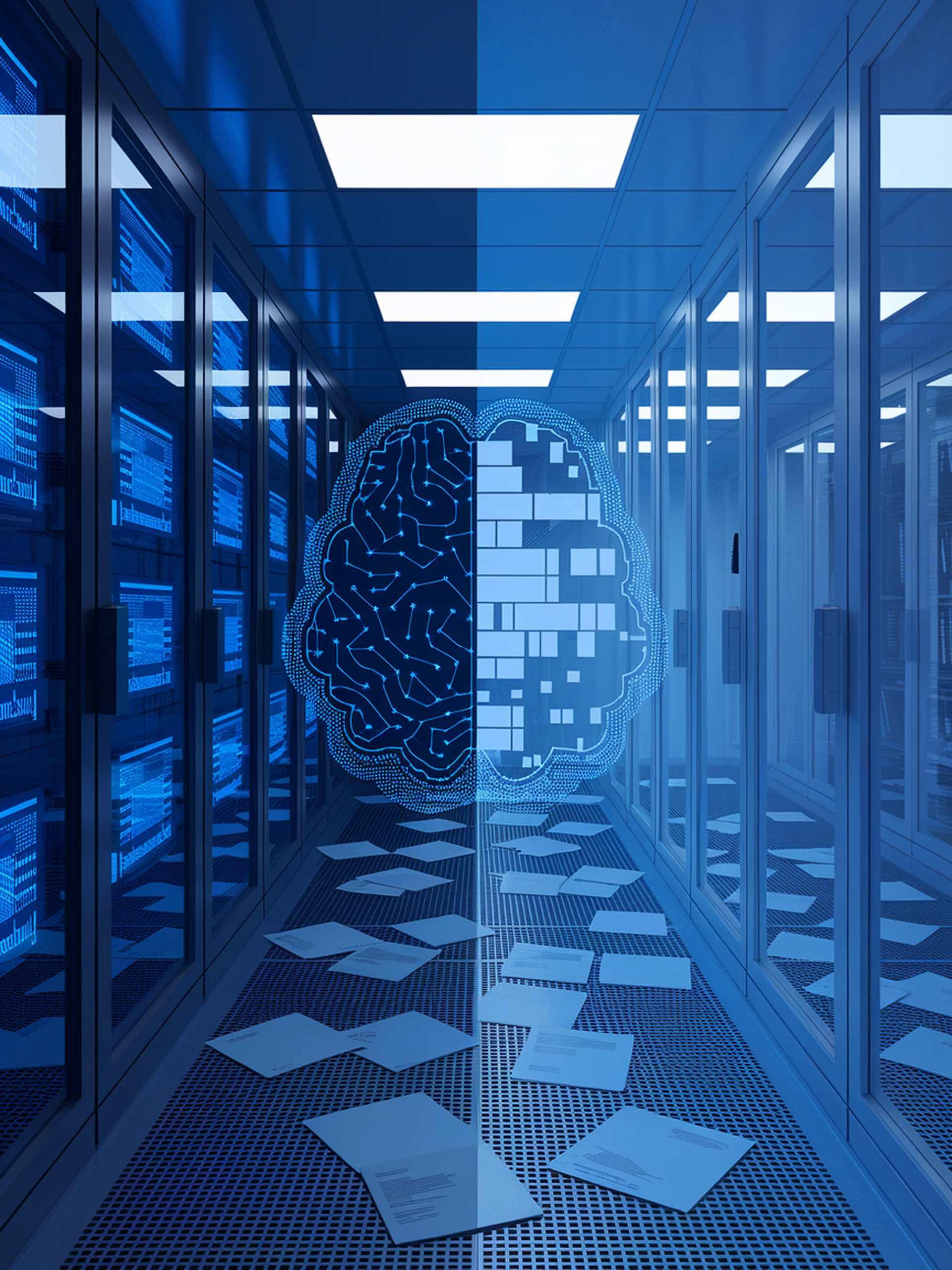
The Illusion of LLM Unlearning Progress
Why current benchmarks fail to measure true unlearning effectiveness
This research reveals critical weaknesses in existing LLM unlearning evaluation methods that create a false sense of progress in removing sensitive information from models.
- Current benchmarks provide overly optimistic and potentially misleading assessments of unlearning methods
- Simple modifications to evaluation approaches expose significant gaps in purported unlearning effectiveness
- Reliable unlearning is essential for security and privacy, particularly when models contain harmful or sensitive information
- The paper calls for more robust evaluation frameworks to accurately measure unlearning progress
This matters for security professionals as it highlights the need for caution when implementing unlearning methods to protect sensitive data and prevent harmful outputs in deployed LLM systems.
Position: LLM Unlearning Benchmarks are Weak Measures of Progress