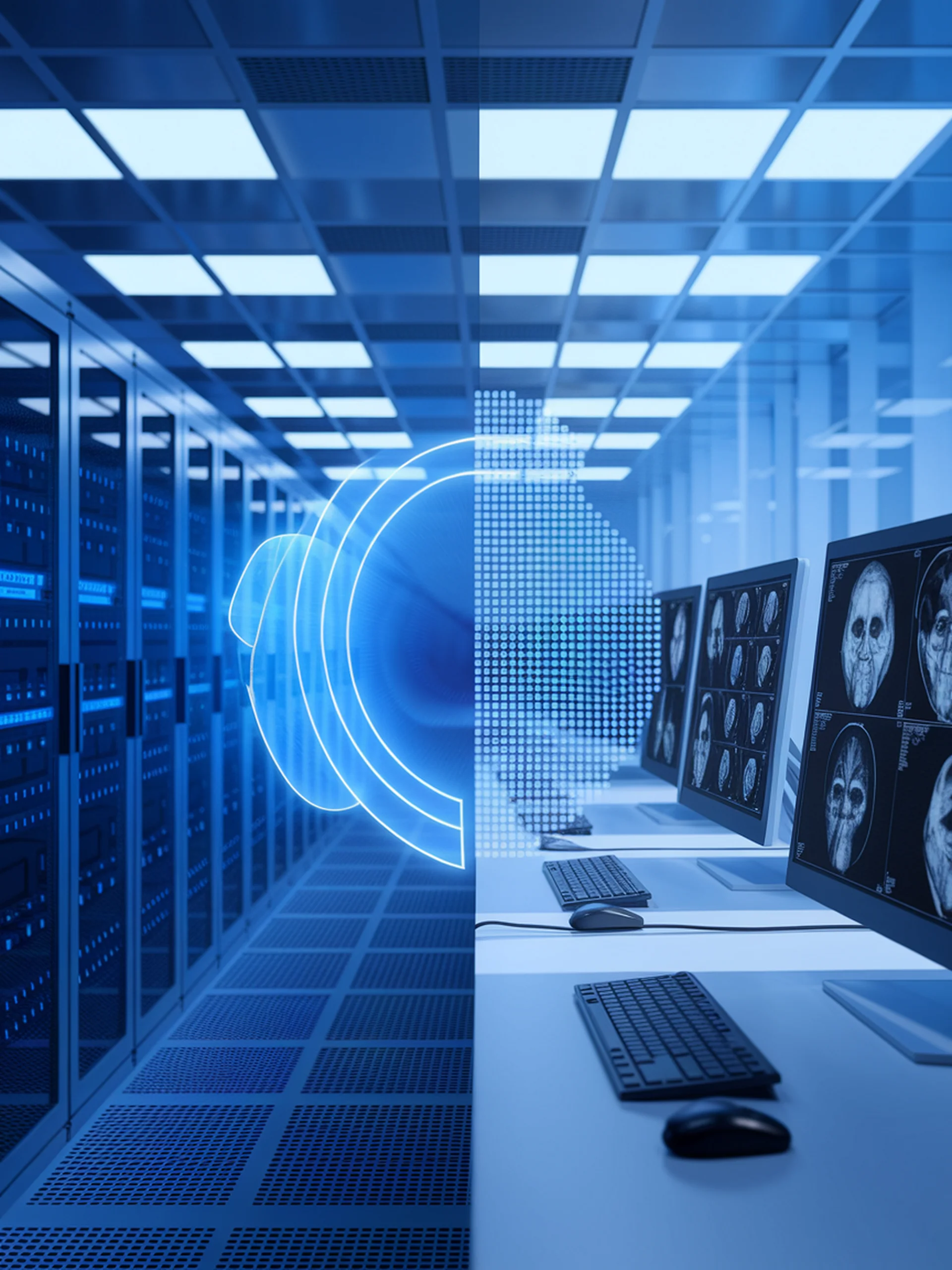
Balancing Privacy and Data Efficiency
A Novel Framework for Privacy-Preserving Active Learning
This research introduces a framework that reconciles the tension between active learning for efficient data selection and differential privacy for protecting sensitive information.
- Develops the first comprehensive approach to implement active learning while maintaining formal privacy guarantees
- Introduces Private Uncertainty Sampling that achieves up to 90% of non-private active learning performance
- Proposes Private Query-by-Committee that balances data utility and privacy protection
- Provides theoretical privacy bounds and practical implementation guidelines
Critical for organizations handling sensitive data that need to maximize learning efficiency while ensuring regulatory compliance and protecting individual privacy.
Differentially Private Active Learning: Balancing Effective Data Selection and Privacy