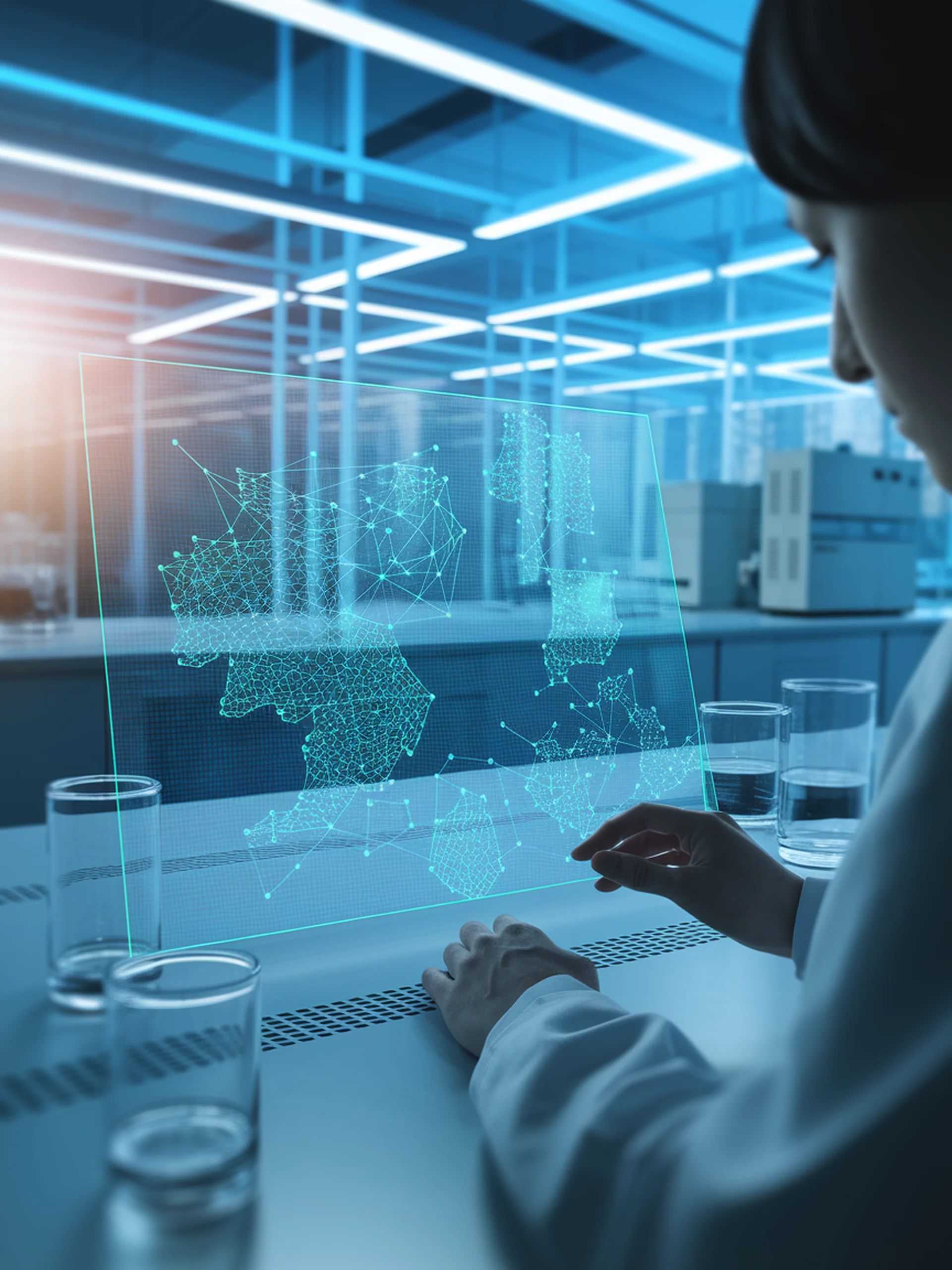
Unlocking Hidden Patterns in Biological Data
Exploring Sparse Autoencoders for Interpretable Biological Insights
This research demonstrates how sparse autoencoders (SAEs) can effectively decompose complex biological data into interpretable features, revealing hidden variables and relationships.
- SAEs can uncover both observable variables and hidden upstream variables in superposition
- The approach provides a powerful tool for interpreting high-dimensional biological datasets where underlying variables are unknown
- Results show SAEs can successfully extract meaningful biological signals from complex data
This work offers significant potential for advancing biological research by providing interpretable representations of complex data, enabling scientists to identify key biological processes and patterns that might otherwise remain hidden.
Can sparse autoencoders make sense of latent representations?