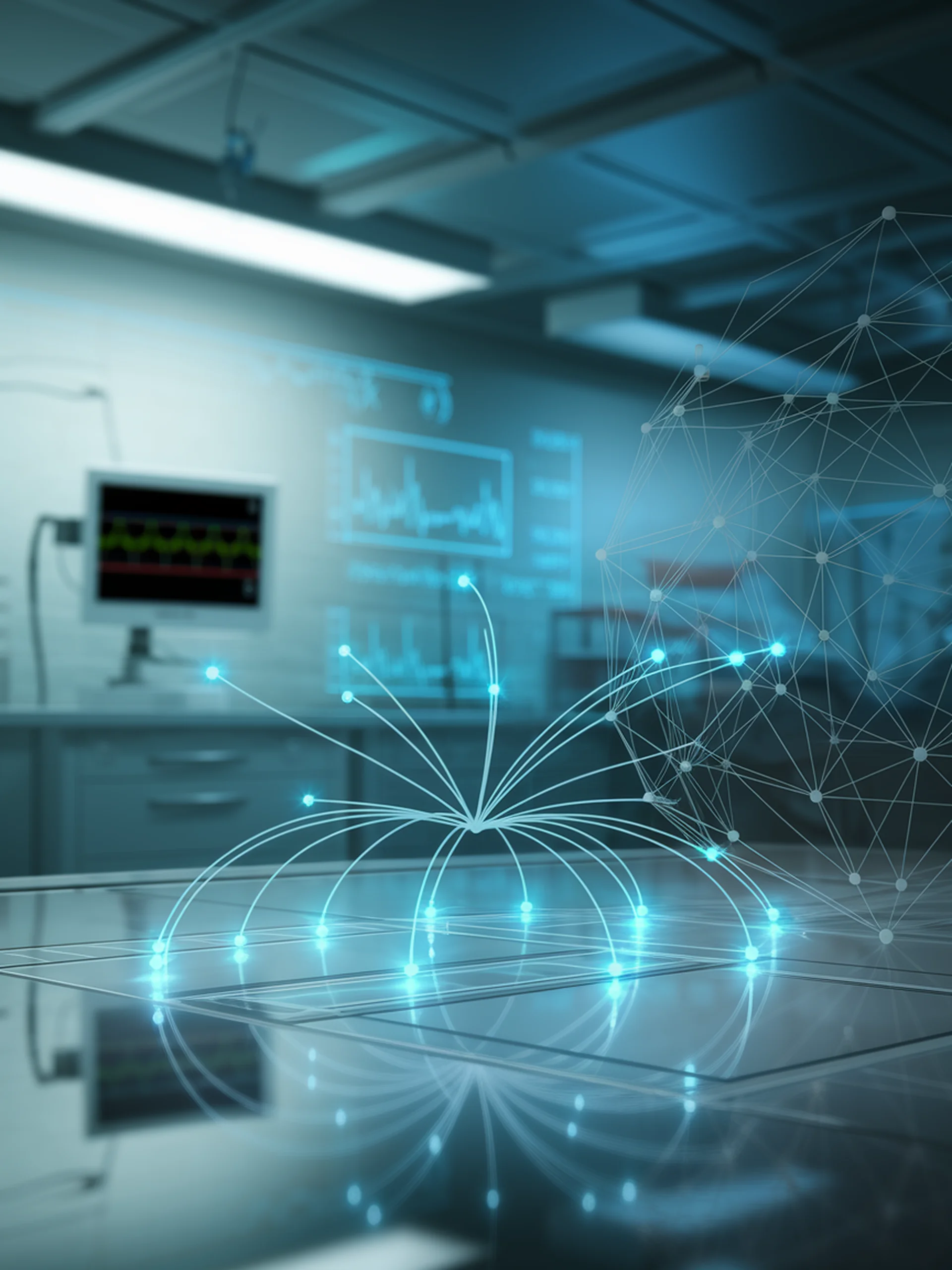
Simple Neural Networks Outperform Complex Models
Less complexity, better performance in time series forecasting
This research reveals that simple feedforward neural networks (SFNNs) can match or exceed the performance of more complex models like Transformers and GNNs for time series forecasting tasks.
- SFNNs achieve state-of-the-art results with significantly less computational complexity
- Complex models designed for language processing don't necessarily translate to better time series forecasting
- Simplicity offers advantages in training efficiency and interpretability
- Applicable across multiple domains including healthcare for medical time series prediction
For healthcare applications, this finding enables more efficient processing of patient monitoring data, clinical time series, and predictive healthcare analytics without sacrificing accuracy.
Simple Feedfoward Neural Networks are Almost All You Need for Time Series Forecasting