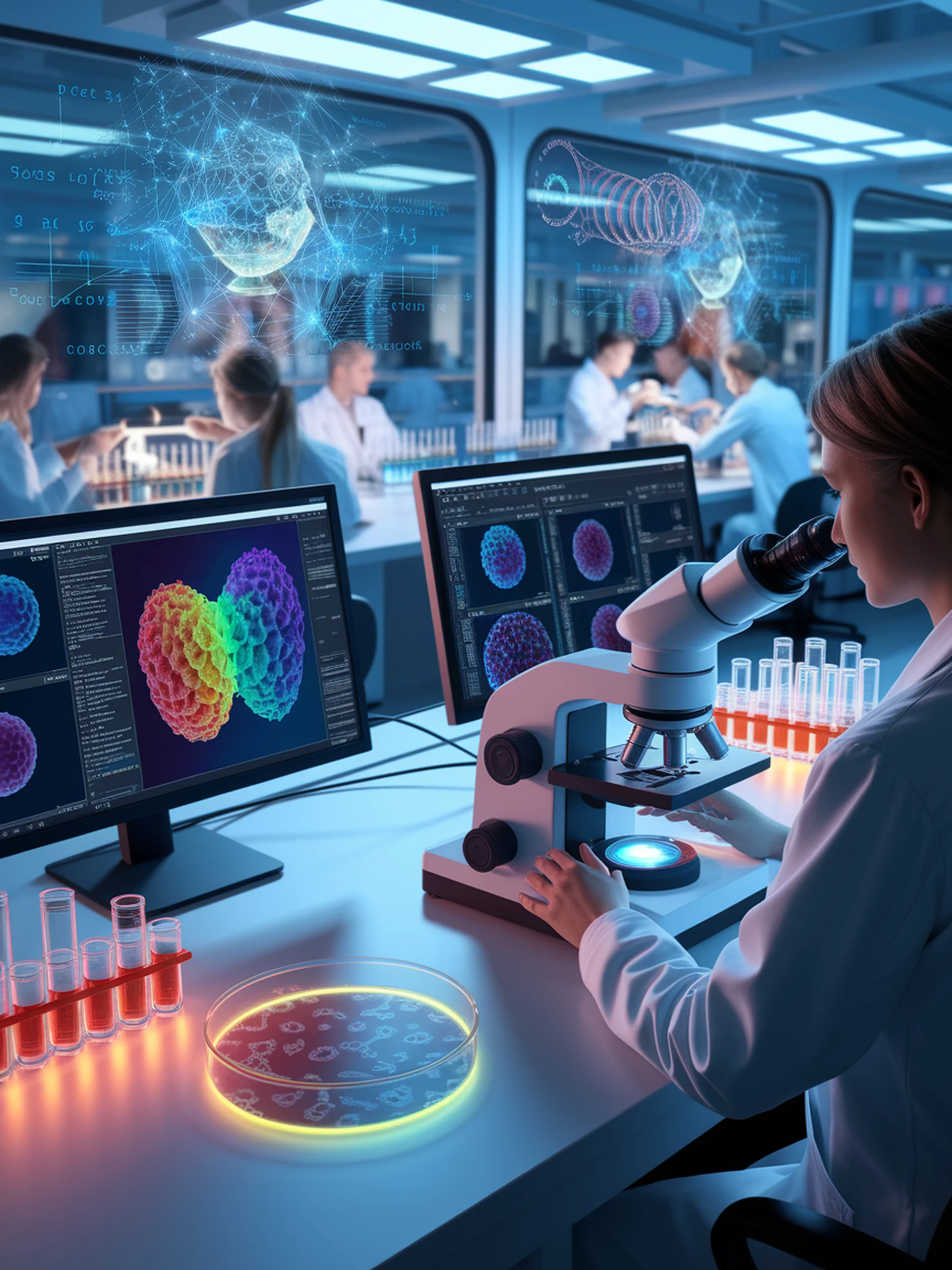
AI-Powered Biological Discovery
Leveraging Language Models to Guide Perturbation Experiments
This research introduces a novel framework that combines language models with biological experimentation to efficiently explore perturbation spaces and extract meaningful insights.
- Integrates semantic biological knowledge into machine learning pipelines
- Aligns computational objectives with downstream biological analyses
- Reduces experimental costs while maintaining scientific rigor
- Enables more targeted and efficient exploration of complex biomolecular systems
Why It Matters: This approach has the potential to dramatically accelerate biological discovery by making high-content perturbation experiments more accessible and interpretable, leading to faster insights in drug discovery and disease understanding.
Contextualizing biological perturbation experiments through language