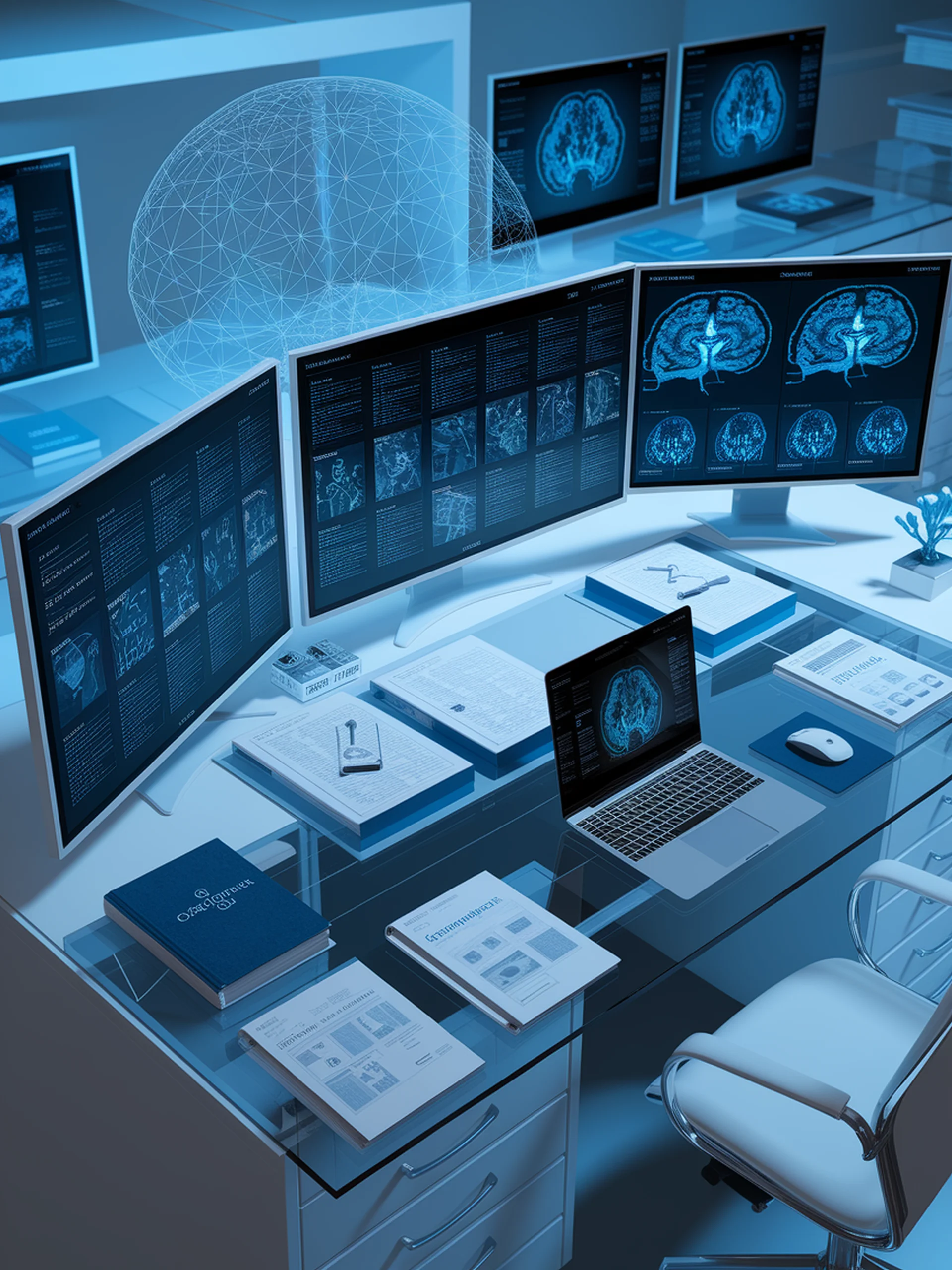
Optimizing RAG Systems
How context size and model selection impact retrieval-augmented generation
This research systematically explores the critical components of Retrieval-Augmented Generation (RAG) systems, focusing on context size optimization and model selection.
- Context size matters: The study identifies optimal context window sizes for different domains and LLMs
- Model-specific performance: Different base LLMs exhibit varying retrieval efficiency and answer quality
- Domain considerations: Performance variations between encyclopedic and specialized domains (like biomedical) highlight the importance of domain-specific tuning
- Practical implications: Findings help organizations optimize RAG systems for cost-efficiency and accuracy
For medical applications, this research is particularly valuable as it demonstrates how to build more reliable information retrieval systems that can access and accurately utilize biomedical knowledge, potentially improving clinical decision support tools.
On the Influence of Context Size and Model Choice in Retrieval-Augmented Generation Systems