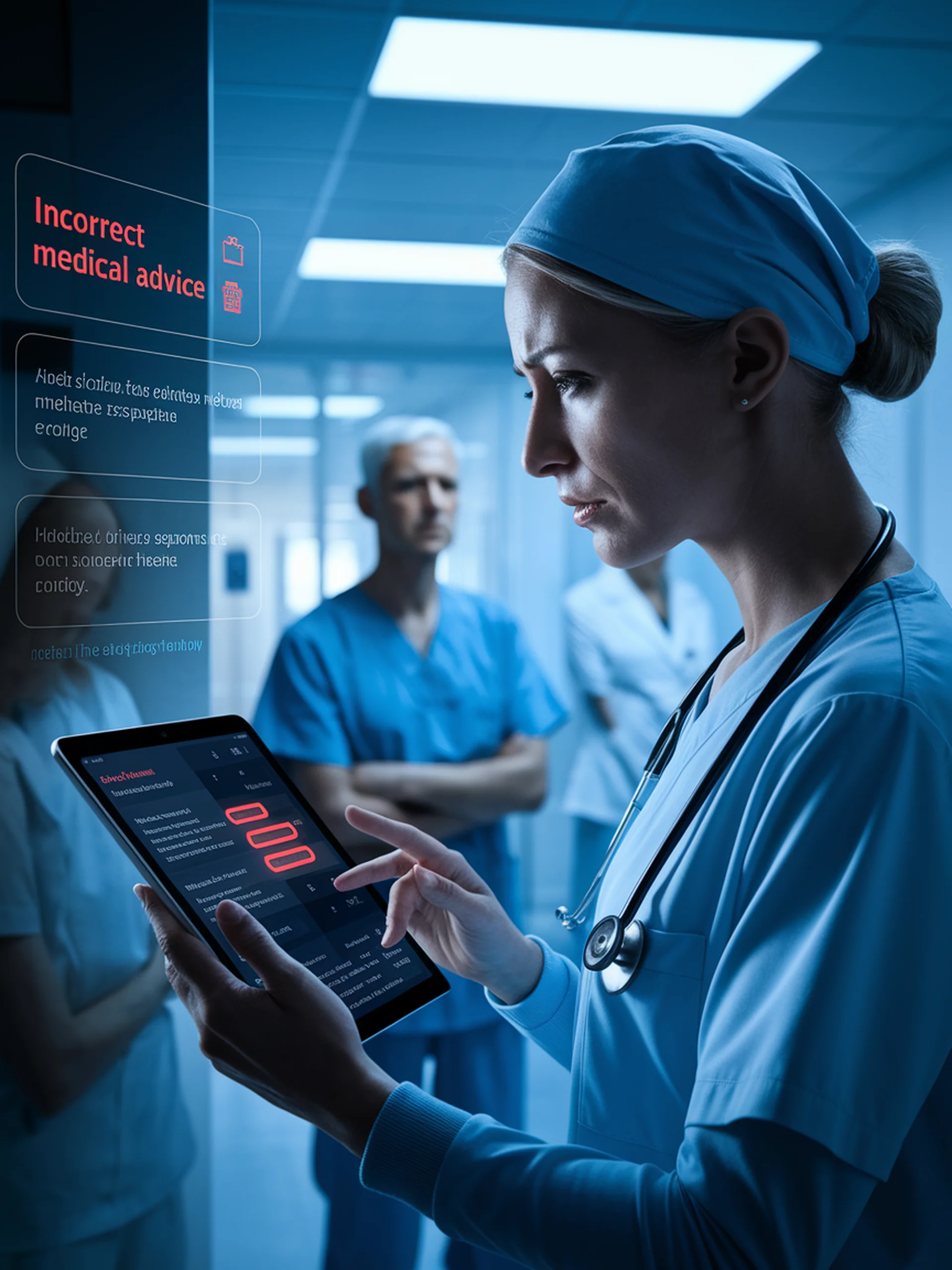
When LLMs Get Medical Advice Wrong
How user inputs compromise AI reliability in healthcare
This study reveals how user-driven factors significantly impact the accuracy and reliability of large language models in medical contexts.
- Misinformation framing and perceived source authority can lead models to generate incorrect medical information
- Omission of key clinical details substantially degrades diagnostic accuracy
- The way questions are phrased and the completeness of clinical information are critical factors in model performance
Why it matters: As healthcare increasingly adopts AI systems, understanding these vulnerabilities is essential for patient safety, clinician training, and developing safeguards against potential misdiagnosis or misinformation propagation.
Susceptibility of Large Language Models to User-Driven Factors in Medical Queries