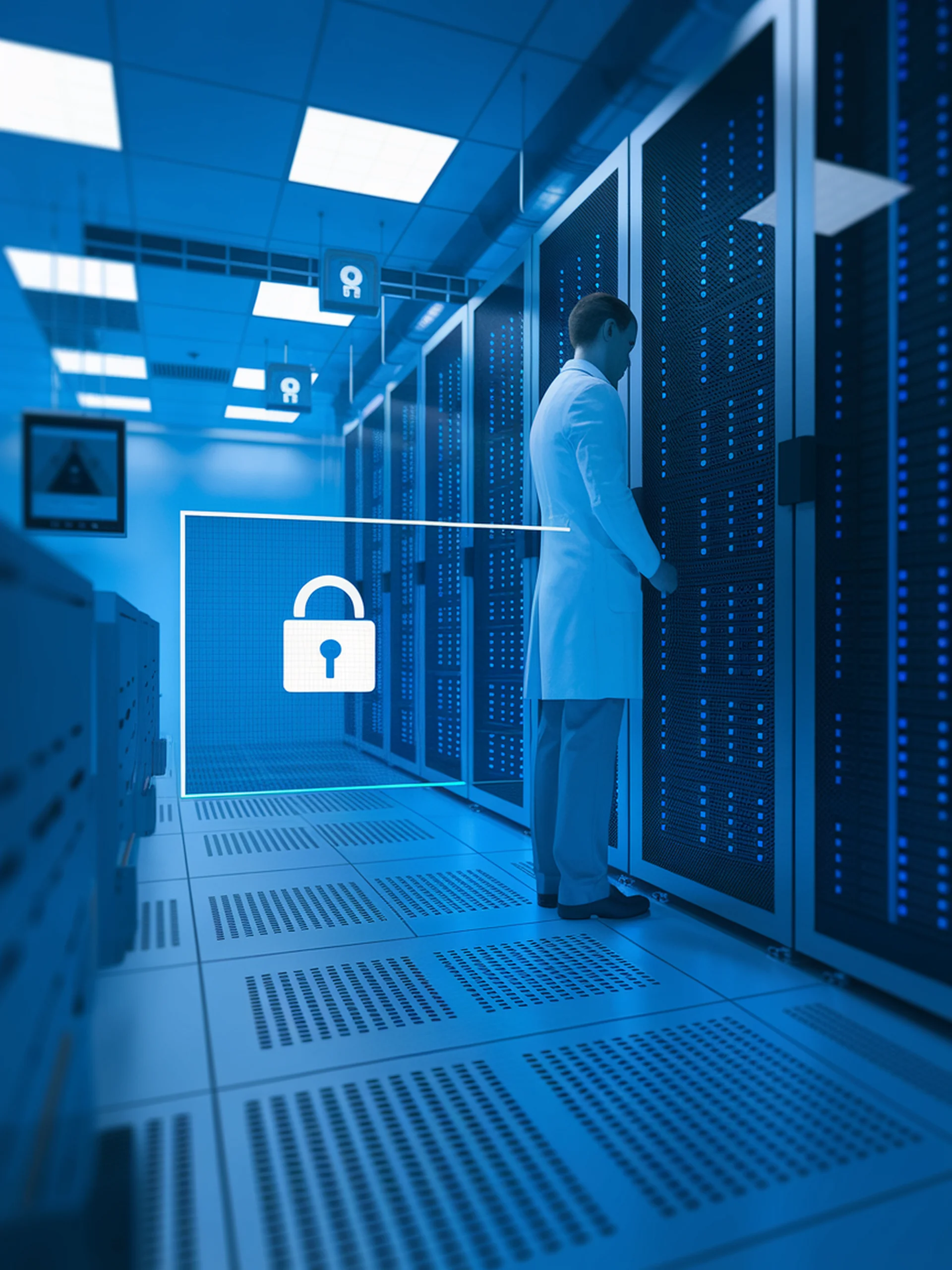
Protecting Privacy in the Age of LLMs
Critical threats and practical safeguards for sensitive data
This comprehensive survey maps the growing privacy challenges posed by Large Language Models and evaluates current mitigation strategies.
Key insights:
- Privacy vulnerabilities are particularly concerning in critical domains like healthcare where data sensitivity is heightened
- The research identifies multiple privacy attack vectors against LLMs and their training data
- Effective countermeasures include anonymization techniques, differential privacy, and machine unlearning
- Organizations deploying LLMs must implement appropriate privacy-preserving frameworks based on their specific use cases
For security professionals, this research provides essential guidance for balancing LLM utility with robust privacy protections when handling sensitive information.
Preserving Privacy in Large Language Models: A Survey on Current Threats and Solutions