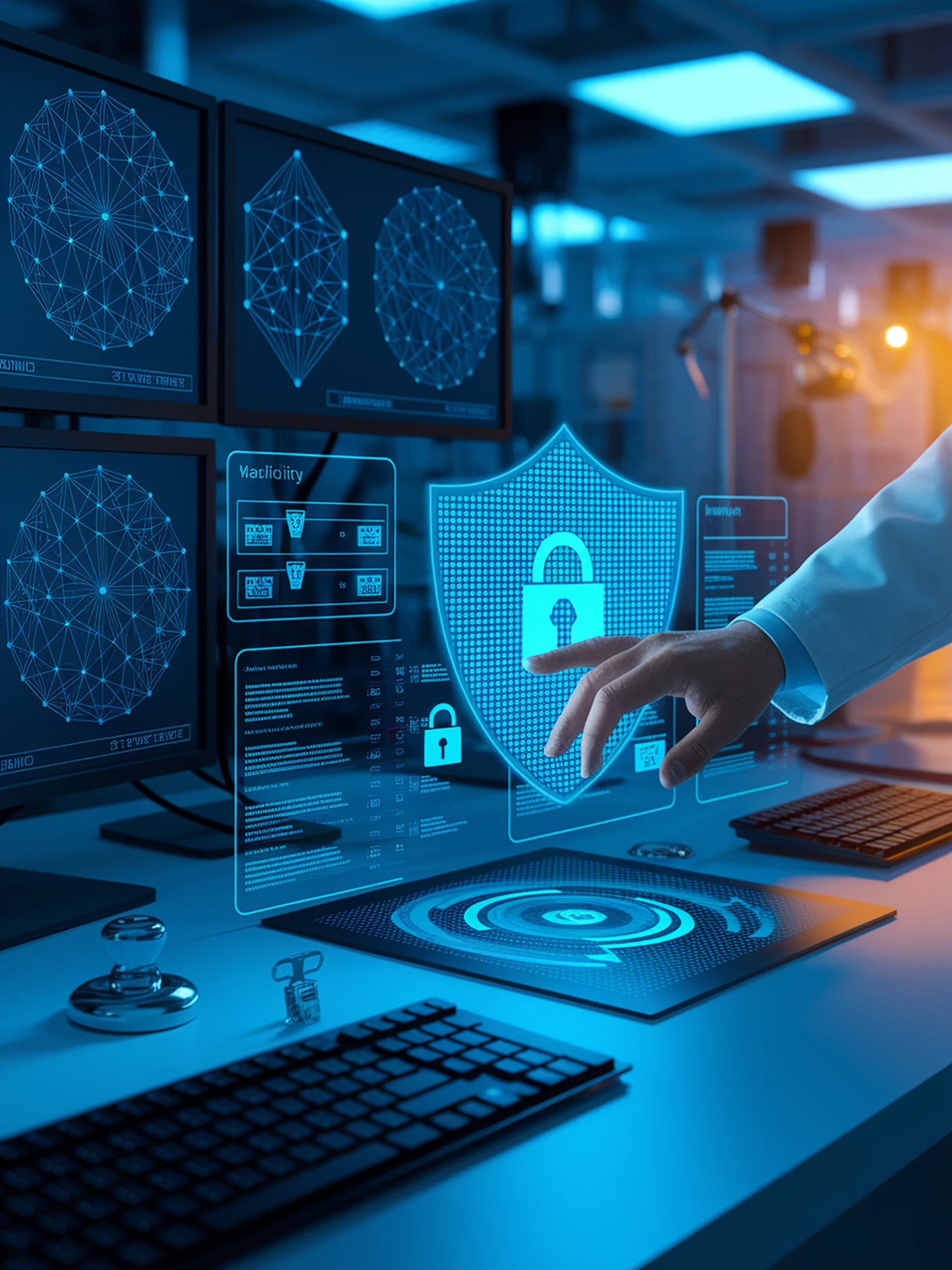
Benchmarking LLM Safety Refusal
A systematic approach to evaluating how LLMs reject unsafe requests
SORRY-Bench introduces a comprehensive benchmark for systematically evaluating how large language models recognize and refuse potentially harmful requests.
- Addresses limitations in existing evaluation methods by using fine-grained taxonomies of unsafe topics
- Provides balanced representation across 44 potentially unsafe topics
- Enables consistent assessment of LLMs' safety refusal capabilities
- Supports more secure and policy-compliant AI deployments
Why it matters: As LLMs become more integrated into sensitive environments, systematically evaluating their ability to refuse harmful requests is critical for maintaining security standards and building trustworthy AI systems.
SORRY-Bench: Systematically Evaluating Large Language Model Safety Refusal