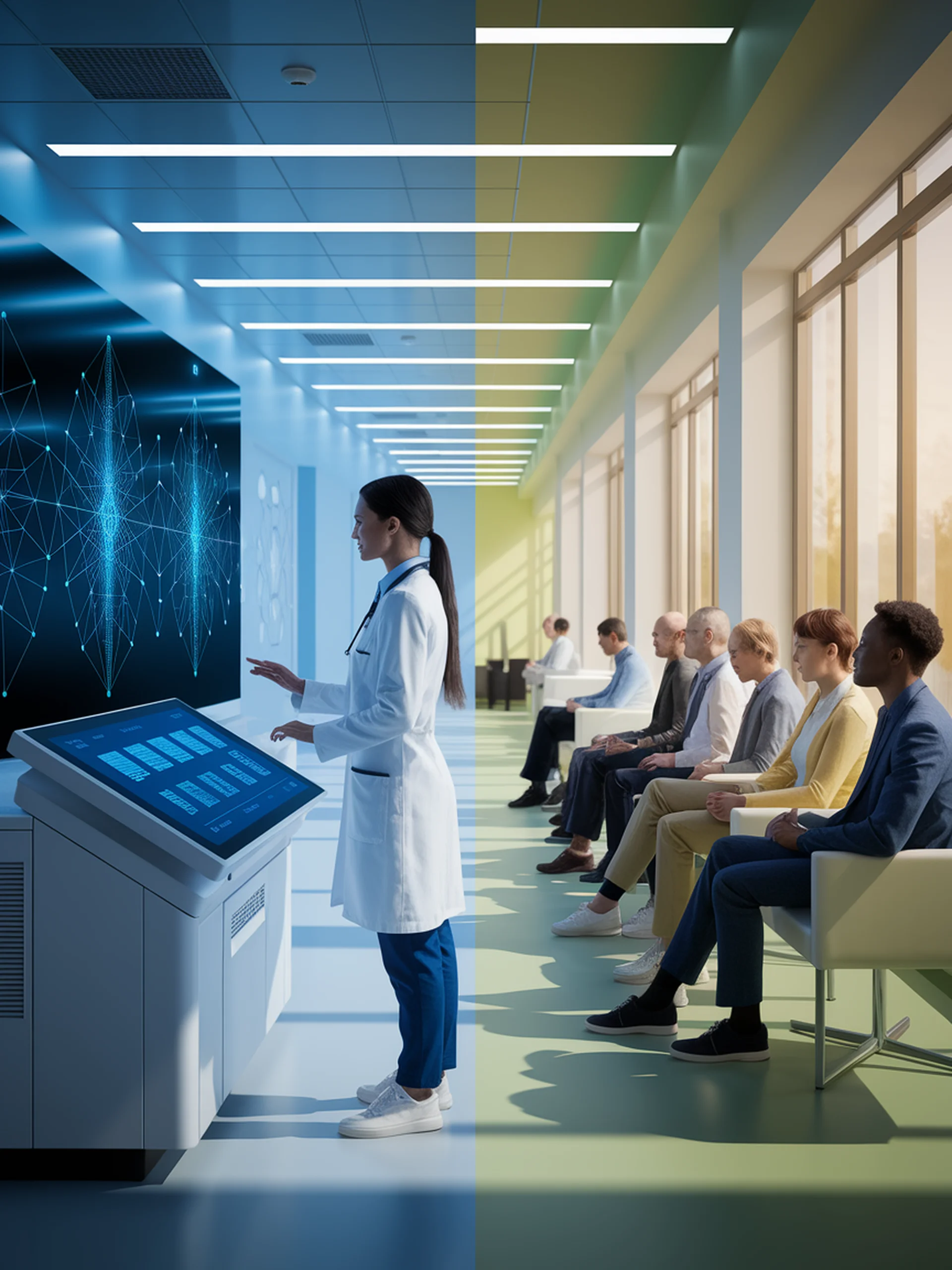
Debiasing LLMs for Fair Decision-Making
A Causality-Guided Approach to Mitigate Social Biases
This research introduces a framework to reduce discriminatory responses in large language models by identifying and removing causal relationships between social information and model decisions.
Key Findings:
- Leverages causal inference to identify and mitigate biases in LLM responses
- Targets critical applications in high-stakes decision-making including hiring and healthcare
- Reduces objectionable dependencies between social information inputs and model outputs
- Demonstrates a novel approach to fairness that can be implemented through effective prompting
Security Implications:
As LLMs increasingly influence important decisions, this framework addresses a critical security concern by preventing discriminatory outputs that could lead to unfair treatment or legal liability in sensitive domains.
Prompting Fairness: Integrating Causality to Debias Large Language Models