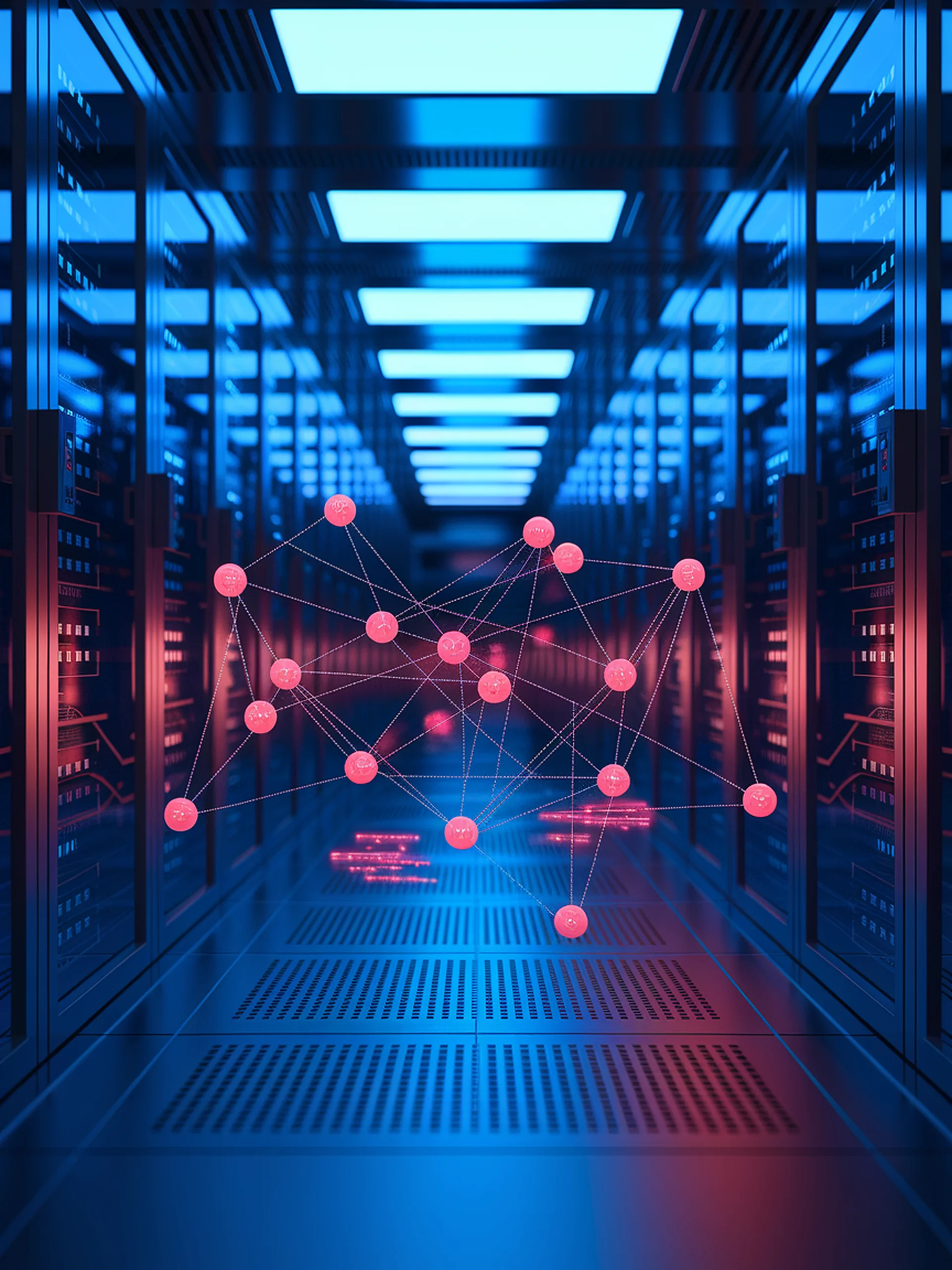
Privacy Vulnerabilities in Federated Learning
How malicious actors can extract sensitive data from language models
This research reveals critical security vulnerabilities allowing attackers to steal private data in federated learning environments for language models.
- FLTrojan attack demonstrated successful extraction of sensitive information (including healthcare data and credentials)
- Exposes how attackers can use selective weight tampering to target specific information
- Shows these attacks can bypass existing privacy protection mechanisms
- Highlights the urgent need for robust security measures in federated learning deployments
This work is significant for security professionals as it identifies previously unknown exploitable weaknesses in a widely adopted privacy-preserving machine learning approach.